A Prospective Observational Study of Clinical Acceptability of Deep Learning Model for the Automated Segmentation of Organs at Risk for Head and Neck Radiotherapy Treatment Planning
International Journal of Radiation Oncology*Biology*Physics(2022)
摘要
Purpose/Objective(s)
Accurate organ-at-risk (OAR) contouring is essential to improve plan quality. Head and neck (H&N) cancer has many OARs and contouring requires substantial time and effort. We developed a deep learning model (DLM) for autosegmentation of H&N OARs and performed a prospective study to assess time savings using DLM to generate contours for review compared to those created by medical dosimetrist's assistants (MDAs) with experience in H&N OAR contouring as part of routine practice. A prospective study was run to assess if the use of the DLM would reduce contouring time by more than 30%.Materials/Methods
Using a custom 3D U-net architecture operating on 32 × 512 × 512-voxel subvolumes, the DLM was trained to segment 42 OARs for H&N cancer using retrospective planning CTs with OAR contours generated by H&N expert radiation oncologists (ROs) using sufficient protected time to represent the institutional "gold standard" (312 training, 51 model tuning, 82 testing), with 20 held out for prospective validation through an IRB-approved study with H&N specialized MDAs and ROs as consenting participants. The prospective study involved two arms for each hold out CT: the standard arm involved MDAs creating initial contours followed by revision by an RO (Manual arm) and initial contours for the same CT created by the DLM and revised by a different H&N RO (AI arm), the ROs were blinded to the origin of the initial contours and were randomly assigned an equal number of cases from each arm and asked to revise the contours to meet clinical standards for planning. The study was powered to detect greater than 30% reduction in contouring time (α = 0.025). Final RO-edited contours from each arm were compared using Dice Similarity Coefficient (DSC) to the gold standard (GS). Participant surveys were obtained after each session and the completion of the study.Results
The mean total time for the manual arm was 3.4 hours (95% CI, 2.9-3.9 hours), while for the AI arm it was 0.7 hours (95% CI, 0.6-1.6 hours), a reduction of 76% (95% CI, 65%-88%) which was significantly greater than 30% (p<0.0001). 36 OARs (86%) had mean DSC greater than 0.8 on the AI arm compared to 20 (45%) for the Manual arm. The mean DSC for select OARs are given in table below. Survey of ROs showed satisfaction with DLM contours and interest in using for future cases.Conclusion
The DLM demonstrated a significant reduction in the time needed for contouring the OARs for H&N cancer while improving agreement with the institutional gold standard. Integration of the DLM into the clinic for prospective evaluation is underway.查看译文
关键词
deep learning model,radiotherapy,deep learning,automated segmentation,organs
AI 理解论文
溯源树
样例
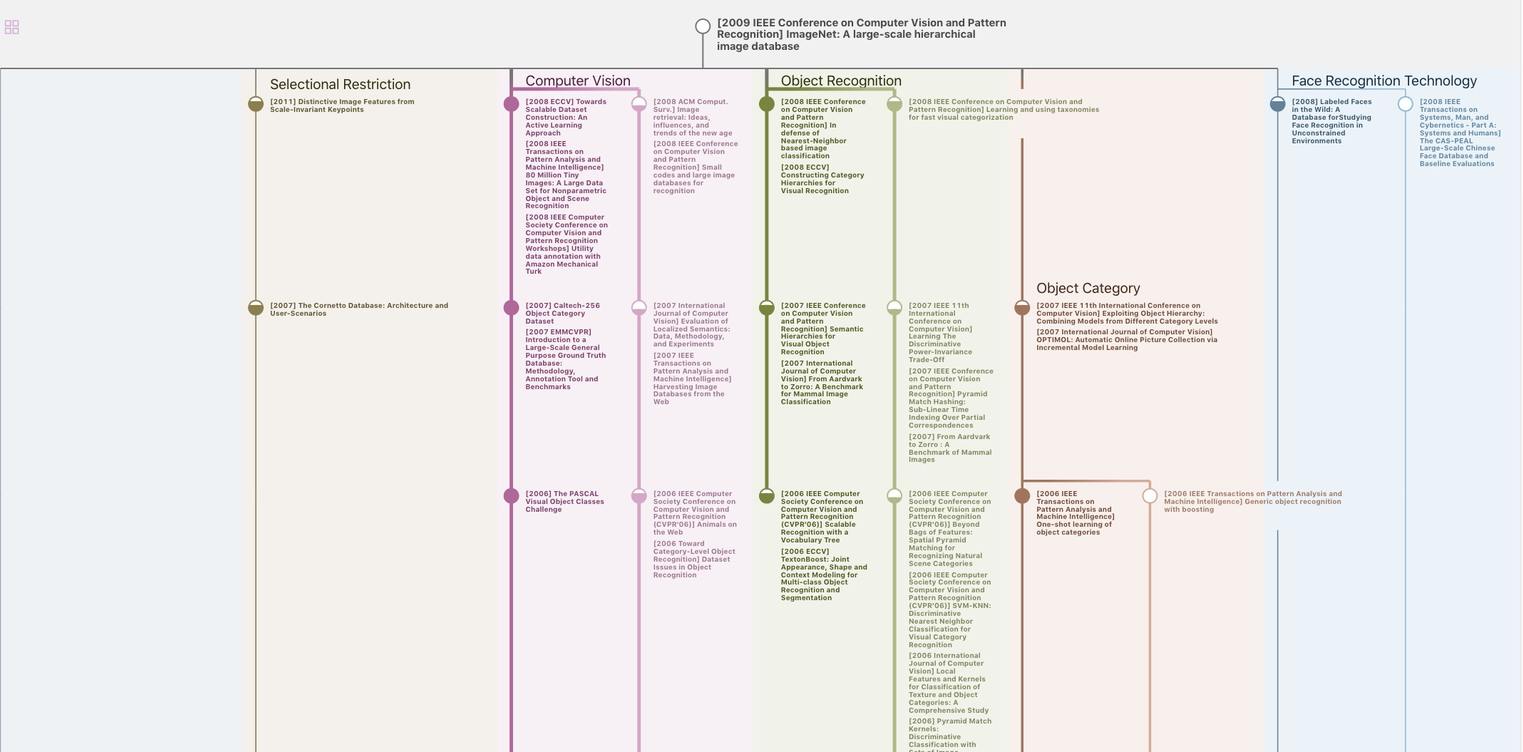
生成溯源树,研究论文发展脉络
Chat Paper
正在生成论文摘要