Phonotactic Language Recognition Using A Universal Phoneme Recognizer and A Transformer Architecture.
IEEE International Conference on Acoustics, Speech, and Signal Processing (ICASSP)(2022)
摘要
In this paper, we describe a phonotactic language recognition model that effectively manages long and short n-gram input sequences to learn contextual phonotactic-based vector embeddings. Our approach uses a transformer-based encoder that integrates a sliding window attention to attempt finding discriminative short and long cooccurrences of language dependent n-gram phonetic units. We then evaluate and compare the use of different phoneme recognizers (Brno and Allosaurus) and sub-unit tokenizers to help select the more discriminative n-grams. The proposed architecture is evaluated using the Kalaka-3 database that contains clean and noisy audio recordings for very similar languages (i.e. Iberian languages, e.g., Spanish, Galician, Catalan). We provide results using the Cavg and accuracy metrics used in NIST evaluations. The experimental results show that our proposed approach outperforms by 21% of relative improvement to the best system presented in the Albayzin LR competition.
更多查看译文
关键词
Language recognition,phonotactic information,transformers,acoustic systems
AI 理解论文
溯源树
样例
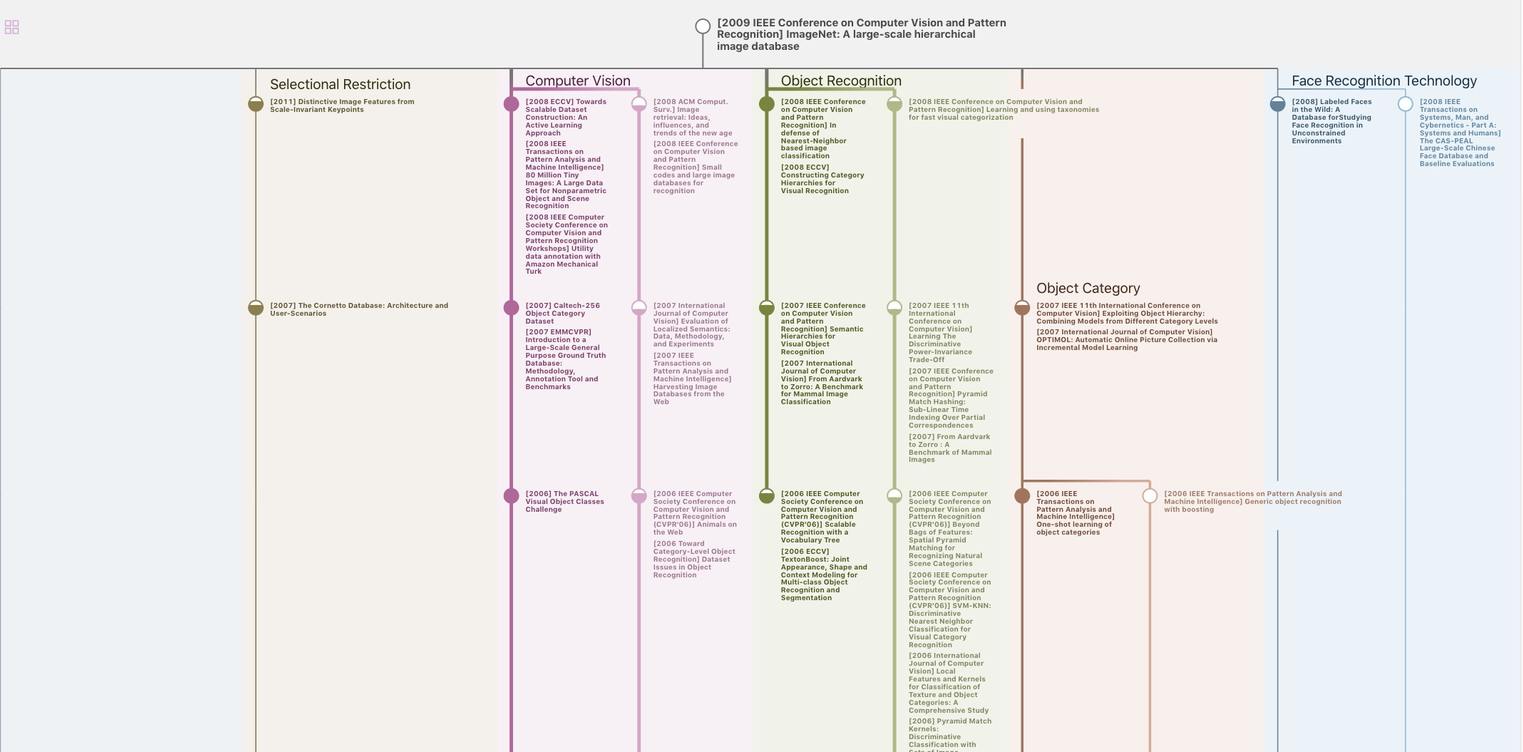
生成溯源树,研究论文发展脉络
Chat Paper
正在生成论文摘要