Structure-based Drug Design with Equivariant Diffusion Models
ICLR 2023(2022)
摘要
Structure-based drug design (SBDD) aims to design small-molecule ligands that bind with high affinity and specificity to pre-determined protein targets. Traditional SBDD pipelines start with large-scale docking of compound libraries from public databases, thus limiting the exploration of chemical space to existent previously studied regions. Recent machine learning methods approached this problem using an atom-by-atom generation approach, which is computationally expensive. In this paper, we formulate SBDD as a 3D-conditional generation problem and present DiffSBDD, an E(3)-equivariant 3D-conditional diffusion model that generates novel ligands conditioned on protein pockets. Furthermore, we curate a new dataset of experimentally determined binding complex data from Binding MOAD to provide a realistic binding scenario that complements the synthetic CrossDocked dataset. Comprehensive in silico experiments demonstrate the efficiency of DiffSBDD in generating novel and diverse drug-like ligands that engage protein pockets with high binding energies as predicted by in silico docking.
更多查看译文
关键词
Diffusion Models,Equivariant Neural Networks,Structure-based Drug Design,Molecule Generation,Conditional Generation
AI 理解论文
溯源树
样例
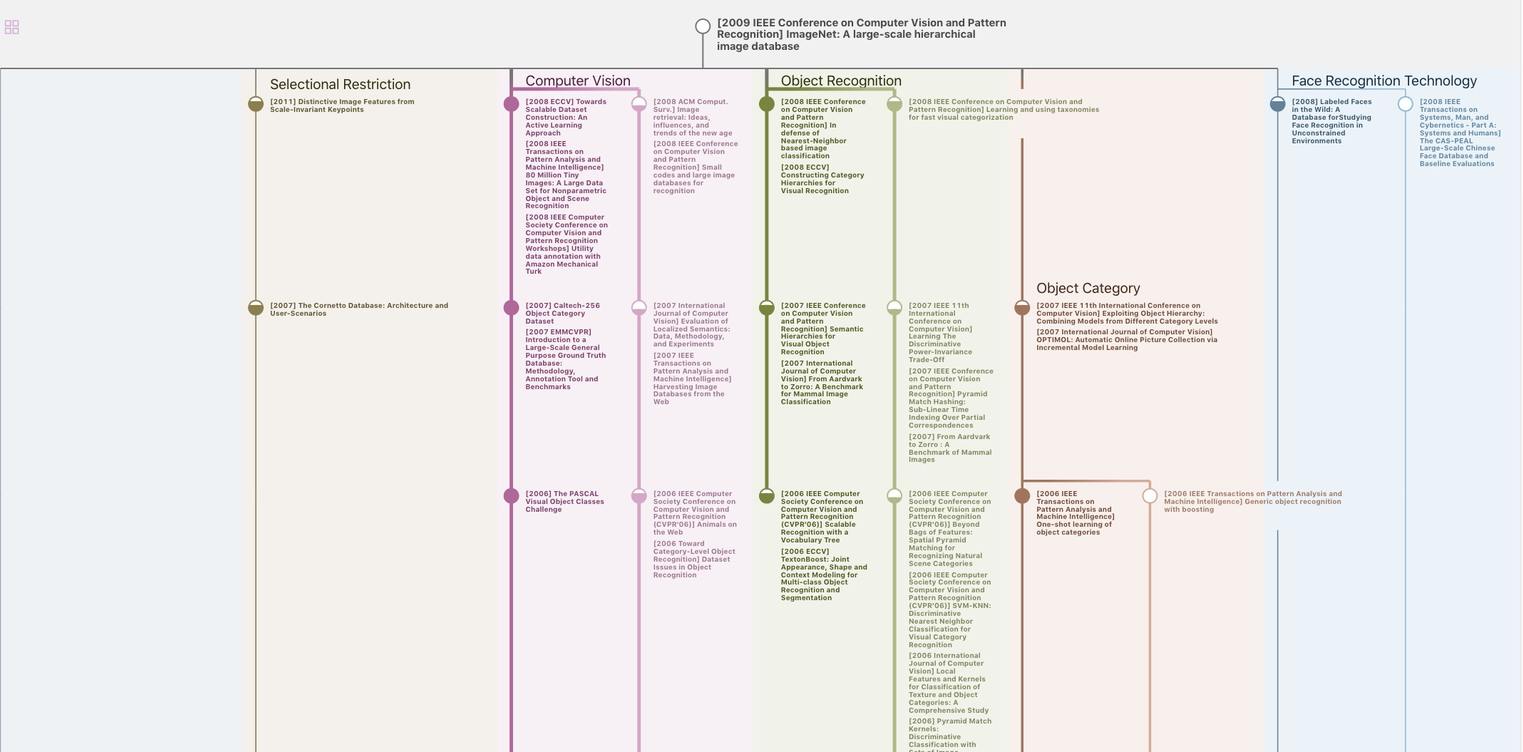
生成溯源树,研究论文发展脉络
Chat Paper
正在生成论文摘要