Deepwalk-aware graph convolutional networks
Science China Information Sciences(2022)
摘要
Graph convolutional networks (GCNs) provide a promising way to extract the useful information from graph-structured data. Most of the existing GCNs methods usually focus on local neighborhood information based on specific convolution operations, and ignore the global structure of the input data. To extract the latent representation for the graph-structured data more effectively, we introduce a deepwalk strategy into GCNs to efficiently explore the global graph information. This strategy can complement the local neighborhood information of a graph, resulting in the more robust representation for the graph data. The fusion of the local neighboring and global structured information of a graph can further facilitate deep feature learning at the output layer of GCNs for node classification. Experimental results show that the proposed model has achieved state-of-the-art results on three benchmark datasets including Cora, Citeseer, and Pubmed citation networks.
更多查看译文
关键词
graph,convolutional networks,global information,fusion,node classification
AI 理解论文
溯源树
样例
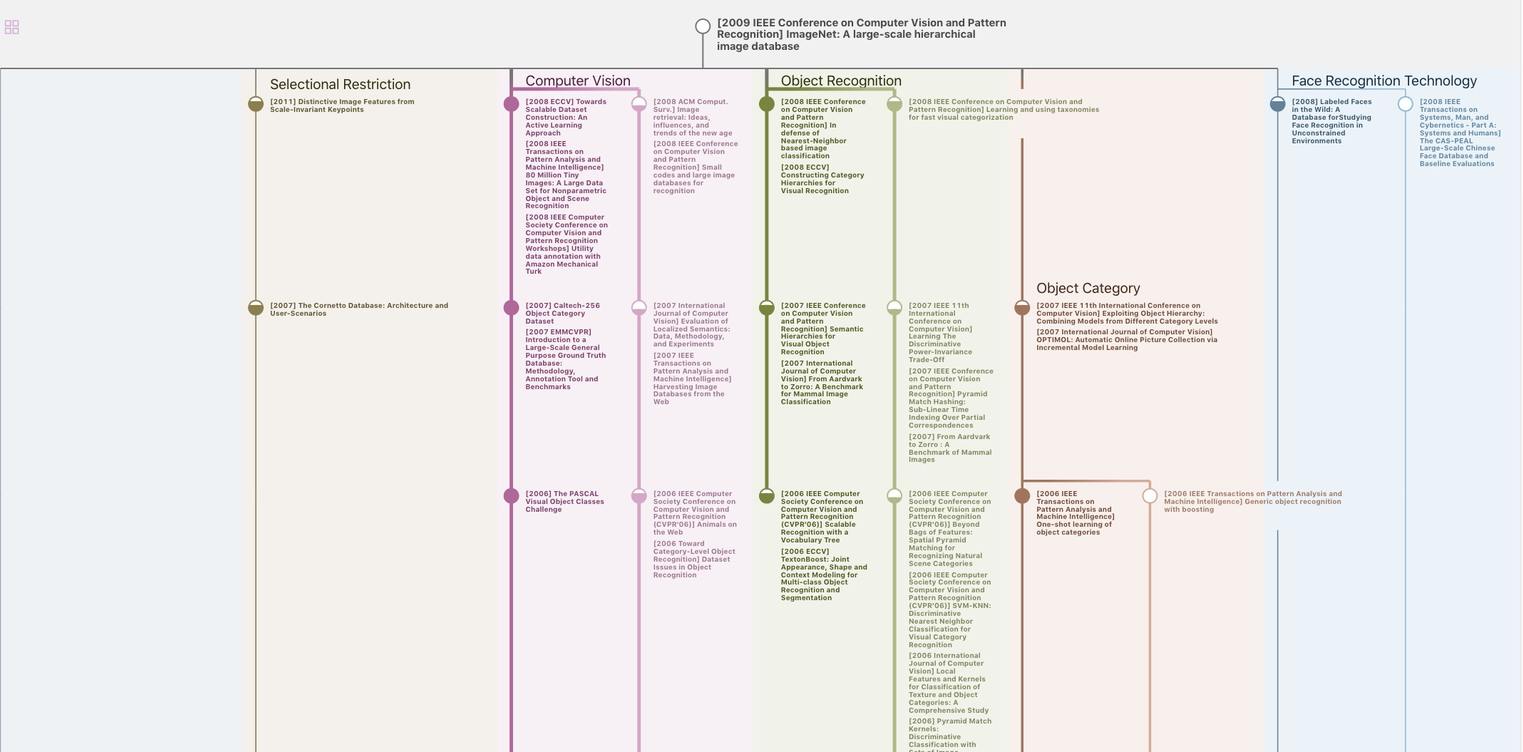
生成溯源树,研究论文发展脉络
Chat Paper
正在生成论文摘要