CasNet: Investigating Channel Robustness for Speech Separation
arxiv(2022)
摘要
Recording channel mismatch between training and testing conditions has been shown to be a serious problem for speech separation. This situation greatly reduces the separation performance, and cannot meet the requirement of daily use. In this study, inheriting the use of our previously constructed TAT-2mix corpus, we address the channel mismatch problem by proposing a channel-aware audio separation network (CasNet), a deep learning framework for end-to-end time-domain speech separation. CasNet is implemented on top of TasNet. Channel embedding (characterizing channel information in a mixture of multiple utterances) generated by Channel Encoder is introduced into the separation module by the FiLM technique. Through two training strategies, we explore two roles that channel embedding may play: 1) a real-life noise disturbance, making the model more robust, or 2) a guide, instructing the separation model to retain the desired channel information. Experimental results on TAT-2mix show that CasNet trained with both training strategies outperforms the TasNet baseline, which does not use channel embeddings.
更多查看译文
关键词
speech separation,channel robustness
AI 理解论文
溯源树
样例
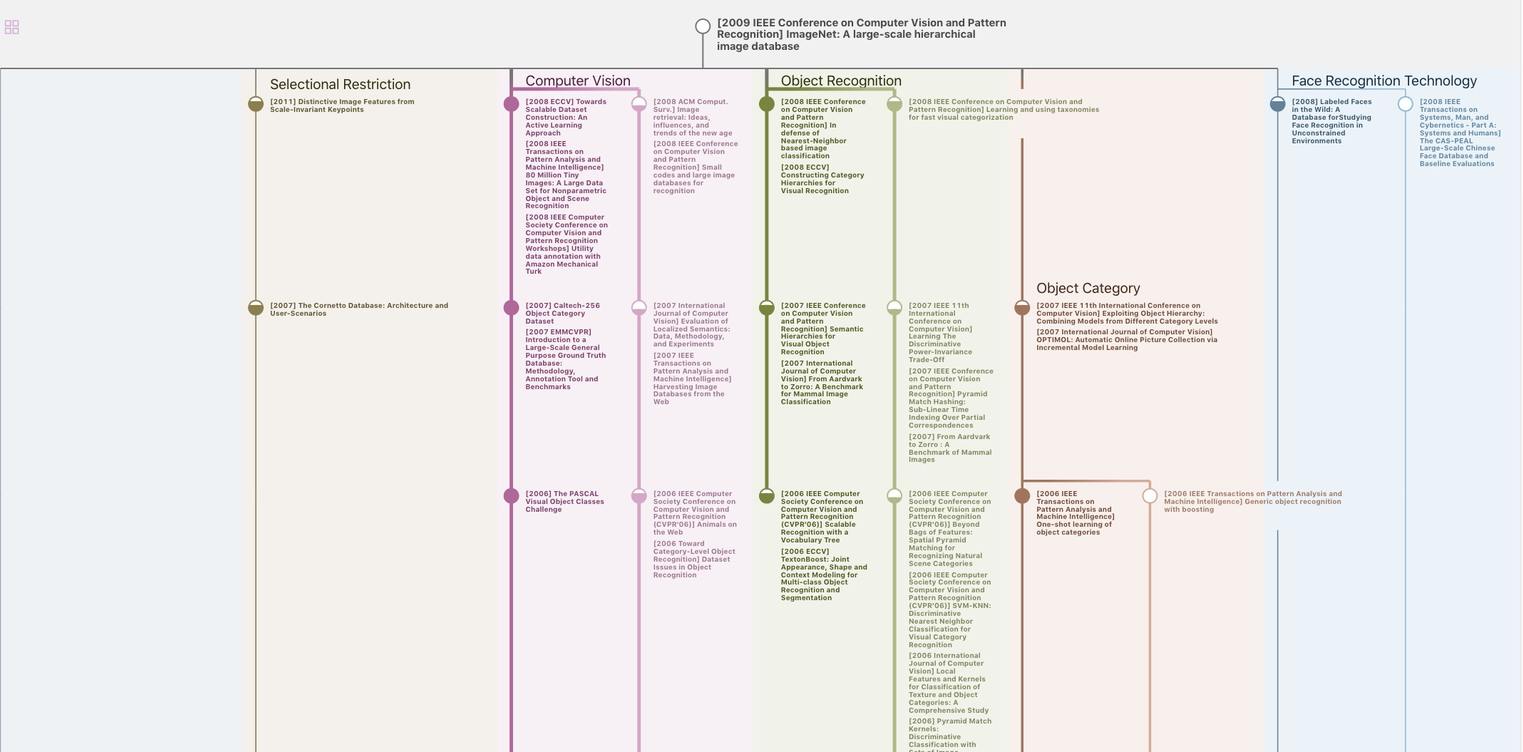
生成溯源树,研究论文发展脉络
Chat Paper
正在生成论文摘要