Nonparametric Bayesian functional selection in 1-M matched case-crossover studies.
Statistical methods in medical research(2023)
摘要
The matched case-crossover study has been used in many areas such as public health, biomedical, and epidemiological research for humans, animals, and other subjects with clustered binary outcomes. The control information for each stratum is based on the subject's exposure experience, and the stratifying variable is the individual subject. It is generally accepted that any effects associated with the matching covariates by stratum can be removed in the conditional logistic regression model. However, when there are numerous covariates, it is important to perform variable selection to study the functional association between the variables and the relative risk of diseases or clustered binary outcomes by simultaneously adjusting effect modifications. The methods for simultaneously evaluating effect modifications by matching covariates such as time, as well as performing automatic variable and functional selections under semiparametric model frameworks, are quite limited. In this article, we propose a unified Bayesian approach due to its ability to detect both parametric and nonparametric relationships between the predictors and the relative risk of diseases or binary outcomes, accounting for potential effect modifications by matching covariates such as time, and perform automatic variable and functional selections. We demonstrate the advantages of our approach using simulation study and an epidemiological example of a 1-4 bidirectional case-crossover study.
更多查看译文
关键词
Functional selection,matched case-crossover study,time-varying coefficient
AI 理解论文
溯源树
样例
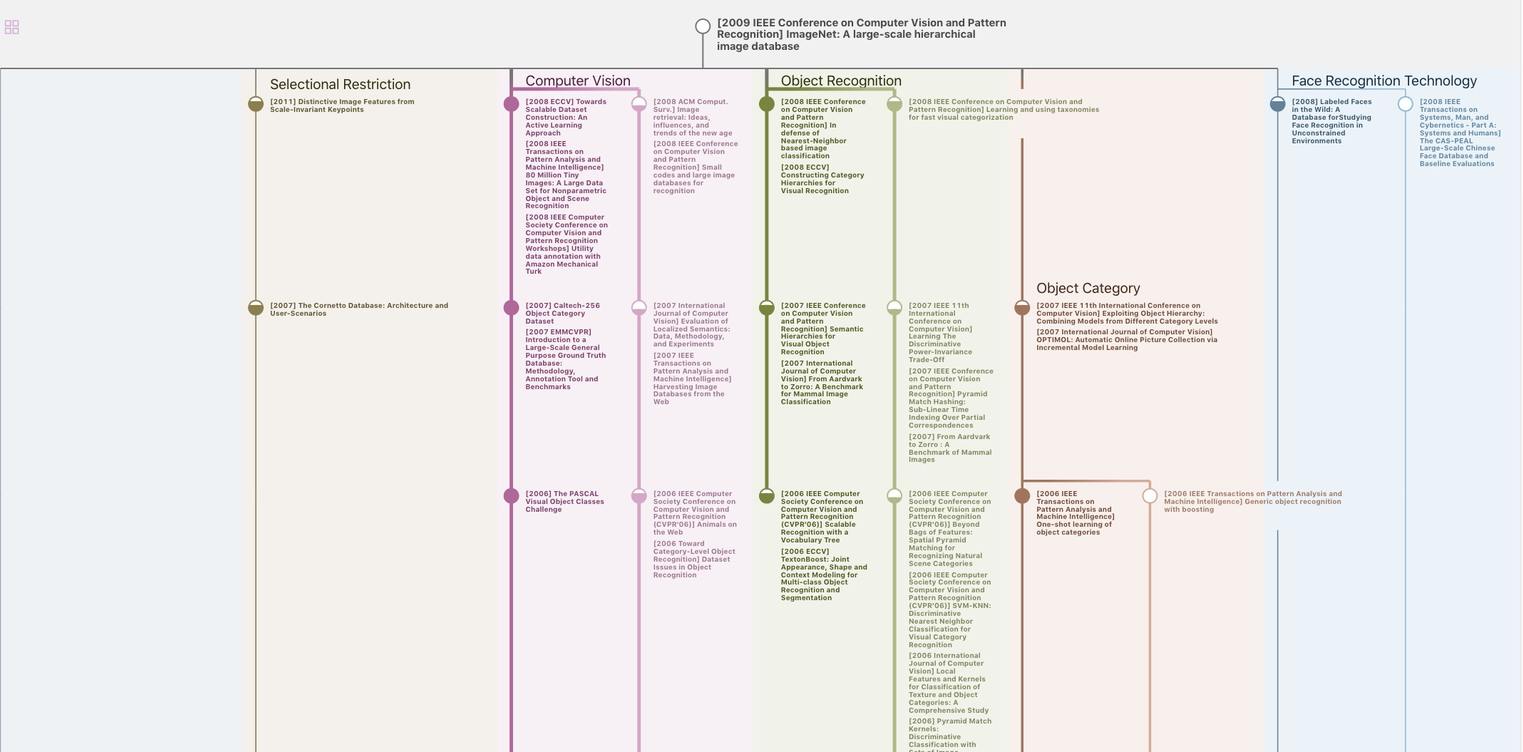
生成溯源树,研究论文发展脉络
Chat Paper
正在生成论文摘要