MasakhaNER 2.0: Africa-centric Transfer Learning for Named Entity Recognition
arxiv(2022)
摘要
African languages are spoken by over a billion people, but are underrepresented in NLP research and development. The challenges impeding progress include the limited availability of annotated datasets, as well as a lack of understanding of the settings where current methods are effective. In this paper, we make progress towards solutions for these challenges, focusing on the task of named entity recognition (NER). We create the largest human-annotated NER dataset for 20 African languages, and we study the behavior of state-of-the-art cross-lingual transfer methods in an Africa-centric setting, demonstrating that the choice of source language significantly affects performance. We show that choosing the best transfer language improves zero-shot F1 scores by an average of 14 points across 20 languages compared to using English. Our results highlight the need for benchmark datasets and models that cover typologically-diverse African languages.
更多查看译文
关键词
transfer learning,named entity
AI 理解论文
溯源树
样例
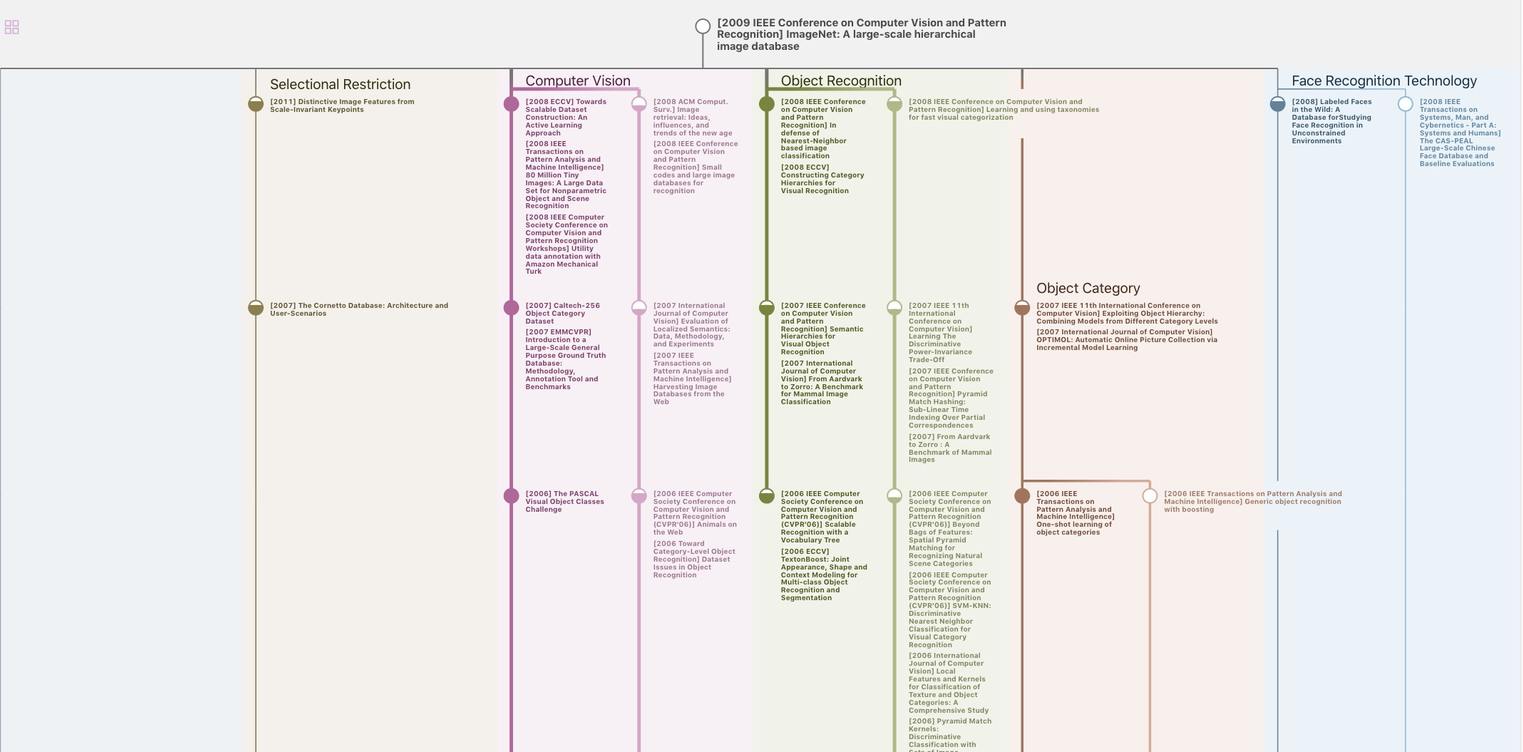
生成溯源树,研究论文发展脉络
Chat Paper
正在生成论文摘要