An Empirical Revisiting of Linguistic Knowledge Fusion in Language Understanding Tasks
emnlp 2022(2022)
摘要
Though linguistic knowledge emerges during large-scale language model pretraining, recent work attempt to explicitly incorporate human-defined linguistic priors into task-specific fine-tuning. Infusing language models with syntactic or semantic knowledge from parsers has shown improvements on many language understanding tasks. To further investigate the effectiveness of structural linguistic priors, we conduct empirical study of replacing parsed graphs or trees with trivial ones (rarely carrying linguistic knowledge e.g., balanced tree) for tasks in the GLUE benchmark. Encoding with trivial graphs achieves competitive or even better performance in fully-supervised and few-shot settings. It reveals that the gains might not be significantly attributed to explicit linguistic priors but rather to more feature interactions brought by fusion layers. Hence we call for attention to using trivial graphs as necessary baselines to design advanced knowledge fusion methods in the future.
更多查看译文
关键词
linguistic knowledge fusion,understanding tasks,language
AI 理解论文
溯源树
样例
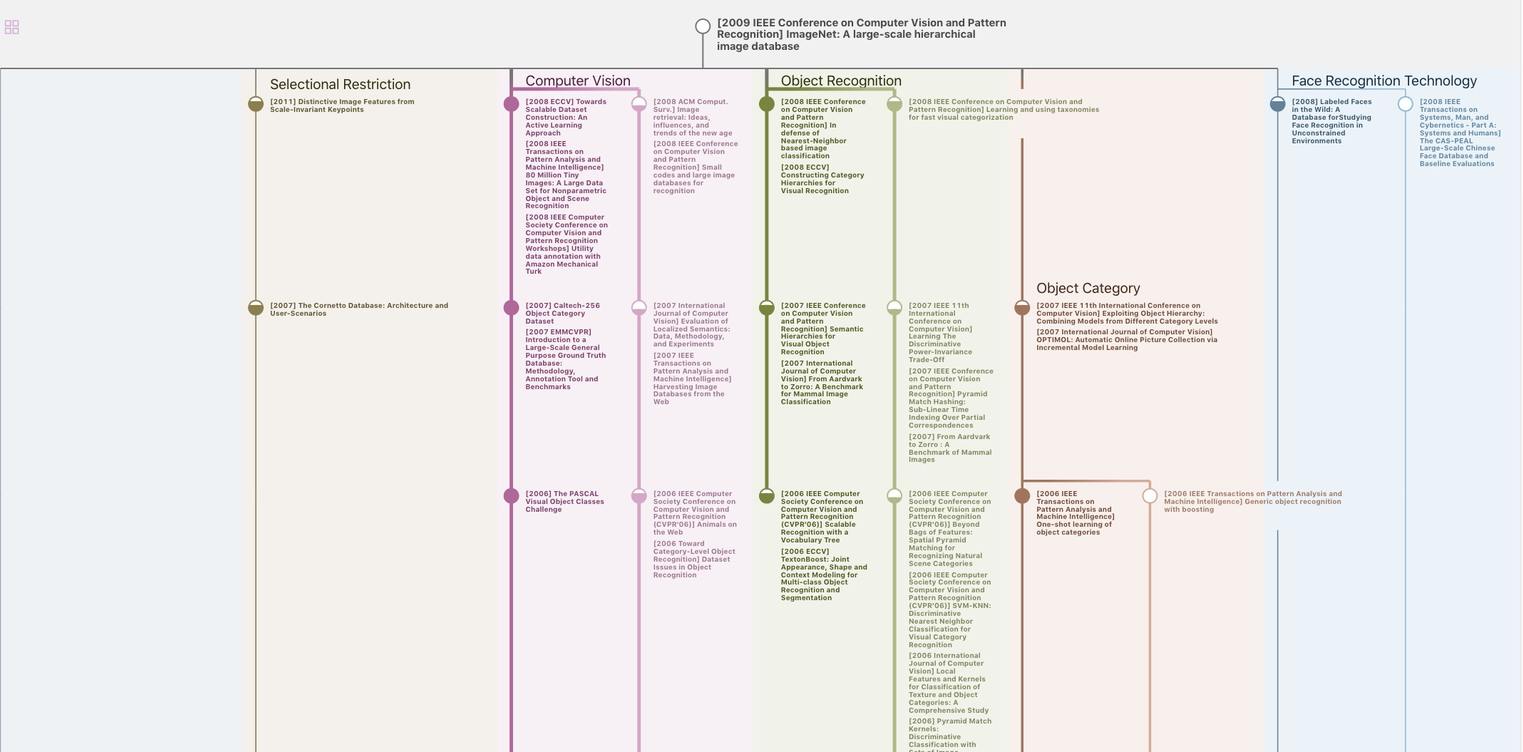
生成溯源树,研究论文发展脉络
Chat Paper
正在生成论文摘要