Invisible and Efficient Backdoor Attacks for Compressed Deep Neural Networks
ICASSP 2022 - 2022 IEEE International Conference on Acoustics, Speech and Signal Processing (ICASSP)(2022)
摘要
Compressed deep neural network (DNN) models have been widely deployed in many resource-constrained platforms and devices. However, the security issue of the compressed models, especially their vulnerability against backdoor attacks, is not well explored yet. In this paper, we study the feasibility of practical backdoor attacks for the compressed DNNs. More specifically, we propose a universal adversarial perturbation (UAP)-based approach to achieve both high attack stealthiness and high attack efficiency simultaneously. Evaluation results across different DNN models and datasets with various compression ratios demonstrate our approach’s superior performance compared with the existing solutions.
更多查看译文
关键词
Backdoor attack,deep neural network,compression
AI 理解论文
溯源树
样例
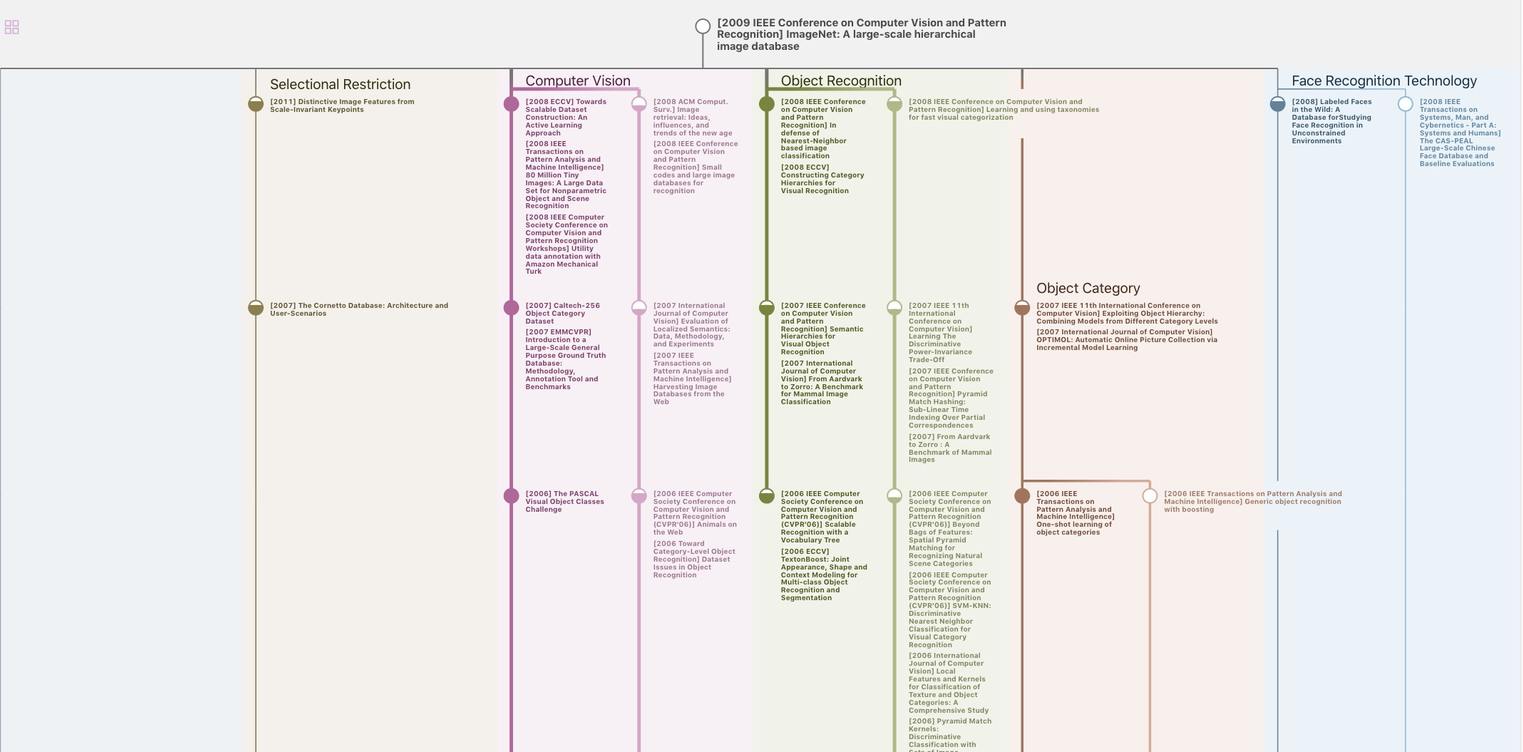
生成溯源树,研究论文发展脉络
Chat Paper
正在生成论文摘要