Improving Interpretability of Machine Learning in Head and Neck Radiation Therapy Pretreatment Physics Plan Reviews
International Journal of Radiation Oncology*Biology*Physics(2022)
摘要
Purpose/Objective(s)
Quality assurance (QA) measures such as pre-treatment physics plan review are central to the patient safety and quality of radiation therapy (RT) care. Performing QA tasks for Head and Neck (H&N) RT plans is cognitively demanding and time intensive. The purpose of this study is to evaluate feature selection methods to improve the interpretability and transparency of machine learning models in predicting the degree of difficulty of physics pretreatment plan reviews for H&N RT plans. We demonstrate a machine learning approach to classify H&N plans as easy, moderate, and difficult with reference to QA time involved and cognitive workload.Materials/Methods
Pre-treatment data were collected for 212 cases encompassing H&N cancer sites that were planned between July 2018 and October 2020. The outcome variable, a degree of difficulty on a scale of 1-10 was collected as a subjective rating by physicists who performed the pre-treatment chart check. Scores of 1-3 were labeled as easy, 4-6 as intermediate, and 7-10 as difficult. A clinician and physicist then identified potential features, based on clinical relevance (3 features), contribution to plan complexity (9 features), and QA metrics (4 features). 169 H&N RT plans were used as a training set and the remaining 43 were set aside as a test set, an 80/20 split. Synthetic Minority Oversampling Technique (SMOTE) with a minority sampling strategy was used to oversample the difficult class and improve performance (i.e., accuracy). Mutual information and chi-squared were compared for random forest, decision tree, and support vector machine (SVM). Features with a mutual information score > 0.05 and p value < 0.05 were included in the analysis.Results
On the test set, all three classifiers had the highest performance with SMOTE oversampling using chi-squared. Organ count, number of images, pregnancy (yes/no), author (physicist on plan) (p value < 0.01) and number of fractions (p value < 0.05) were identified as key features in predicting the degree of difficulty of H&N RT plans.Conclusion
We demonstrate that feature selection methods improve the transparency and interpretability as well as performance of ML algorithms. Identifying features that are most important in predicting the degree of difficulty of H&N RT plans will improve physicists' adoption of these classifiers in a future clinical implementation.查看译文
关键词
radiation therapy,machine learning,interpretability,neck
AI 理解论文
溯源树
样例
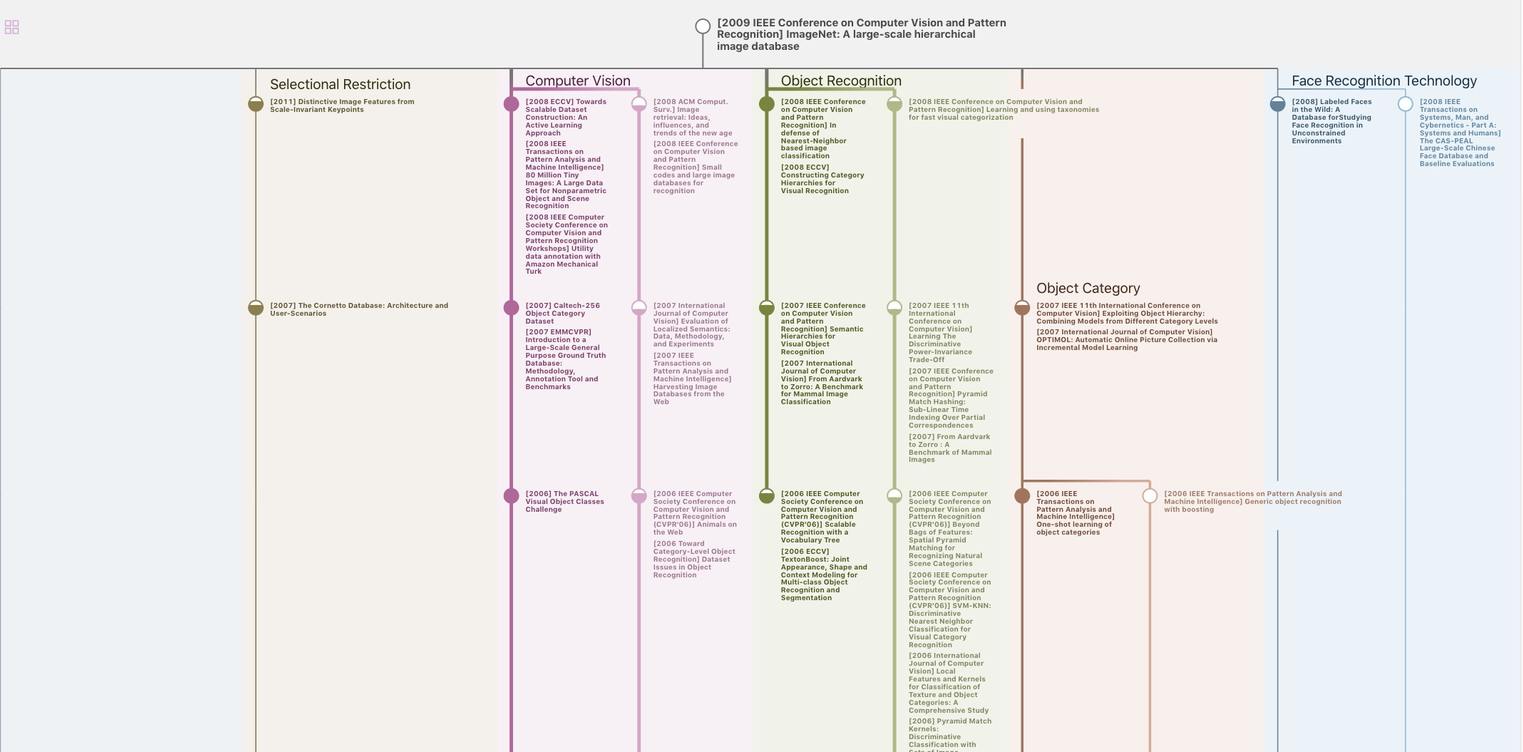
生成溯源树,研究论文发展脉络
Chat Paper
正在生成论文摘要