Spectral estimation of large stochastic blockmodels with discrete nodal covariates
Journal of Business & Economic Statistics(2022)
摘要
In many applications of network analysis, it is important to distinguish between observed and unobserved factors affecting network structure. We show that a network model with discrete unobserved link heterogeneity and binary (or discrete) covariates corresponds to a stochastic blockmodel (SBM). We develop a spectral estimator for the effect of covariates on link probabilities, exploiting the correspondence of SBMs and generalized random dot product graphs (GRDPG). We show that computing our estimator is much faster than standard variational expectation-maximization algorithms and scales well for large networks. Monte Carlo experiments suggest that the estimator performs well under different data generating processes. Our application to Facebook data shows evidence of homophily in gender, role and campus-residence, while allowing us to discover unobserved communities. Finally, we establish asymptotic normality of our estimators.
更多查看译文
关键词
Asymptotic normality,Conditionally independent links,Generalized random dot product graphs,Large networks,Spectral estimation,Stochastic blockmodel
AI 理解论文
溯源树
样例
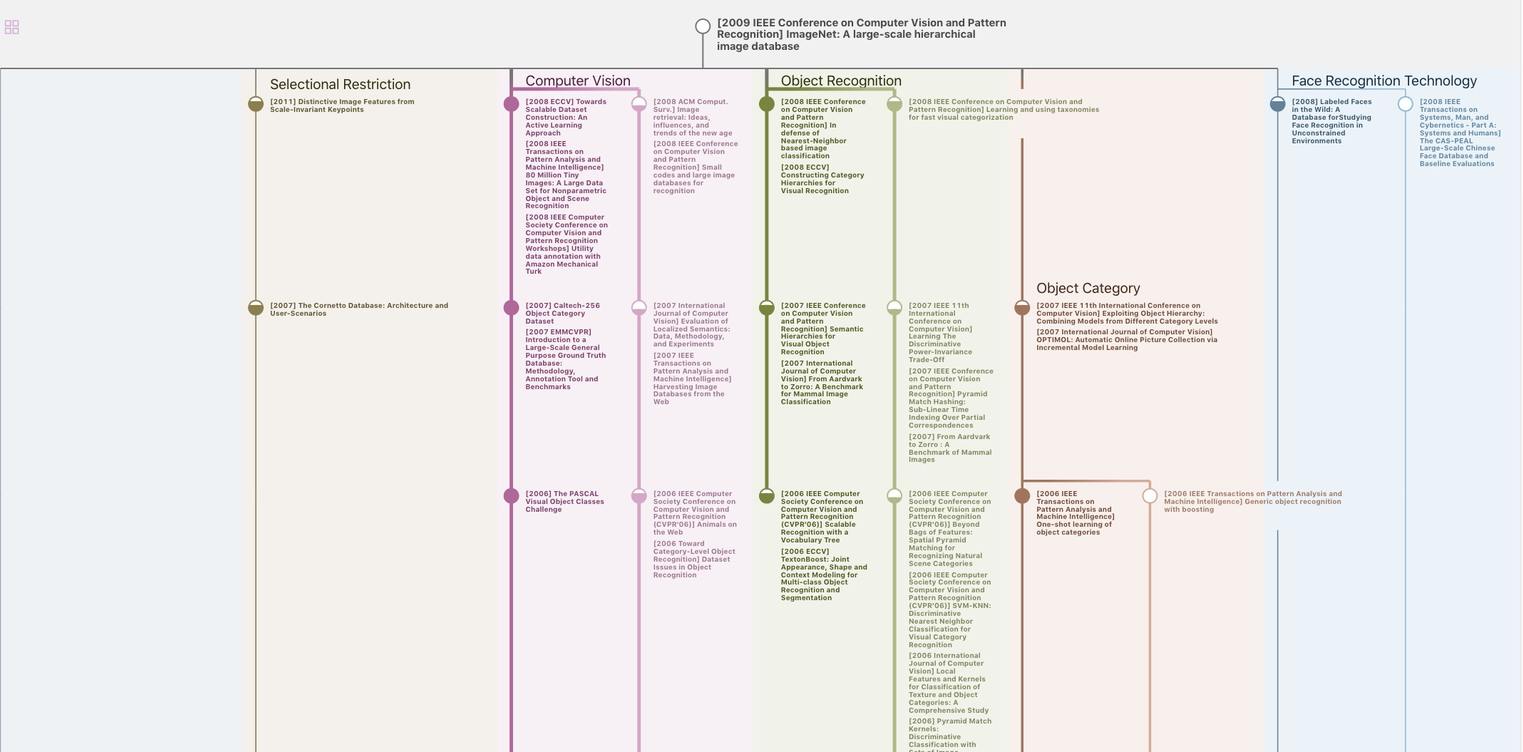
生成溯源树,研究论文发展脉络
Chat Paper
正在生成论文摘要