A novel heterogeneous transfer learning method based on data stitching for the sequential coding brain computer interface
COMPUTERS IN BIOLOGY AND MEDICINE(2022)
摘要
Objective: For the brain computer interface (BCI), it is necessary to collect enough electroencephalography (EEG) signals to train the classification model. When the operation dimension of BCI is large, it will bring great burden to data acquisition. Fortunately, this problem can be solved by our proposed transfer learning method.Method: For the sequential coding experimental paradigm, the multi-band data stitching with label alignment and tangent space mapping (MDSLATSM) algorithm is proposed as a novel heterogeneous transfer learning method. After filtering by multi-band filtering, the artificial signals can be obtained by data stitching from the source domain, which build a bridge between the source domain and target domain. To make the distribution of two domains closer, their covariance matrices are aligned by label alignment. After mapping to the tangent space, the features are extracted from the Riemannian manifold. Finally, the classification results are obtained with feature selection and classification.Results: Our data set includes the EEG signals from 16 subjects. For the heterogeneous transfer learning of cross -label, the average classification accuracy is 78.28%. MDSLATSM is also tested for cross-subject, and the average classification accuracy is 64.01%, which is better than existing methods.Significance: Combining multi-band filtering, data stitching, label alignment and tangent space mapping, a novel heterogeneous transfer learning method can be achieved with superior performance, which promotes the practical application of the BCI systems.
更多查看译文
关键词
Brain-computer interfaces (BCI),Transfer learning,Label alignment,Tangent space mapping,Multi-band filtering
AI 理解论文
溯源树
样例
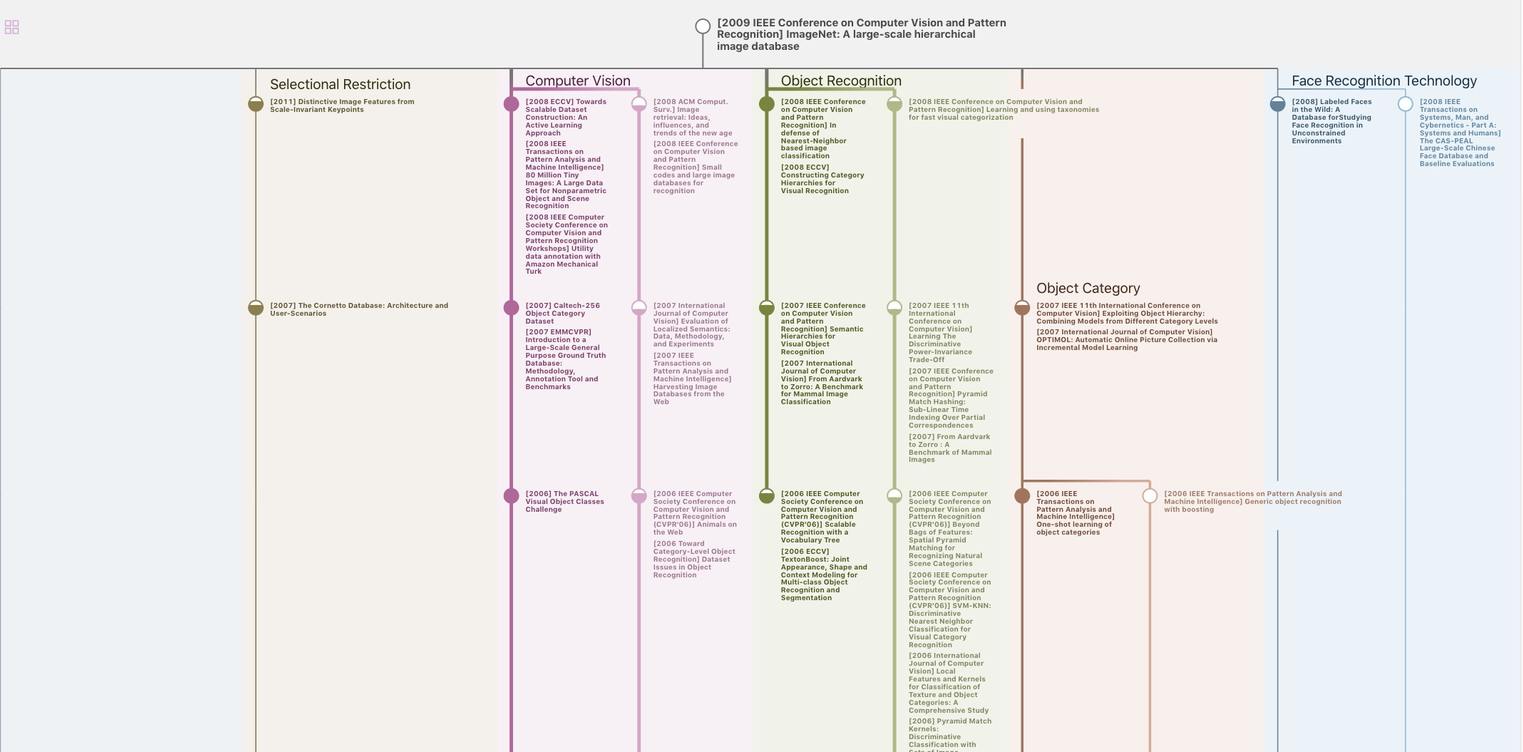
生成溯源树,研究论文发展脉络
Chat Paper
正在生成论文摘要