Efficiently Tuned Parameters are Task Embeddings
emnlp 2022(2022)
摘要
Intermediate-task transfer can benefit a wide range of NLP tasks with properly selected source datasets. However, it is computationally infeasible to experiment with all intermediate transfer combinations, making choosing a useful source task a challenging problem. In this paper, we anticipate that task-specific parameters updated in parameter-efficient tuning methods are likely to encode task-specific information. Therefore, such parameters can be predictive for inter-task transferability. Thus, we propose to exploit these efficiently tuned parameters as off-the-shelf task embeddings for the efficient selection of source datasets for intermediate-task transfer. We experiment with 11 text classification tasks and 11 question answering tasks. Experimental results show that our approach can consistently outperform existing inter-task transferability prediction methods while being conceptually simple and computationally efficient. Our analysis also reveals that the ability of efficiently tuned parameters on transferability prediction is disentangled with their in-task performance. This allows us to use parameters from early checkpoints as task embeddings to further improve efficiency.
更多查看译文
关键词
tuned parameters,task
AI 理解论文
溯源树
样例
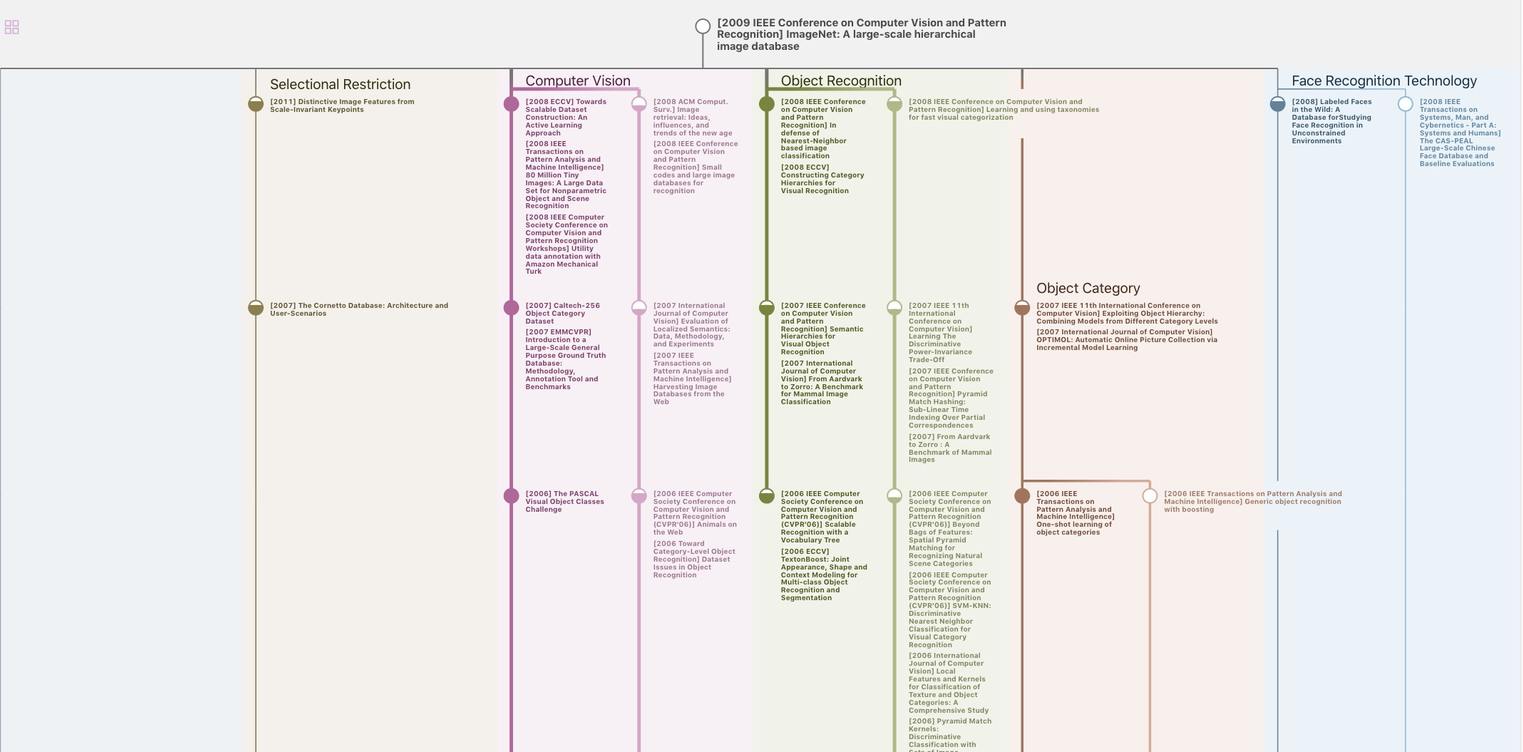
生成溯源树,研究论文发展脉络
Chat Paper
正在生成论文摘要