Gendered Mental Health Stigma in Masked Language Models
emnlp 2022(2022)
摘要
Mental health stigma prevents many individuals from receiving the appropriate care, and social psychology studies have shown that mental health tends to be overlooked in men. In this work, we investigate gendered mental health stigma in masked language models. In doing so, we operationalize mental health stigma by developing a framework grounded in psychology research: we use clinical psychology literature to curate prompts, then evaluate the models' propensity to generate gendered words. We find that masked language models capture societal stigma about gender in mental health: models are consistently more likely to predict female subjects than male in sentences about having a mental health condition (32% vs. 19%), and this disparity is exacerbated for sentences that indicate treatment-seeking behavior. Furthermore, we find that different models capture dimensions of stigma differently for men and women, associating stereotypes like anger, blame, and pity more with women with mental health conditions than with men. In showing the complex nuances of models' gendered mental health stigma, we demonstrate that context and overlapping dimensions of identity are important considerations when assessing computational models' social biases.
更多查看译文
关键词
masked language models,mental health
AI 理解论文
溯源树
样例
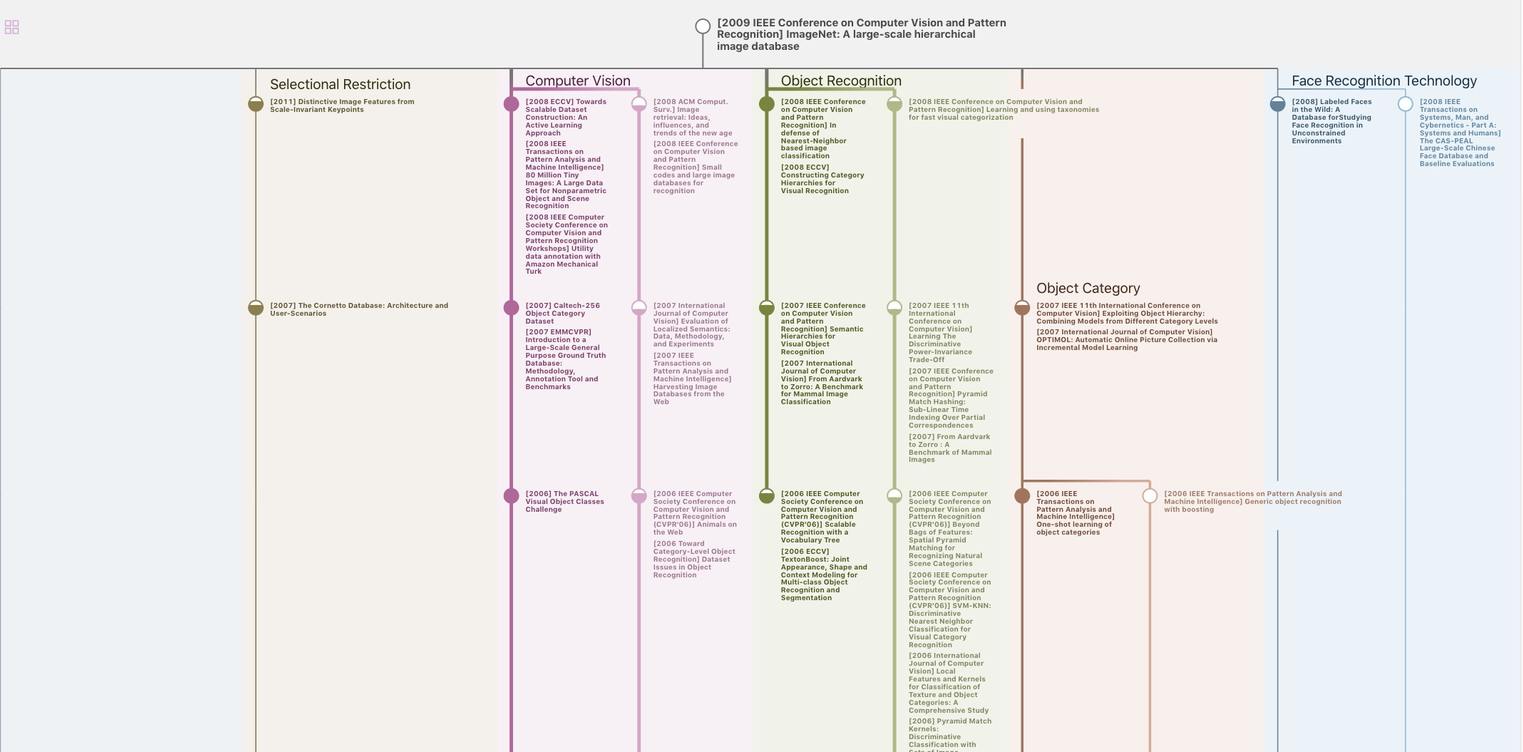
生成溯源树,研究论文发展脉络
Chat Paper
正在生成论文摘要