Inducer-tuning: Connecting Prefix-tuning and Adapter-tuning
emnlp 2022(2022)
摘要
Prefix-tuning, or more generally continuous prompt tuning, has become an essential paradigm of parameter-efficient transfer learning. Using a large pre-trained language model (PLM), prefix-tuning can obtain strong performance by training only a small portion of parameters. In this paper, we propose to understand and further develop prefix-tuning through the kernel lens. Specifically, we make an analogy between \textit{prefixes} and \textit{inducing variables} in kernel methods and hypothesize that \textit{prefixes} serving as \textit{inducing variables} would improve their overall mechanism. From the kernel estimator perspective, we suggest a new variant of prefix-tuning -- \textit{inducer-tuning}, which shares the exact mechanism as prefix-tuning while leveraging the residual form found in adapter-tuning. This mitigates the initialization issue in prefix-tuning. Through comprehensive empirical experiments on natural language understanding and generation tasks, we demonstrate that inducer-tuning can close the performance gap between prefix-tuning and fine-tuning.
更多查看译文
关键词
inducer-tuning,prefix-tuning,adapter-tuning
AI 理解论文
溯源树
样例
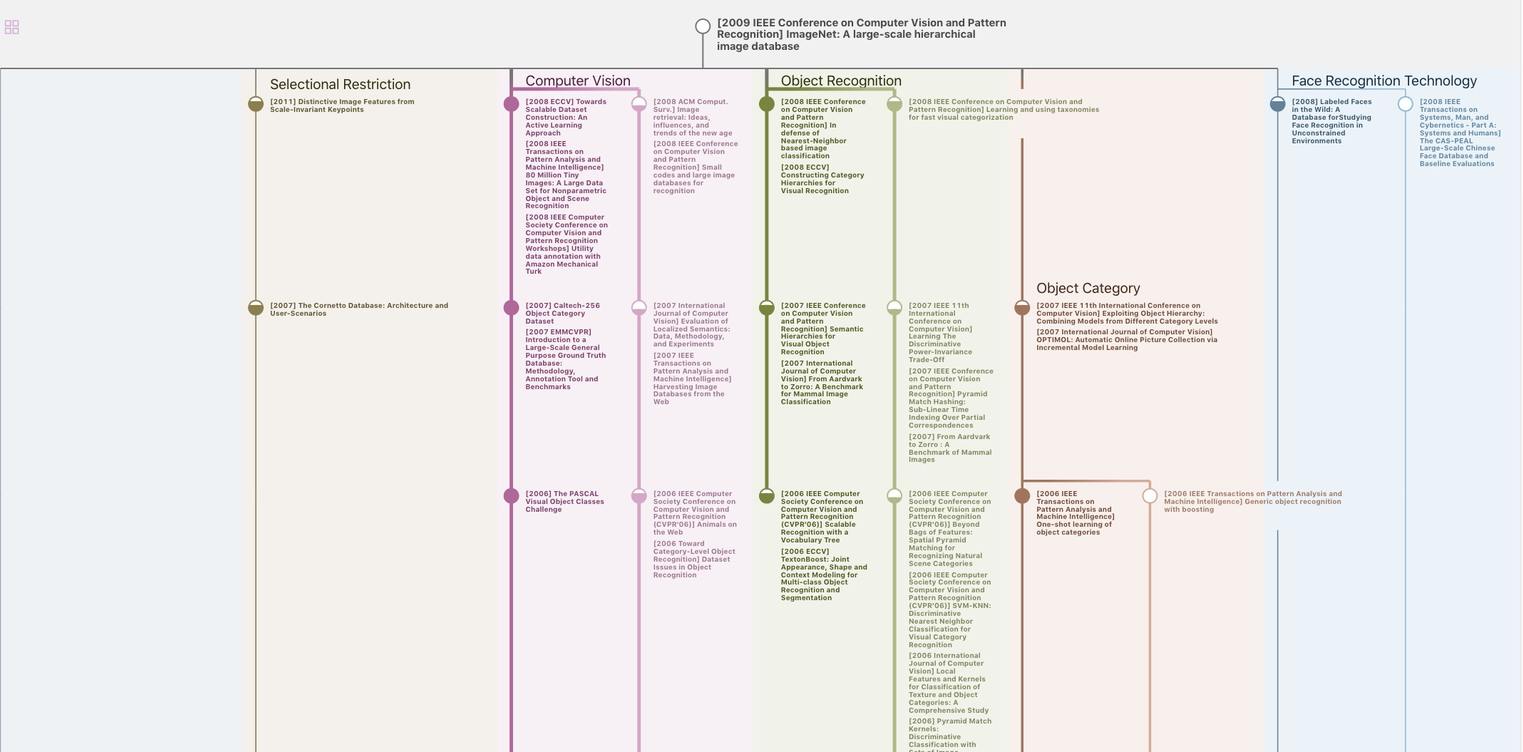
生成溯源树,研究论文发展脉络
Chat Paper
正在生成论文摘要