Improving Class Activation Map for Weakly Supervised Object Localization.
IEEE International Conference on Acoustics, Speech, and Signal Processing (ICASSP)(2022)
摘要
We propose a Weakly Supervised Object Localization (WSOL) method that can locate an object within a given image using a pre-trained network learned with only class labels without location annotations. Most existing WSOL methods rely on thresholding a Class Activation Map (CAM) generated by the pre-trained network to highlight and localize the object. However such approaches often produce incomplete object bounding boxes, as only the discriminative parts of the object are selected during thresholding. We revisit current CAM-based WSOL approaches and propose a pipeline to: (1) refine the CAM map using Weighted Global Average Pooling (WGAP), (2) recombine weights to make use of the negative features, (3) adaptively select a suitable threshold to achieve better object localization. Our method does not require additional learning or hyperparameter tuning. We show that our simple approach can achieve competitive results when evaluated on the CUB-200-2011 and ILSVRC 2016 datasets against other state-of-the-art methods.
更多查看译文
关键词
weakly supervised object localization,class activation map,weighted global average pooling
AI 理解论文
溯源树
样例
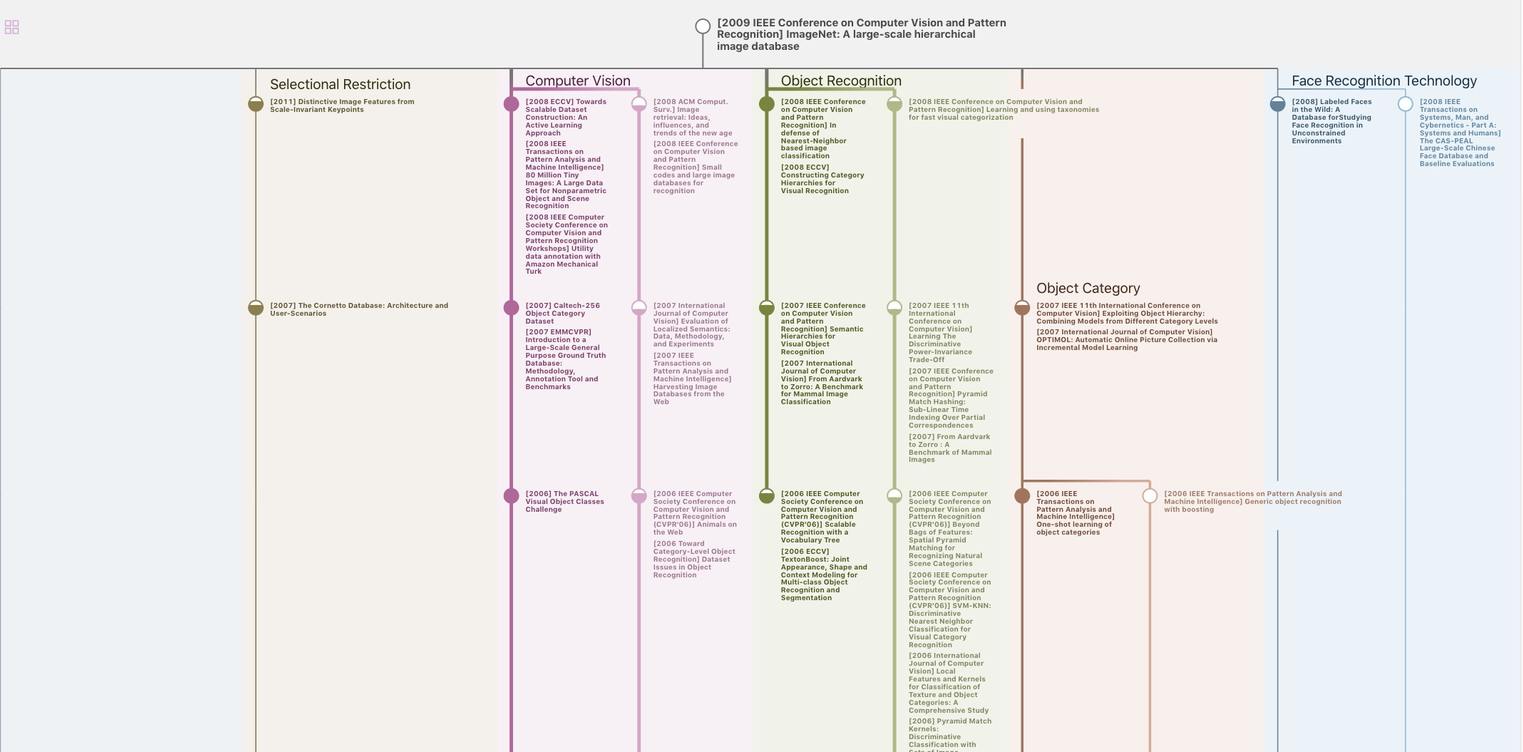
生成溯源树,研究论文发展脉络
Chat Paper
正在生成论文摘要