The effect of spatial granularity on optimal renewable energy portfolios in an integrated climate-energy assessment model
Sustainable Energy Technologies and Assessments(2022)
摘要
Renewable energy planning is key to achieving target levels of renewable-energy penetration and electricity demand in the European Union. Mean–variance analysis can be used to identify the optimal spatial and technological deployment of variable renewable energy (VRE) sources in terms of maximizing VRE penetration and minimizing supply risks. We investigate the extent to which optimizing capacities at the scale of climate-data grid points, instead of administrative regions (a common approach due to data availability and computation costs), helps generate more optimal renewable deployment scenarios. A finer description of climate resources, and thus the VRE capacity factors, results in a better exploitation of complementarities, partly due to the increased degrees of freedom in the optimization. A detailed analysis of the causes behind these improvements shows that better describing local conditions leads to two advantages over less granular counterparts: higher average capacity factors and generation combinations that offer lower covariances. This analysis also reveals that more granular approaches significantly reduce variability in daily and annual climate frequencies in renewable generation under the optimal scenario. These results provide evidence of the need to account for detailed climate information to accurately identify optimal renewable deployment scenarios and support stakeholders and policy makers when it comes to making sustainable commitments.
更多查看译文
关键词
46N99
AI 理解论文
溯源树
样例
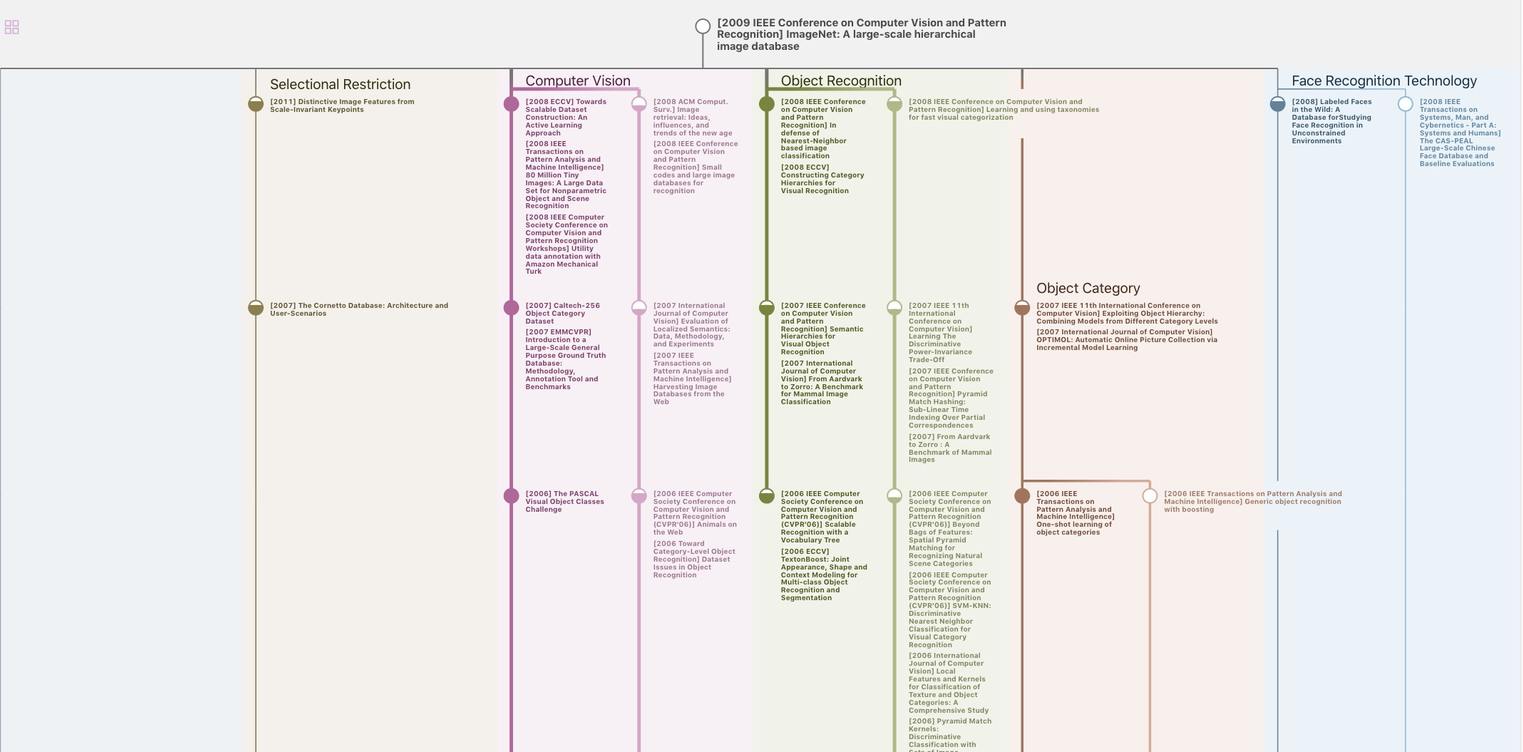
生成溯源树,研究论文发展脉络
Chat Paper
正在生成论文摘要