Temporal Dynamic Concept Modeling Network for Explainable Video Event Recognition
ACM Transactions on Multimedia Computing, Communications, and Applications(2022)
摘要
Recently, with the vigorous development of deep learning and multimedia technology, intelligent urban computing has received more and more extensive attention from academia and industry. Unfortunately, most of the related technologies are black-box paradigms that lack interpretability. Among them, video event recognition is a basic technology. Event contains multiple concepts and their rich interactions, which can assist us to construct explainable event recognition methods. However, the crucial concepts needed to recognize events have various temporal existing patterns, and the relationship between events and the temporal characteristics of concepts has not been fully exploited. This brings great challenges for concept-based event categorization. To address the above issues, we introduce the temporal concept receptive field, which is the length of the temporal window size required to capture key concepts for concept-based event recognition methods. Accordingly, we introduce the temporal dynamic convolution (TDC) to model the temporal concept receptive field dynamically according to different events. Its core idea is to combine the results of multiple convolution layers with the learned coefficients from two complementary perspectives. These convolution layers contain a variety of kernel sizes, which can provide temporal concept receptive fields of different lengths. Similarly, we also propose the cross-domain temporal dynamic convolution (CrTDC) with the help of the rich relationship between different concepts. Different coefficients can help us to capture suitable temporal concept receptive field sizes and highlight crucial concepts, so as to obtain accurate and complete concept representations for event analysis. Based on the TDC and CrTDC, we introduce the temporal dynamic concept modeling network (TDCMN) for explainable video event recognition. We evaluate TDCMN on large-scale and challenging datasets FCVID, ActivityNet, and CCV. Experimental results show that TDCMN significantly improves the event recognition performance of concept-based methods, and the explainability of our method inspires us to construct more explainable models from the perspective of the temporal concept receptive field.
更多查看译文
关键词
explainable video event recognition,dynamic,concept
AI 理解论文
溯源树
样例
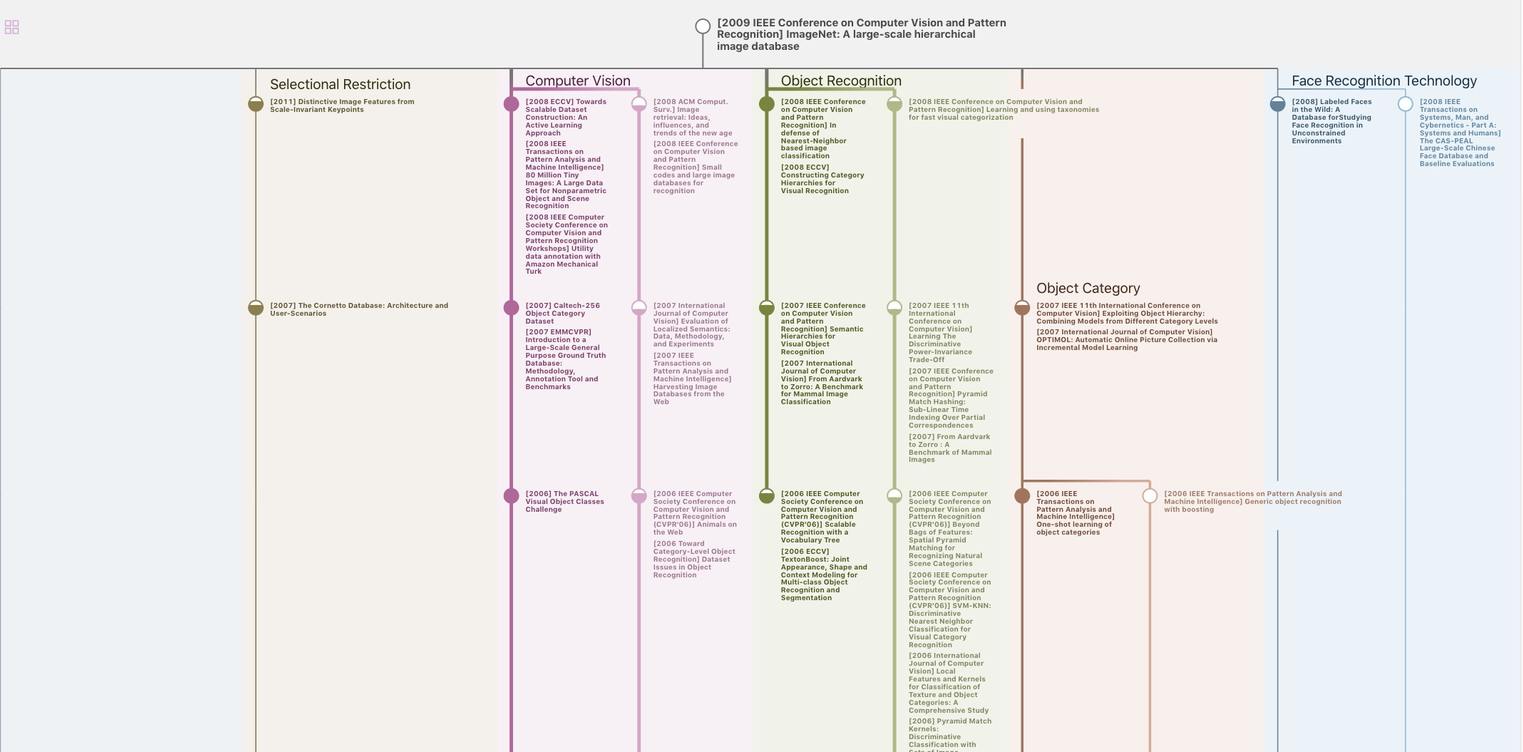
生成溯源树,研究论文发展脉络
Chat Paper
正在生成论文摘要