Wassertrain: An Adversarial Training Framework Against Wasserstein Adversarial Attacks.
IEEE International Conference on Acoustics, Speech, and Signal Processing (ICASSP)(2022)
摘要
This paper presents an adversarial training framework WasserTrain for improving model robustness against the adversarial attacks in terms of the Wasserstein distance. First, an effective attack method WasserAttack is introduced with a novel encoding of the optimization problem, which directly finds the worst point within the Wasserstein ball while keeping the relaxation error of the Wasserstein transformation as small as possible. The proposed adversarial training framework utilizes these high-quality adversarial examples to train robust models. Experiments on MNIST show that the adversarial loss arising from adversarial examples found by our method is about three times as much as that found by the PGD-based attack method. Furthermore, within the Wasserstein ball with a radius of 0.5, the WasserTrain model achieves 31% adversarial robustness against WasserAttack, which is 22% higher than that on the PGD-based training model.
更多查看译文
关键词
Wasserstein distance,adversarial attack,adversarial training
AI 理解论文
溯源树
样例
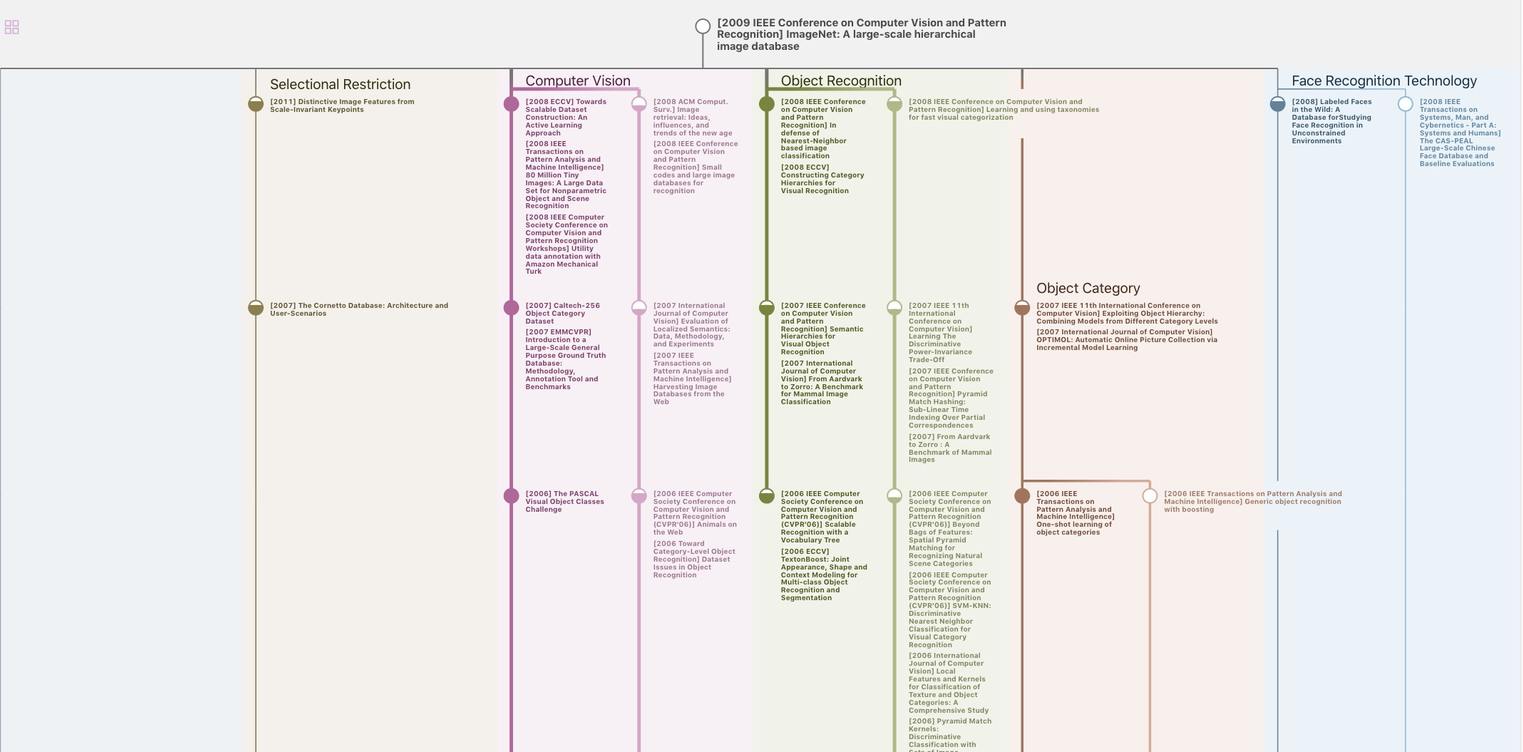
生成溯源树,研究论文发展脉络
Chat Paper
正在生成论文摘要