A copula-based set-variant association test for bivariate continuous, binary or mixed phenotypes
The International Journal of Biostatistics(2022)
摘要
Abstract In genome wide association studies (GWAS), researchers are often dealing with dichotomous and non-normally distributed traits, or a mixture of discrete-continuous traits. However, most of the current region-based methods rely on multivariate linear mixed models (mvLMMs) and assume a multivariate normal distribution for the phenotypes of interest. Hence, these methods are not applicable to disease or non-normally distributed traits. Therefore, there is a need to develop unified and flexible methods to study association between a set of (possibly rare) genetic variants and non-normal multivariate phenotypes. Copulas are multivariate distribution functions with uniform margins on the [0, 1] interval and they provide suitable models to deal with non-normality of errors in multivariate association studies. We propose a novel unified and flexible copula-based multivariate association test (CBMAT) for discovering association between a genetic region and a bivariate continuous, binary or mixed phenotype. We also derive a data-driven analytic p-value procedure of the proposed region-based score-type test. Through simulation studies, we demonstrate that CBMAT has well controlled type I error rates and higher power to detect associations compared with other existing methods, for discrete and non-normally distributed traits. At last, we apply CBMAT to detect the association between two genes located on chromosome 11 and several lipid levels measured on 1477 subjects from the ASLPAC study.
更多查看译文
关键词
copulas,generalized linear mixed models,gene-based tests,mixed binary-continuous phenotypes,statistical genetics,variance component score test
AI 理解论文
溯源树
样例
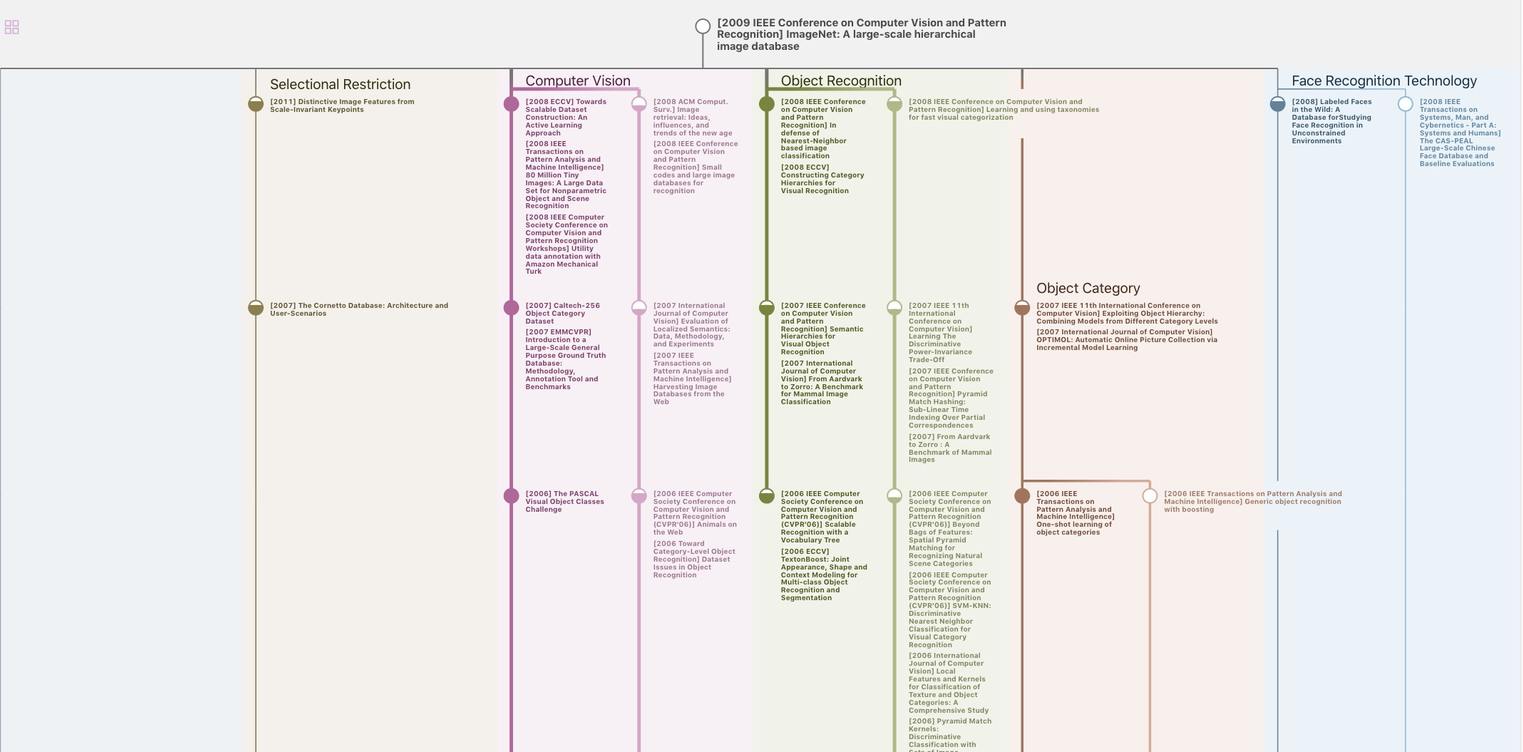
生成溯源树,研究论文发展脉络
Chat Paper
正在生成论文摘要