Learning coordinated emotion representation between voice and face
Applied Intelligence(2022)
摘要
Voice and face information are two most important perceptual modalities for human. In recent years, many researchers show great interest in learning cross-modal representations for different face-voice association tasks. However, these existing methods focus on the various biological attributions but rarely take emotion semantics between voice and face into account. In this paper, we present a novel two-stream model, called Emo tion R epresentation L earning Net work (EmoRL-Net), to learn the cross-modal coordinated emotion representations for various downstream matching and retrieval tasks. Within the proposed approach, we first propose two sub-network architectures that learn two unimodal features from the two modalities. Afterwards, we train EmoRL-Net by an objective loss function which includes one explicit and two implicit constraints. Meanwhile, an online semi-hard negative mining strategy is utilized to construct triplet units in a mini-batch manner, thereby stabilize and speeding up the learning process. Extensive experiments demonstrate that the proposed method can benefit various face-voice emotion tasks, including cross-modal verification, 1:2 matching, 1:N matching, and retrieval scenarios. The experiment results also show the proposed method outperforms the state-of-the-art approaches.
更多查看译文
关键词
Face-voice emotion relationship, Coordinated emotion representation, Cross-modal matching, Metric learning
AI 理解论文
溯源树
样例
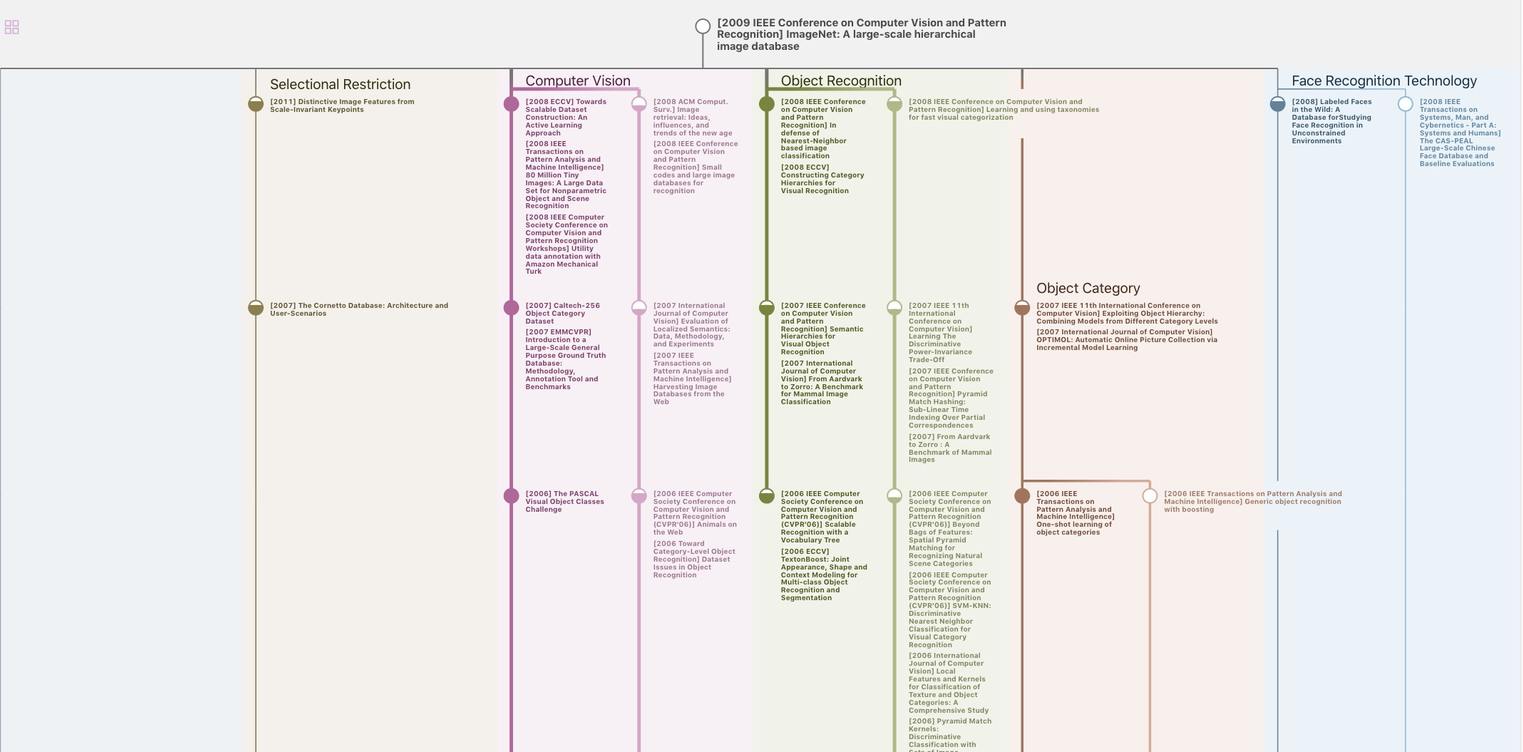
生成溯源树,研究论文发展脉络
Chat Paper
正在生成论文摘要