Online Adaptive Controller Selection in Time-Varying Systems: No-Regret via Contractive Perturbations
NeurIPS(2022)
摘要
We study the problem of online controller selection in systems with time-varying costs and dynamics. We focus on settings where the closed-loop dynamics induced by the policy class satisfy a contractive perturbation property that generalizes an established property of disturbance-feedback controllers. When the policy class is continuously parameterized, under the additional assumption that past dynamics and cost Jacobians are known, we propose Gradient-based Adaptive Policy Selection (GAPS), which achieves a time-averaged adaptive policy regret of $O(1/\sqrt{T})$. Compared with previous work on disturbance feedback controllers in linear systems, our result applies to a more general setting and improves the regret bound by a factor of $\log T$. When the policy class is finite, we propose Bandit-based Adaptive Policy Selection (BAPS), which achieves a time-averaged policy regret of $O(T^{-1/3})$. We apply the proposed algorithms to the setting of Model Predictive Control (MPC) with unreliable predictions to optimize continuous "confidence" parameters (GAPS) and select the MPC horizon (BAPS), and demonstrate good results for GAPS on a nonlinear system that does not fully satisfy the conditions of our regret bound.
更多查看译文
AI 理解论文
溯源树
样例
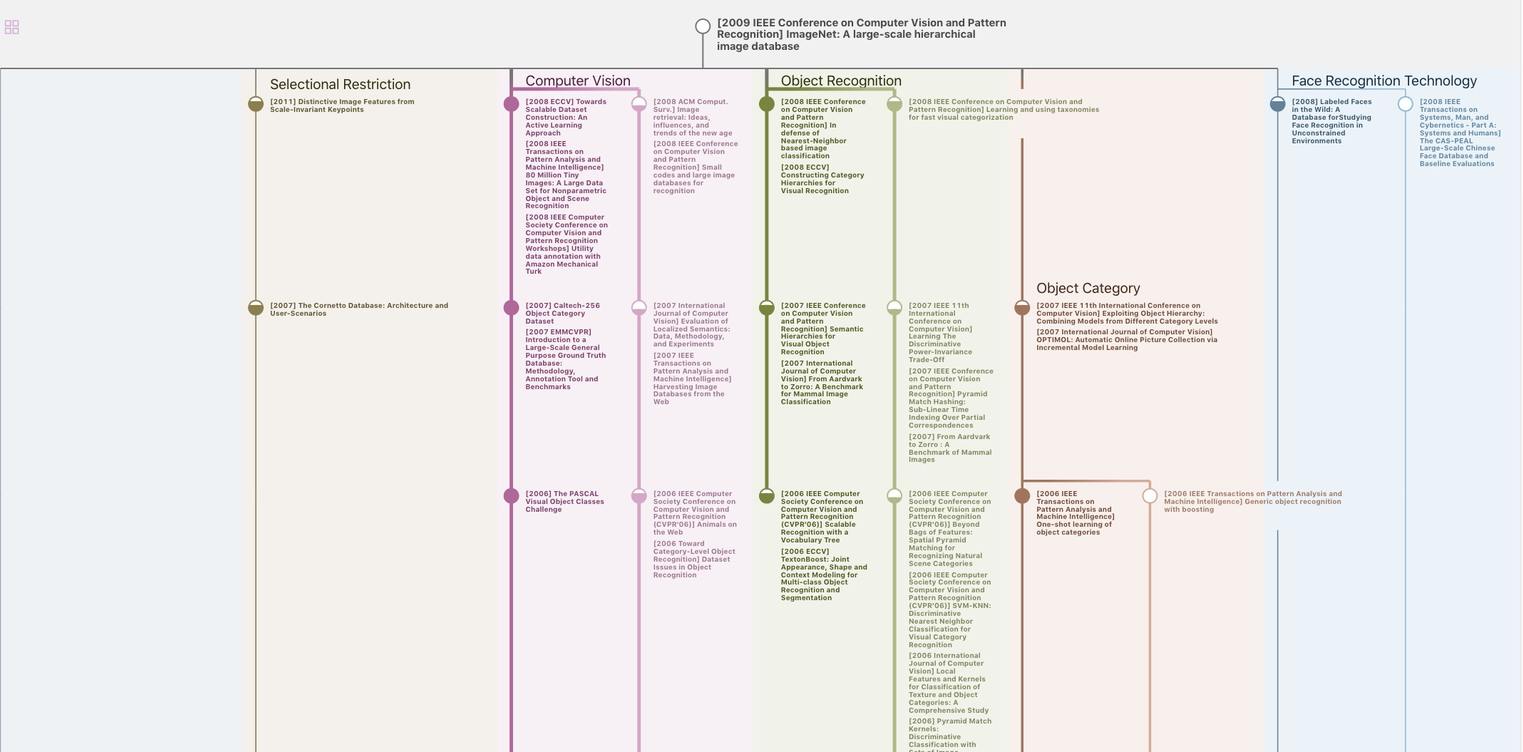
生成溯源树,研究论文发展脉络
Chat Paper
正在生成论文摘要