Robust Singular Values based on L1-norm PCA
2022 IEEE Workshop on Signal Processing Systems (SiPS)(2022)
摘要
Singular-Value Decomposition (SVD) is a ubiquitous data analysis method in engineering, science, and statistics. Singular-value estimation, in particular, is of critical importance in an array of engineering applications, such as channel estimation in communication systems, electromyography signal analysis, and image compression, to name just a few. Conventional SVD of a data matrix coincides with standard Principal-Component Analysis (PCA). The L2-norm (sum of squared values) formulation of PCA promotes peripheral data points and, thus, makes PCA sensitive against outliers. Naturally, SVD inherits this outlier sensitivity. In this work, we present a novel robust non-parametric method for SVD and singular-value estimation based on a L1-norm (sum of absolute values) formulation, which we name L1-cSVD. Accordingly, the proposed method demonstrates sturdy resistance against outliers and can facilitate more reliable data analysis and processing in a wide range of engineering applications.
更多查看译文
关键词
Singular value decomposition,principal component analysis,subspace signal processing,outliers
AI 理解论文
溯源树
样例
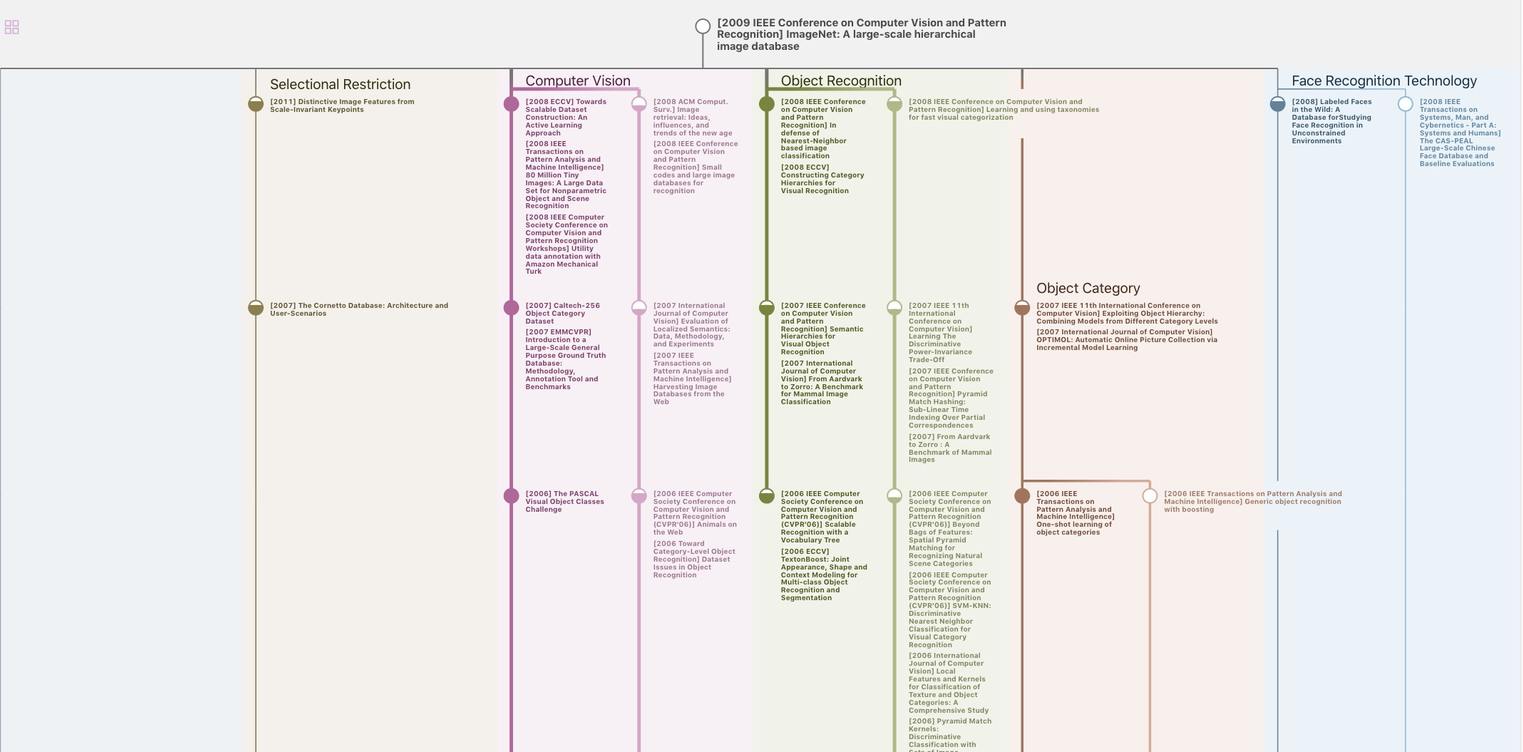
生成溯源树,研究论文发展脉络
Chat Paper
正在生成论文摘要