Text Adaptive Detection for Customizable Keyword Spotting.
IEEE International Conference on Acoustics, Speech, and Signal Processing (ICASSP)(2022)
摘要
Always-on keyword spotting (KWS), i.e., wake word detection, has been widely used in many voice assistant applications running on smart devices. Although fixed wakeup word detection trained on specifically collected data has reached high performance, it is still challenging to build an arbitrarily customizable detection system on general found data. A deep learning classifier, similar to the one in speech recognition, can be used, but the detection performance is usually significantly degraded. In this work, we propose a novel text adaptive detection framework to directly formulate KWS as a detection rather than a classification problem. Here, the text prompt is used as input to promote biased classification, and a series of frame and sequence level detection criteria are employed to replace the cross-entropy criterion and directly optimize detection performance. Experiments on a keyword spotting version of Wall Street Journal (WSJ) dataset show that the text adaptive detection framework can achieve an average relative improvement of 16.88% in the detection metric Fl -score compared to the baseline model.
更多查看译文
关键词
streaming,wake word detection,arbitrary wake word,text prompt,training detection criteria
AI 理解论文
溯源树
样例
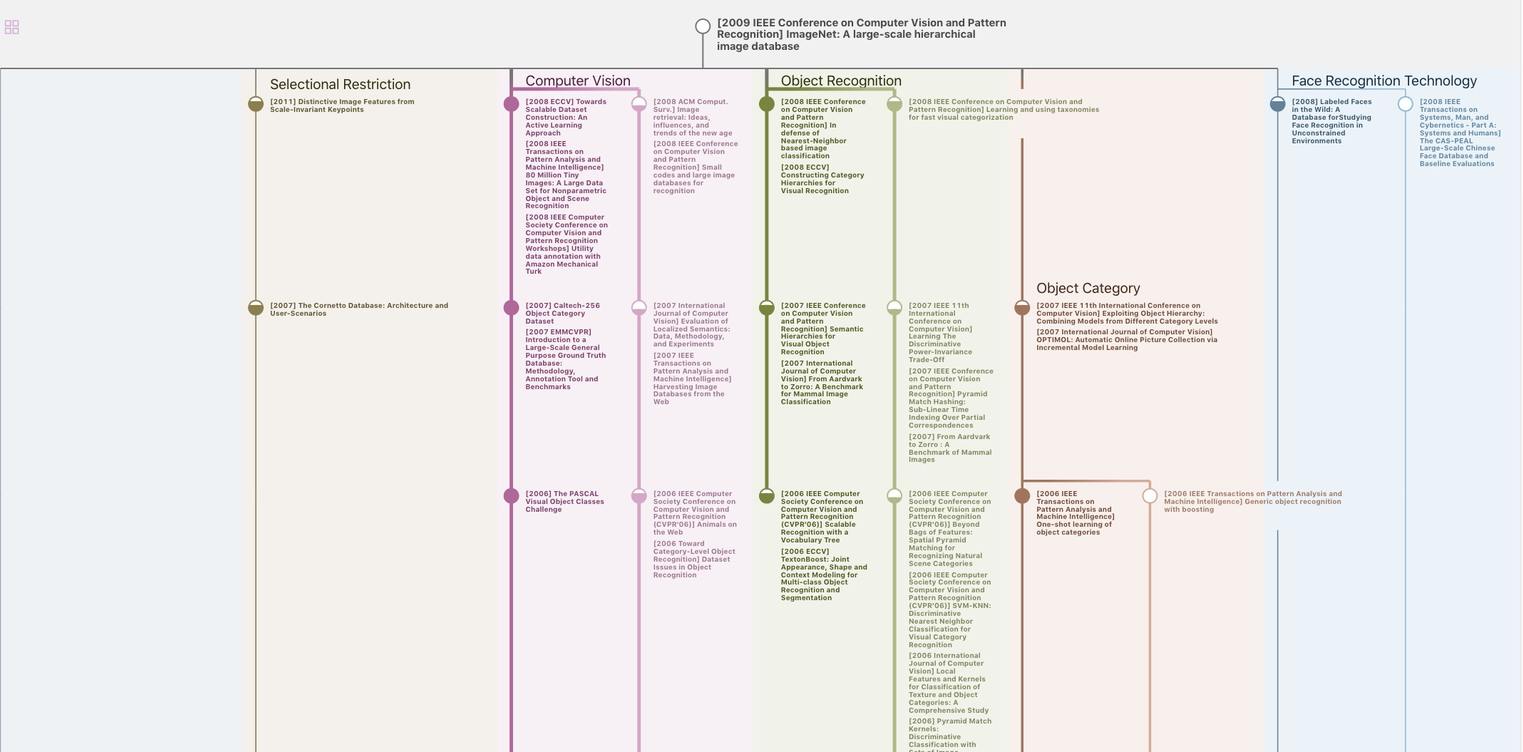
生成溯源树,研究论文发展脉络
Chat Paper
正在生成论文摘要