A Simple Graph Neural Network via Layer Sniffer.
IEEE International Conference on Acoustics, Speech, and Signal Processing (ICASSP)(2022)
摘要
Due to the success of Graph Neural Networks(GNNs) in graph-structure data, many efforts have been devoted to enhancing the propagation ability and alleviating the over-smoothing problem of GNNs. However, from the perspective of closeness extent of node representations, most existing GNNs pay less attention to the attributes of node representation space. In light of this, we design a Layer Sniffer module that can combine the effects of the local node-level representation closeness extent and the global layer-level information attention. On this basis, we propose a simple Layer Sniffer Graph Neural Network (LSGNN) with a propagation scheme that can fuse neighborhood information of different receptive fields densely and adaptively. Our extensive experiments on three public node classification datasets demonstrate the superior performance and stability of our proposed model.
更多查看译文
关键词
Graph Learning,Semi-supervised Learning,Graph Neural Networks,Graph Signal Processing
AI 理解论文
溯源树
样例
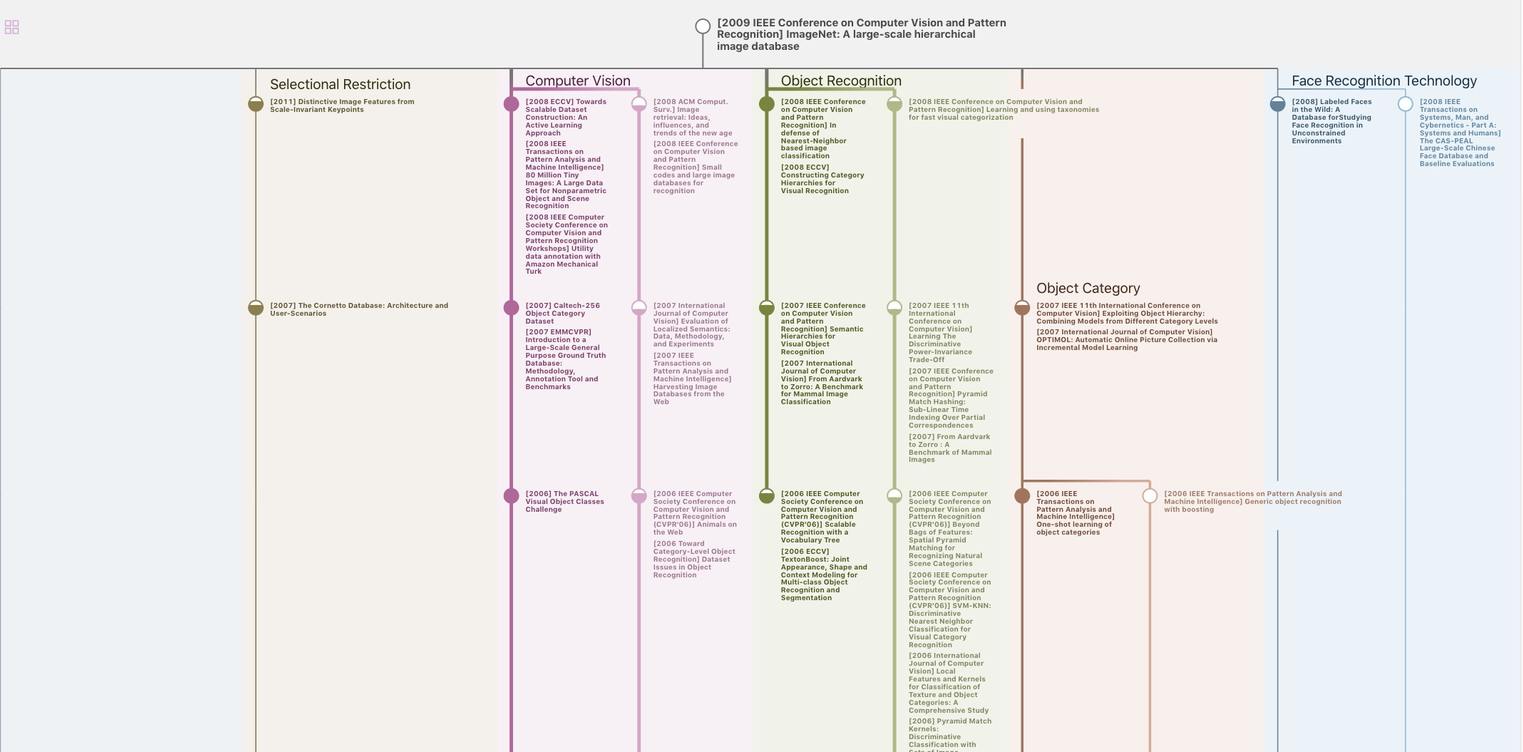
生成溯源树,研究论文发展脉络
Chat Paper
正在生成论文摘要