Pathway-informed deep learning model for survival analysis and pathological classification of gliomas
biorxiv(2022)
摘要
Online assessment of the tumor pathology during surgery is an important task to give feedback to the surgeon who can be more liberal or conservative in the resection based on the input. While there are methods that perform metabolomics-based online tumor grade prediction, their performance and model complexities are limited by the small dataset sizes. Here, we propose a pathway-informed deep learning model, PiDeel, to perform survival analysis simultaneously for a better prognostic assessment. We show that incorporating the pathway information into the model architecture and using the multitask learning framework reduce the model complexity and enable us to use deeper architectures with better performance. We show that PiDeel improves the grade prediction performance of the state-of-the-art in terms of the Area Under the ROC Curve (AUC-ROC) by 3.38% and the Area Under the Precision-Recall Curve (AUC-PR) by 4.06% and survival analysis performance based on the time-dependent concordance index (c-index) by 2.06%. Analyzing the importance of input metabolites and neurons representing pathways provides insights into tumor metabolism. We foresee that using this model in the surgery room will help surgeons adjust the surgery plan on the fly and will result in improved prognosis due to optimized surgical procedures. The code is released at https://github.com/ciceklab/PiDeeL. The data used in this study is released at https://zenodo.org/record/7228791.
### Competing Interest Statement
The authors have declared no competing interest.
更多查看译文
AI 理解论文
溯源树
样例
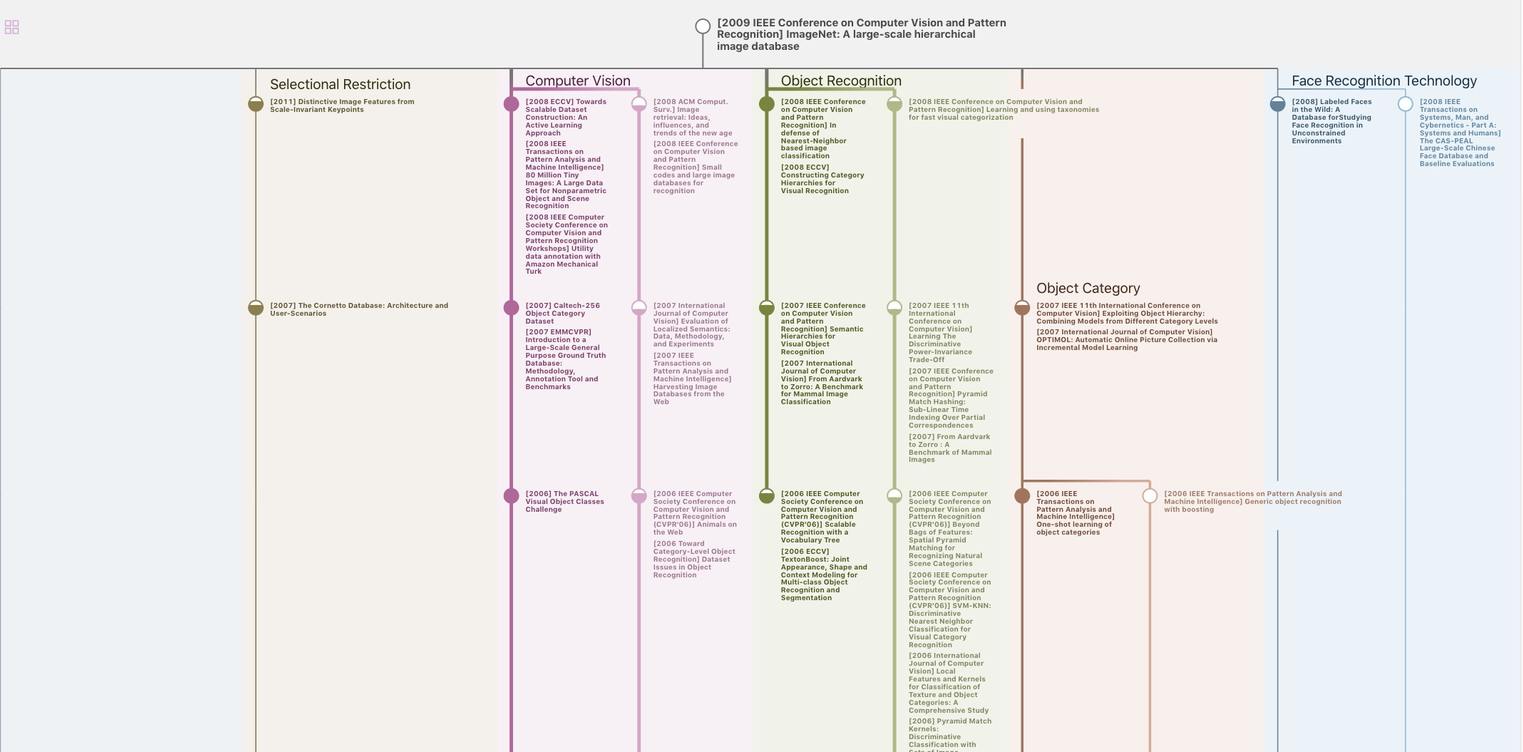
生成溯源树,研究论文发展脉络
Chat Paper
正在生成论文摘要