Learning versus Refutation in Noninteractive Local Differential Privacy
arxiv(2022)
摘要
We study two basic statistical tasks in non-interactive local differential privacy (LDP): learning and refutation. Learning requires finding a concept that best fits an unknown target function (from labelled samples drawn from a distribution), whereas refutation requires distinguishing between data distributions that are well-correlated with some concept in the class, versus distributions where the labels are random. Our main result is a complete characterization of the sample complexity of agnostic PAC learning for non-interactive LDP protocols. We show that the optimal sample complexity for any concept class is captured by the approximate $\gamma_2$~norm of a natural matrix associated with the class. Combined with previous work [Edmonds, Nikolov and Ullman, 2019] this gives an equivalence between learning and refutation in the agnostic setting.
更多查看译文
关键词
local,learning,refutation
AI 理解论文
溯源树
样例
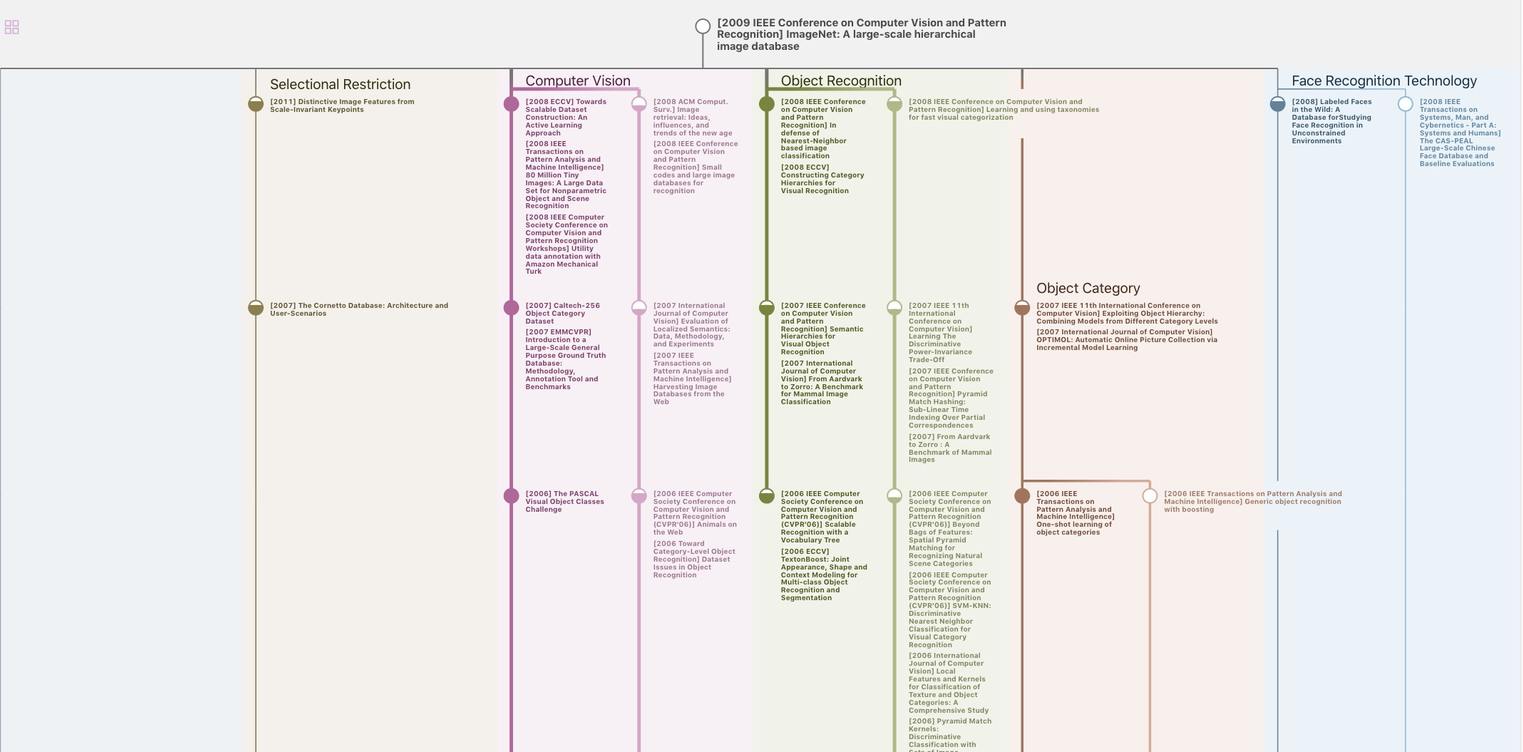
生成溯源树,研究论文发展脉络
Chat Paper
正在生成论文摘要