Investigating Neuron Disturbing in Fusing Heterogeneous Neural Networks
CoRR(2022)
摘要
Fusing deep learning models trained on separately located clients into a global model in a one-shot communication round is a straightforward implementation of Federated Learning. Although current model fusion methods are shown experimentally valid in fusing neural networks with almost identical architectures, they are rarely theoretically analyzed. In this paper, we reveal the phenomenon of neuron disturbing, where neurons from heterogeneous local models interfere with each other mutually. We give detailed explanations from a Bayesian viewpoint combining the data heterogeneity among clients and properties of neural networks. Furthermore, to validate our findings, we propose an experimental method that excludes neuron disturbing and fuses neural networks via adaptively selecting a local model, called AMS, to execute the prediction according to the input. The experiments demonstrate that AMS is more robust in data heterogeneity than general model fusion and ensemble methods. This implies the necessity of considering neural disturbing in model fusion. Besides, AMS is available for fusing models with varying architectures as an experimental algorithm, and we also list several possible extensions of AMS for future work.
更多查看译文
关键词
heterogeneous neural networks,neuron,neural networks
AI 理解论文
溯源树
样例
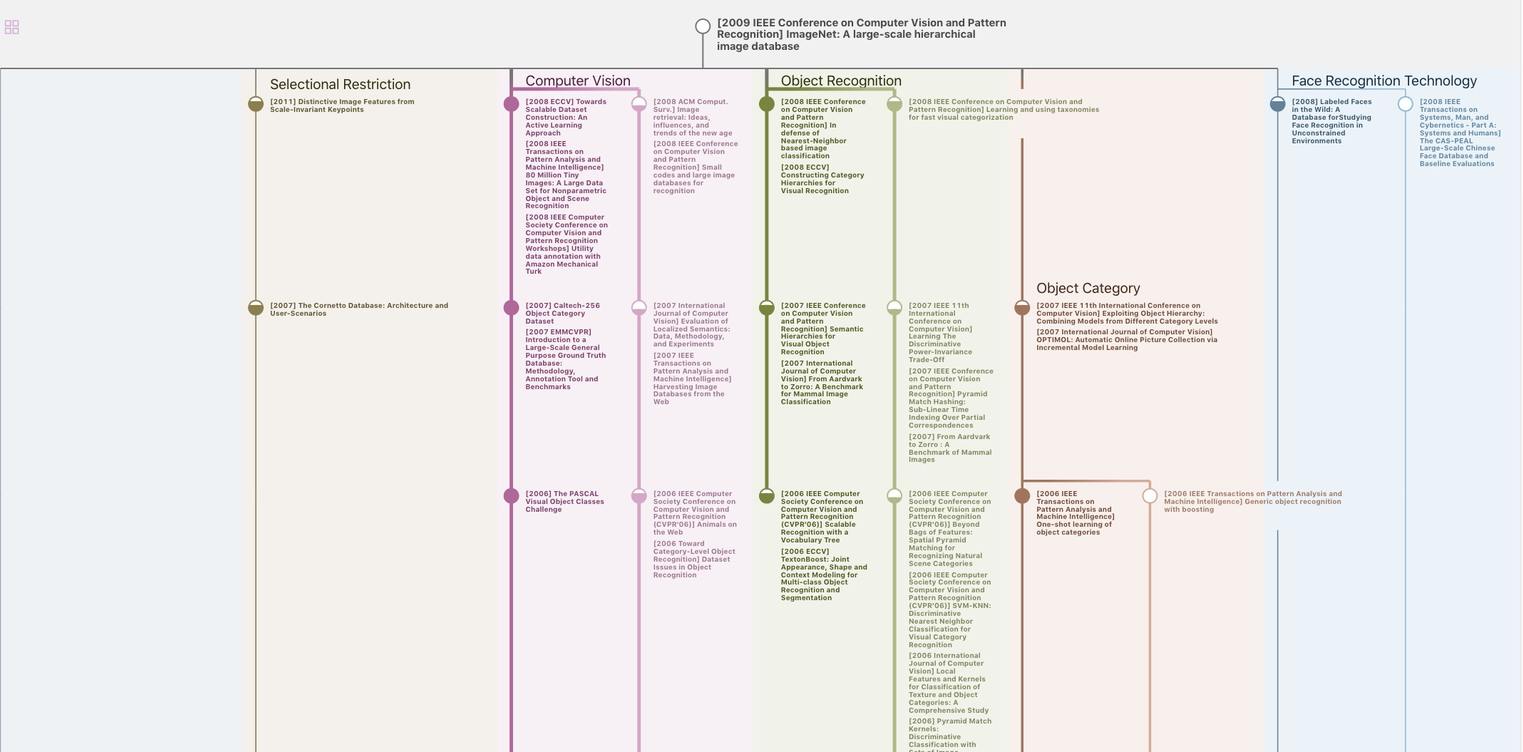
生成溯源树,研究论文发展脉络
Chat Paper
正在生成论文摘要