MEET: A Monte Carlo Exploration-Exploitation Trade-off for Buffer Sampling
arxiv(2023)
摘要
Data selection is essential for any data-based optimization technique, such as Reinforcement Learning. State-of-the-art sampling strategies for the experience replay buffer improve the performance of the Reinforcement Learning agent. However, they do not incorporate uncertainty in the Q-Value estimation. Consequently, they cannot adapt the sampling strategies, including exploration and exploitation of transitions, to the complexity of the task. To address this, this paper proposes a new sampling strategy that leverages the exploration-exploitation trade-off. This is enabled by the uncertainty estimation of the Q-Value function, which guides the sampling to explore more significant transitions and, thus, learn a more efficient policy. Experiments on classical control environments demonstrate stable results across various environments. They show that the proposed method outperforms state-of-the-art sampling strategies for dense rewards w.r.t. convergence and peak performance by 26% on average.
更多查看译文
关键词
uncertainty estimation,experience replay,reinforcement learning
AI 理解论文
溯源树
样例
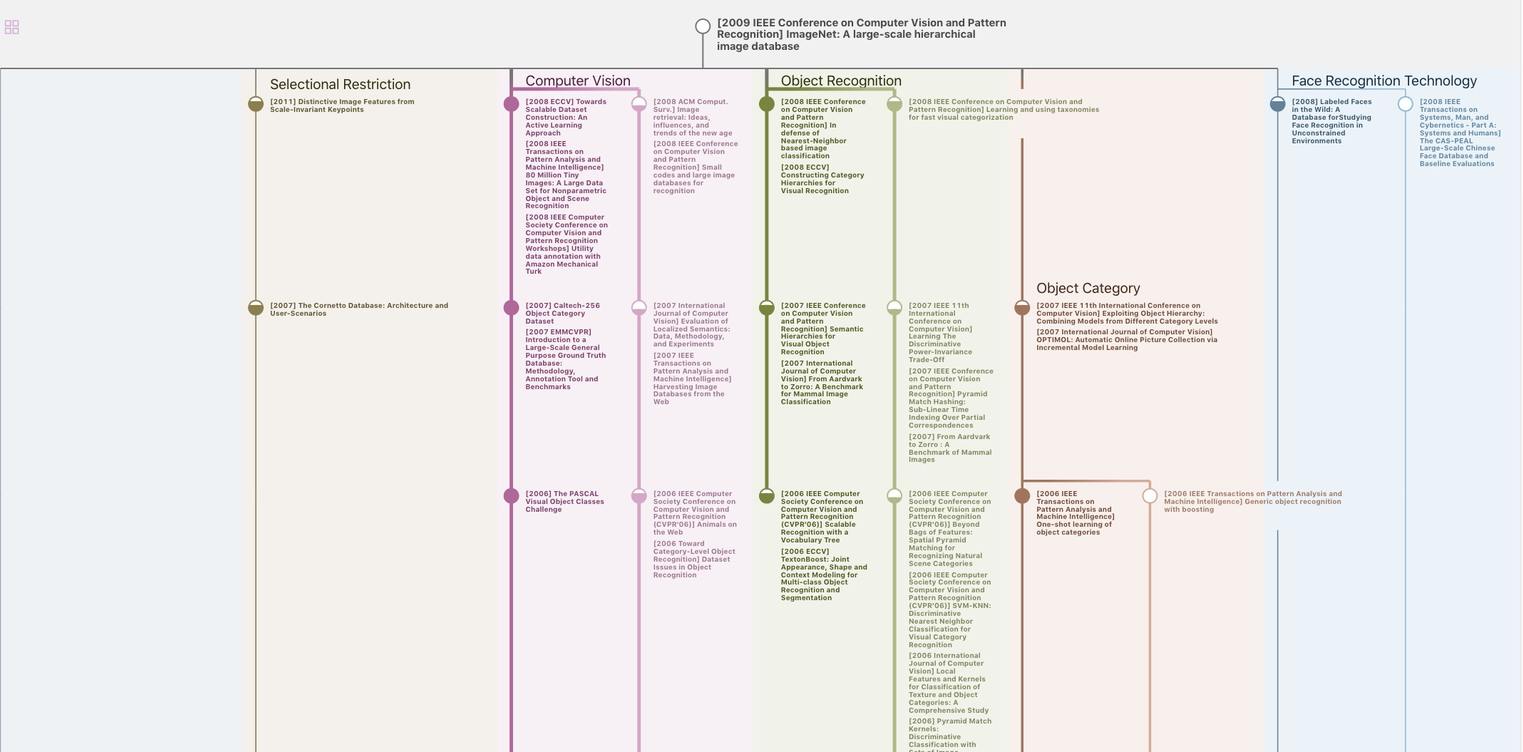
生成溯源树,研究论文发展脉络
Chat Paper
正在生成论文摘要