A PAC-Bayesian Generalization Bound for Equivariant Networks
NeurIPS 2022(2022)
摘要
Equivariant networks capture the inductive bias about the symmetry of the learning task by building those symmetries into the model. In this paper, we study how equivariance relates to generalization error utilizing PAC Bayesian analysis for equivariant networks, where the transformation laws of feature spaces are determined by group representations. By using perturbation analysis of equivariant networks in Fourier domain for each layer, we derive norm-based PAC-Bayesian generalization bounds. The bound characterizes the impact of group size, and multiplicity and degree of irreducible representations on the generalization error and thereby provide a guideline for selecting them. In general, the bound indicates that using larger group size in the model improves the generalization error substantiated by extensive numerical experiments.
更多查看译文
关键词
generalization error,equivariant networks,group representation,PAC Bayesian
AI 理解论文
溯源树
样例
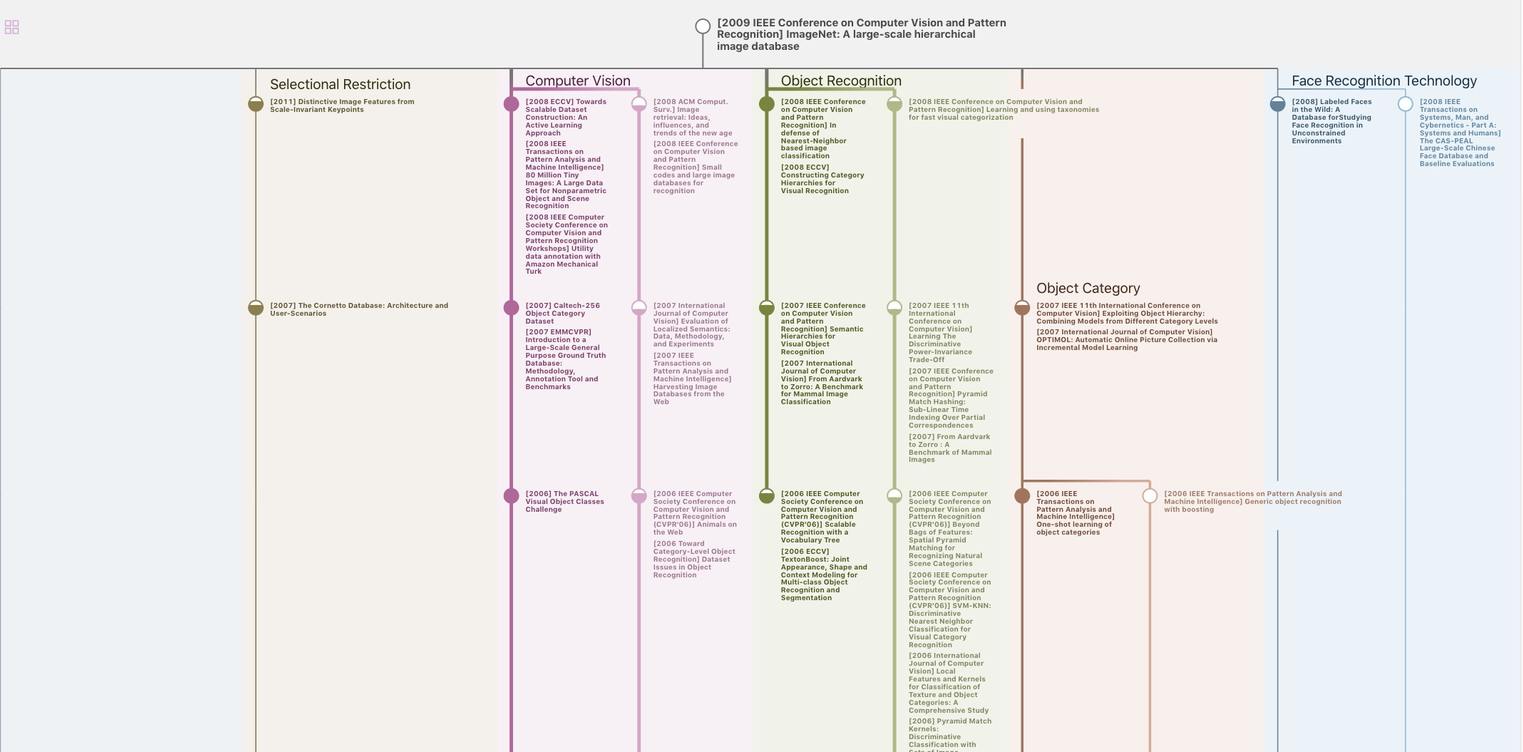
生成溯源树,研究论文发展脉络
Chat Paper
正在生成论文摘要