HiddenGems: Efficient safety boundary detection with active learning.
IEEE/RJS International Conference on Intelligent RObots and Systems (IROS)(2022)
摘要
Evaluating safety performance in a resource-efficient way is crucial for the development of autonomous systems. Simulation of parameterized scenarios is a popular testing strategy but parameter sweeps can be prohibitively expensive. To address this, we propose HiddenGems: a sample-efficient method for discovering the boundary between compliant and non-compliant behavior via active learning. Given a parameterized scenario, one or more compliance metrics, and a simulation oracle, HiddenGems maps the compliant and non-compliant domains of the scenario. The methodology enables critical test case identification, comparative analysis of different versions of the system under test, as well as verification of design objectives. We evaluate HiddenGems on a scenario with a jaywalker crossing in front of an autonomous vehicle and obtain compliance boundary estimates for collision, lane keep, and acceleration metrics individually and in combination, with 6 times fewer simulations than a parameter sweep. We also show how HiddenGems can be used to detect and rectify a failure mode for an unprotected turn with 86% fewer simulations.
更多查看译文
关键词
active learning,autonomous systems,autonomous vehicle,compliance boundary,critical test case identification,efficient safety boundary detection,HiddenGems,noncompliant behavior,safety performance evaluation,sample-efficient method
AI 理解论文
溯源树
样例
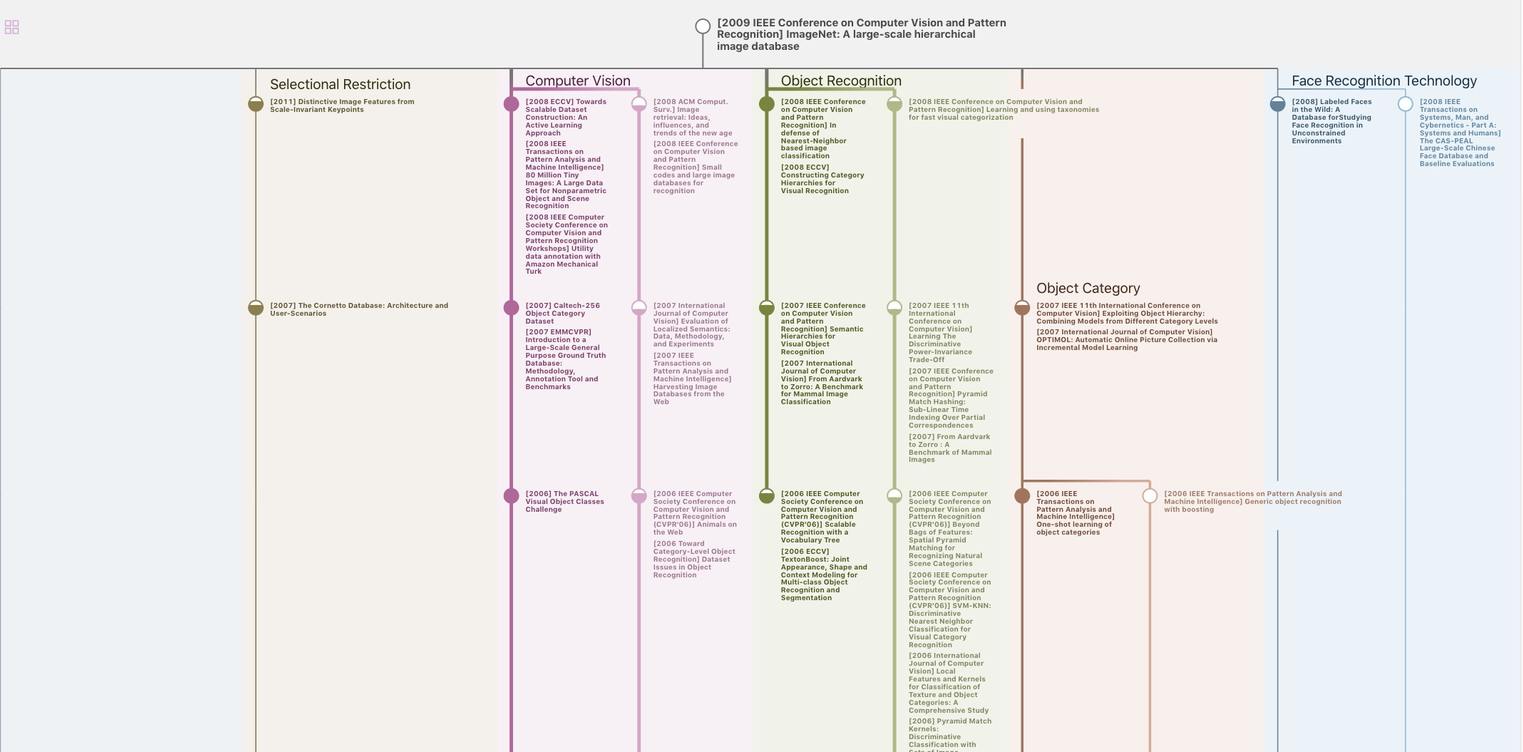
生成溯源树,研究论文发展脉络
Chat Paper
正在生成论文摘要