Gradient-based Weight Density Balancing for Robust Dynamic Sparse Training
arxiv(2022)
摘要
Training a sparse neural network from scratch requires optimizing connections at the same time as the weights themselves. Typically, the weights are redistributed after a predefined number of weight updates, removing a fraction of the parameters of each layer and inserting them at different locations in the same layers. The density of each layer is determined using heuristics, often purely based on the size of the parameter tensor. While the connections per layer are optimized multiple times during training, the density of each layer remains constant. This leaves great unrealized potential, especially in scenarios with a high sparsity of 90% and more. We propose Global Gradient-based Redistribution, a technique which distributes weights across all layers - adding more weights to the layers that need them most. Our evaluation shows that our approach is less prone to unbalanced weight distribution at initialization than previous work and that it is able to find better performing sparse subnetworks at very high sparsity levels.
更多查看译文
关键词
weight density balancing,robust dynamic sparse
AI 理解论文
溯源树
样例
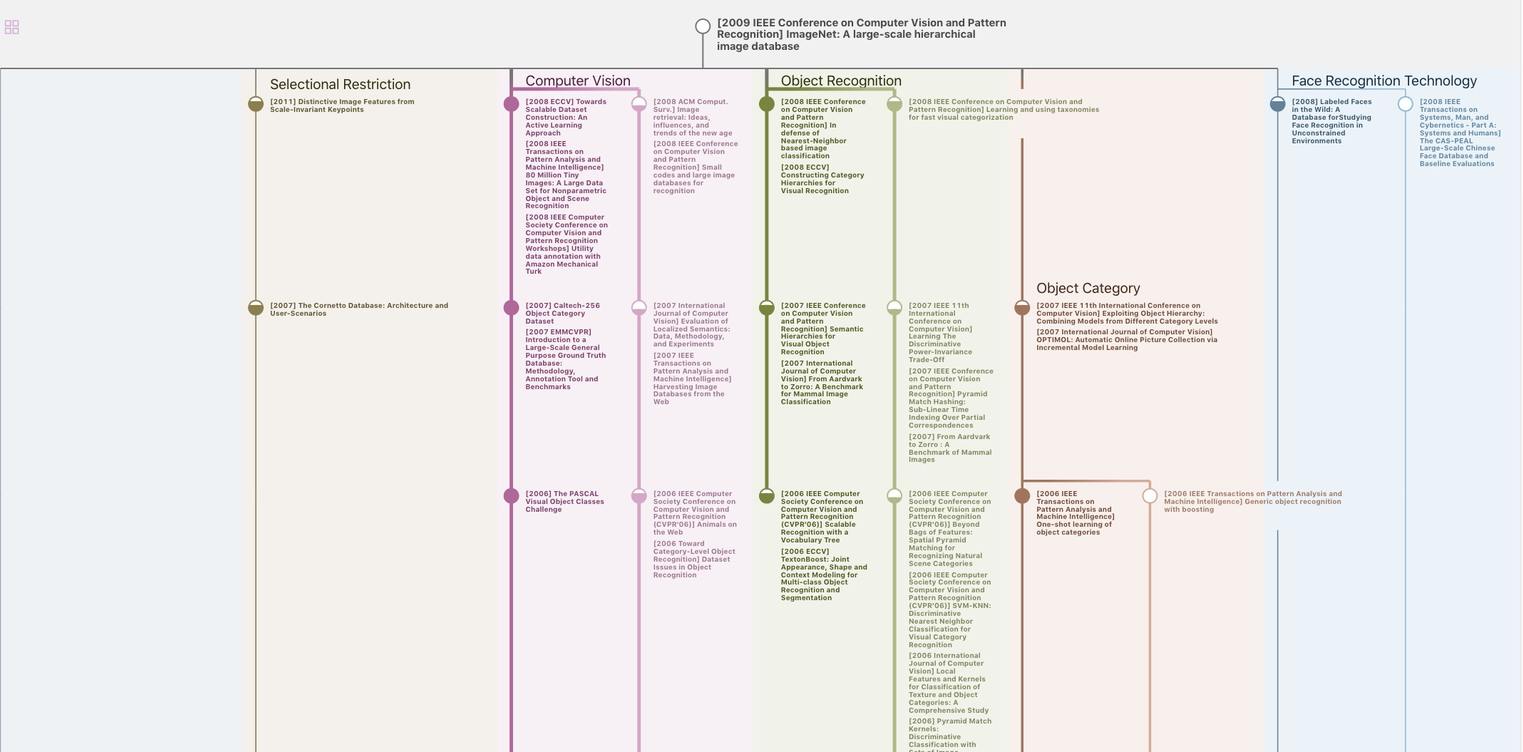
生成溯源树,研究论文发展脉络
Chat Paper
正在生成论文摘要