SpectraNet: Multivariate Forecasting and Imputation under Distribution Shifts and Missing Data
arxiv(2022)
摘要
In this work, we tackle two widespread challenges in real applications for time-series forecasting that have been largely understudied: distribution shifts and missing data. We propose SpectraNet, a novel multivariate time-series forecasting model that dynamically infers a latent space spectral decomposition to capture current temporal dynamics and correlations on the recent observed history. A Convolution Neural Network maps the learned representation by sequentially mixing its components and refining the output. Our proposed approach can simultaneously produce forecasts and interpolate past observations and can, therefore, greatly simplify production systems by unifying imputation and forecasting tasks into a single model. SpectraNet achieves SoTA performance simultaneously on both tasks on five benchmark datasets, compared to forecasting and imputation models, with up to 92% fewer parameters and comparable training times. On settings with up to 80% missing data, SpectraNet has average performance improvements of almost 50% over the second-best alternative. Our code is available at https://github.com/cchallu/spectranet.
更多查看译文
关键词
time series,forecasting,missing values,deep-learning,interpolation,distribution shift
AI 理解论文
溯源树
样例
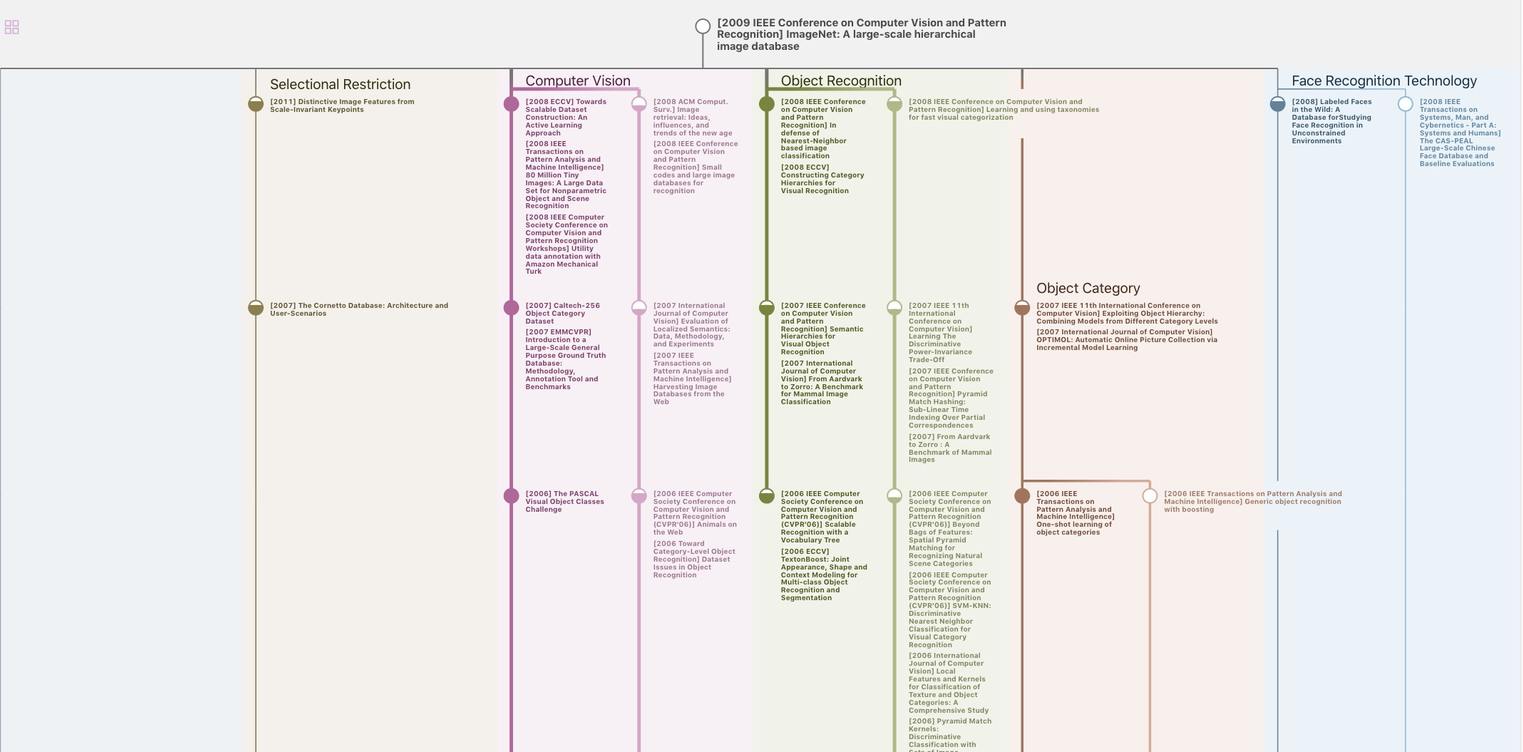
生成溯源树,研究论文发展脉络
Chat Paper
正在生成论文摘要