Towards customizable reinforcement learning agents: Enabling preference specification through online vocabulary expansion
arxiv(2023)
摘要
There is a growing interest in developing automated agents that can work alongside humans. In addition to completing the assigned task, such an agent will undoubtedly be expected to behave in a manner that is preferred by the human. This requires the human to communicate their preferences to the agent. To achieve this, the current approaches either require the users to specify the reward function or the preference is interactively learned from queries that ask the user to compare behavior. The former approach can be challenging if the internal representation used by the agent is inscrutable to the human while the latter is unnecessarily cumbersome for the user if their preference can be specified more easily in symbolic terms. In this work, we propose PRESCA (PREference Specification through Concept Acquisition), a system that allows users to specify their preferences in terms of concepts that they understand. PRESCA maintains a set of such concepts in a shared vocabulary. If the relevant concept is not in the shared vocabulary, then it is learned. To make learning a new concept more feedback efficient, PRESCA leverages causal associations between the target concept and concepts that are already known. In addition, we use a novel data augmentation approach to further reduce required feedback. We evaluate PRESCA by using it on a Minecraft environment and show that it can effectively align the agent with the user's preference.
更多查看译文
关键词
customizable reinforcement,preference
AI 理解论文
溯源树
样例
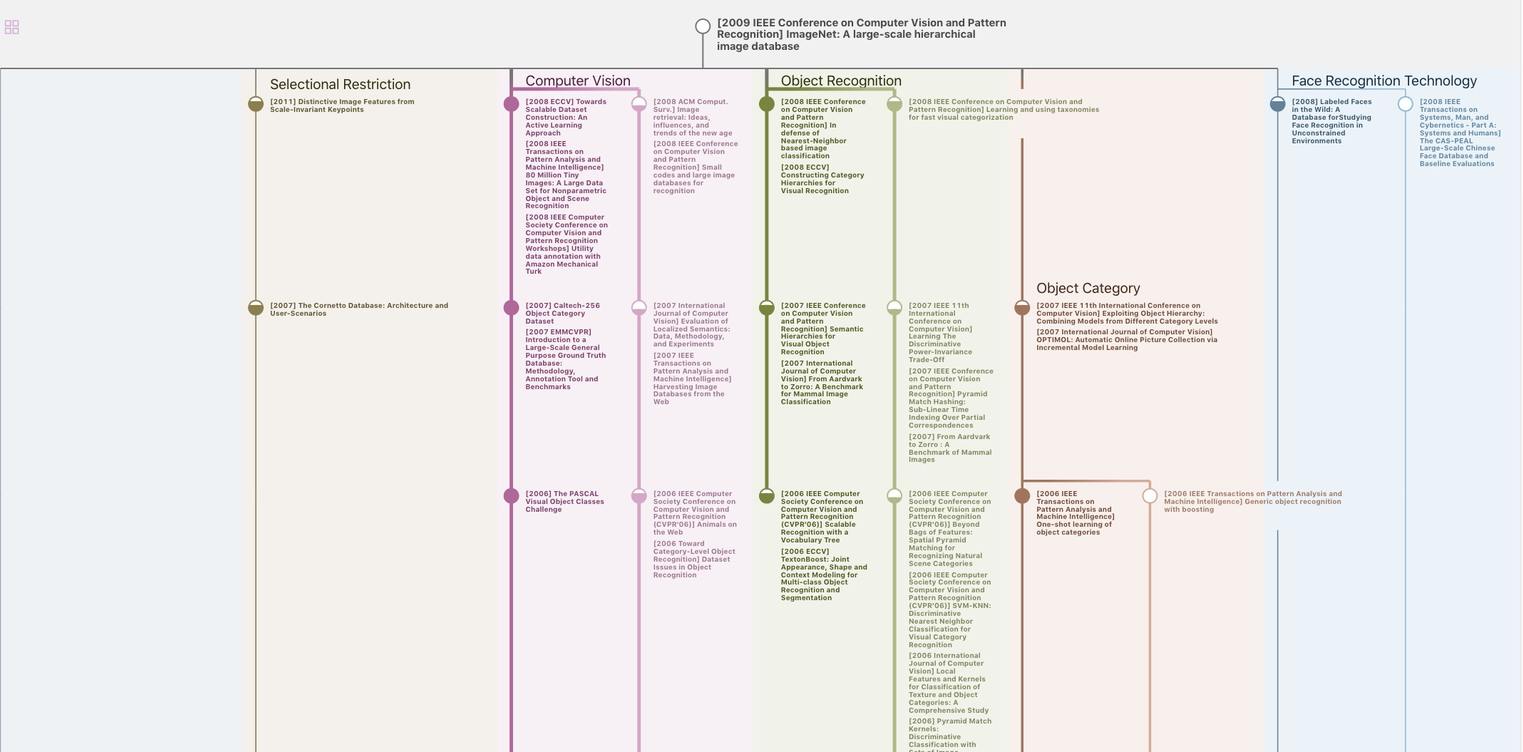
生成溯源树,研究论文发展脉络
Chat Paper
正在生成论文摘要