Improving Medical Predictions by Irregular Multimodal Electronic Health Records Modeling
arxiv(2022)
摘要
Health conditions among patients in intensive care units (ICUs) are monitored via electronic health records (EHRs), composed of numerical time series and lengthy clinical note sequences, both taken at irregular time intervals. Dealing with such irregularity in every modality, and integrating irregularity into multimodal representations to improve medical predictions, is a challenging problem. In this paper, we address this problem by (1) modeling irregular time series by incorporating hand-crafted imputation embeddings into learned interpolation embeddings via a gating mechanism; (2) casting a series of clinical note representations as multivariate irregular time series and tackling irregularity via a time attention mechanism; and (3) fusing multimodalities with an interleaved attention mechanism across temporal steps to integrate irregularity into multimodal representations. To the best of our knowledge, this is the first work to thoroughly model irregularity in multimodalities and to take into account temporal knowledge in multimodal fusion, for improving medical predictions. The results on two medical prediction tasks show that our proposed methods outperform the state-of-the-art (SOTA) methods in both every single modality and multimodal fusion scenarios, illustrating the effectiveness of our methods and the value of modeling irregularity in multimodal fusion.
更多查看译文
关键词
irregular multimodal electronic health
AI 理解论文
溯源树
样例
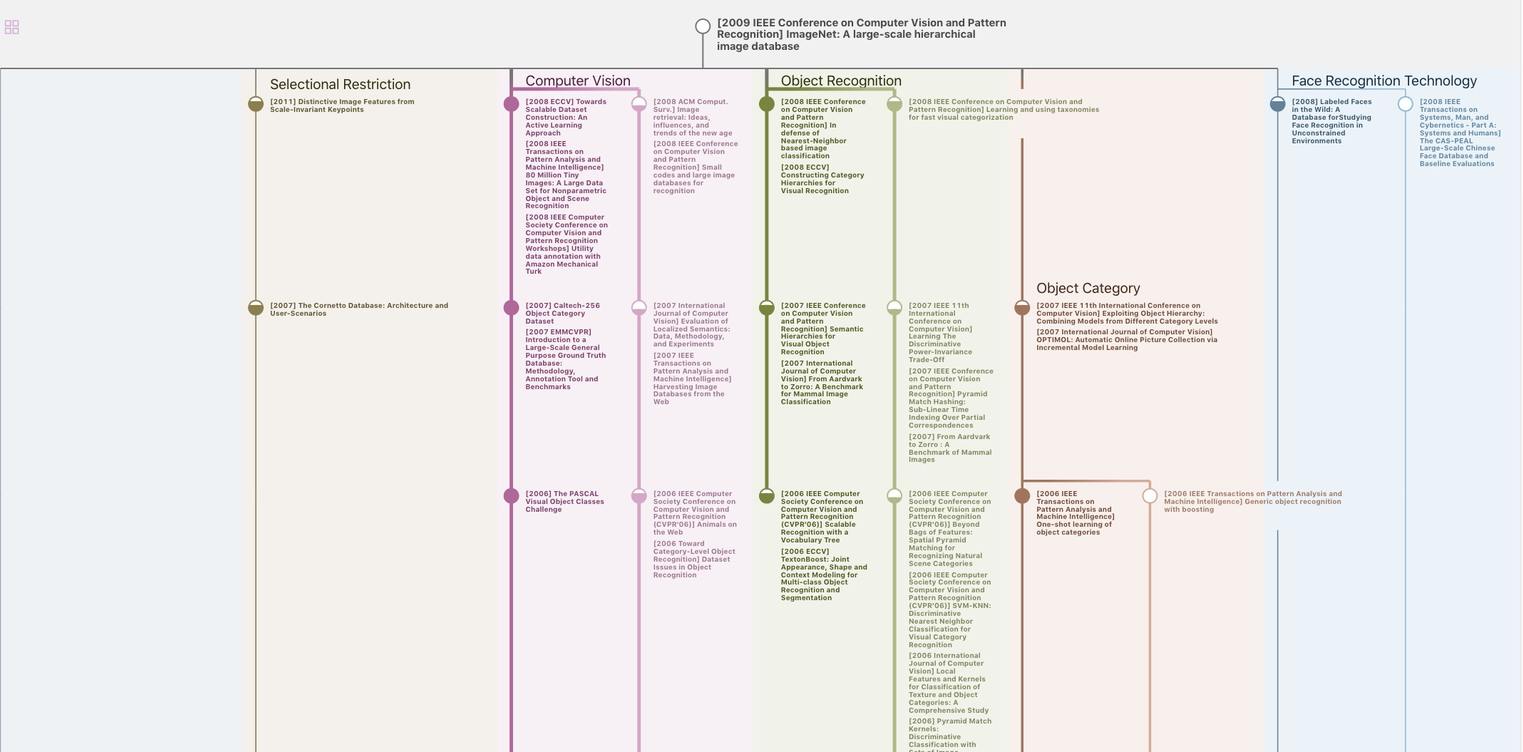
生成溯源树,研究论文发展脉络
Chat Paper
正在生成论文摘要