Toroidal Probabilistic Spherical Discriminant Analysis
arxiv(2022)
摘要
In speaker recognition, where speech segments are mapped to embeddings on the unit hypersphere, two scoring back-ends are commonly used, namely cosine scoring and PLDA. We have recently proposed PSDA, an analog to PLDA that uses Von Mises-Fisher distributions instead of Gaussians. In this paper, we present toroidal PSDA (T-PSDA). It extends PSDA with the ability to model within and between-speaker variabilities in toroidal submanifolds of the hypersphere. Like PLDA and PSDA, the model allows closed-form scoring and closed-form EM updates for training. On VoxCeleb, we find T-PSDA accuracy on par with cosine scoring, while PLDA accuracy is inferior. On NIST SRE'21 we find that T-PSDA gives large accuracy gains compared to both cosine scoring and PLDA.
更多查看译文
关键词
speaker recognition,PSDA,Von Mises-Fisher
AI 理解论文
溯源树
样例
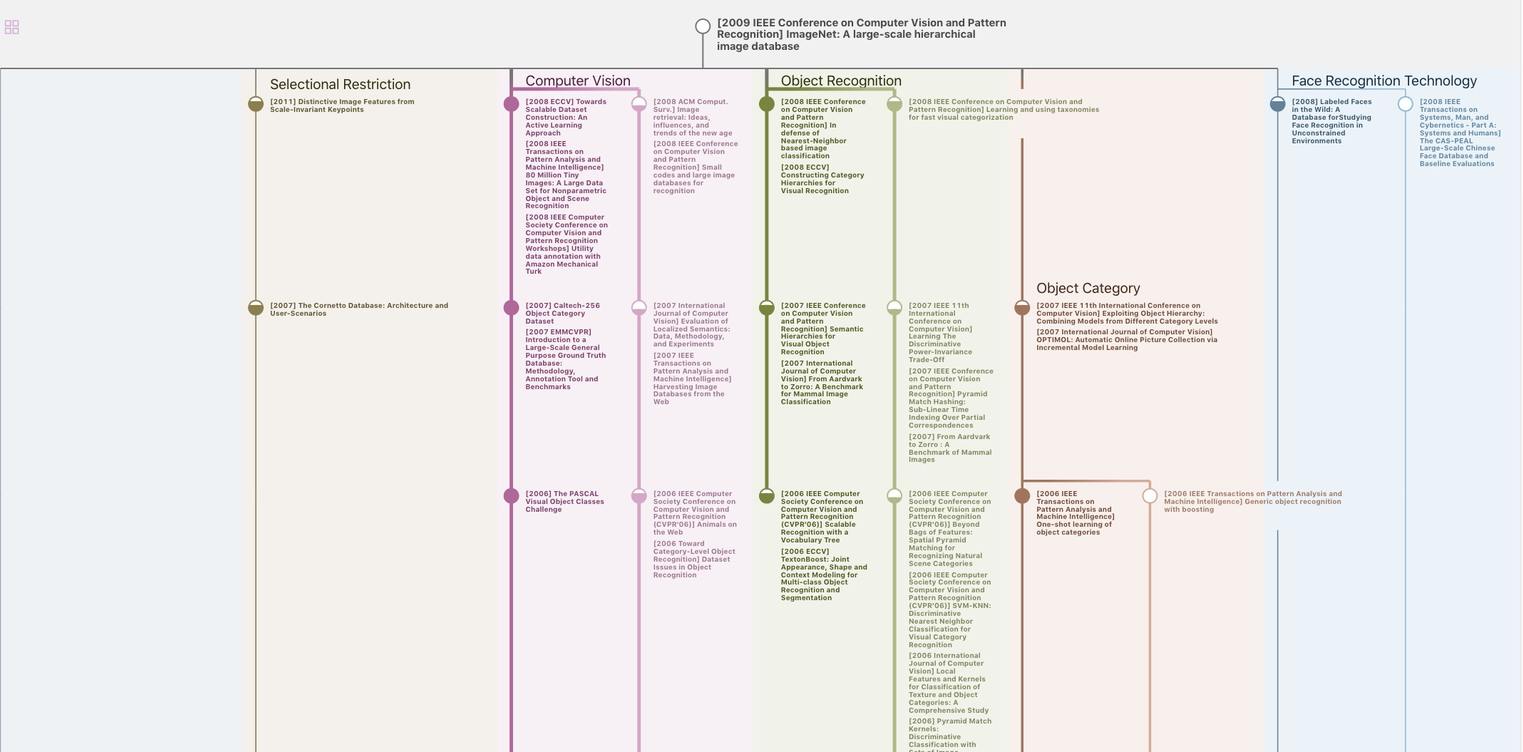
生成溯源树,研究论文发展脉络
Chat Paper
正在生成论文摘要