Orthogonal autoencoder regression for image classification
Information Sciences(2022)
摘要
Least squares regression (LSR) and its extended methods are widely used for image classification. However, these LSR-based methods do not consider the importance of global information and ignore the connection between feature learning and regression representations. To address these problems, we propose a novel method called orthogonal autoencoder regression (OAR) that considers global information by combining the feature learning part with the regression representation part. In addition, to enhance the model’s feature learning ability, we introduce an orthogonal autoencoder model to learn more effective data. To promote the model’s regression representation ability, we also add weight constraints to the model and make the OAR more discriminative. An iterative algorithm with the alternating direction method of multipliers (ADMM) is proposed to solve the model. The experimental results from several databases demonstrate the effectiveness of the OAR.
更多查看译文
关键词
Orthogonal autoencoder,Feature learning,Denoising,Image classification
AI 理解论文
溯源树
样例
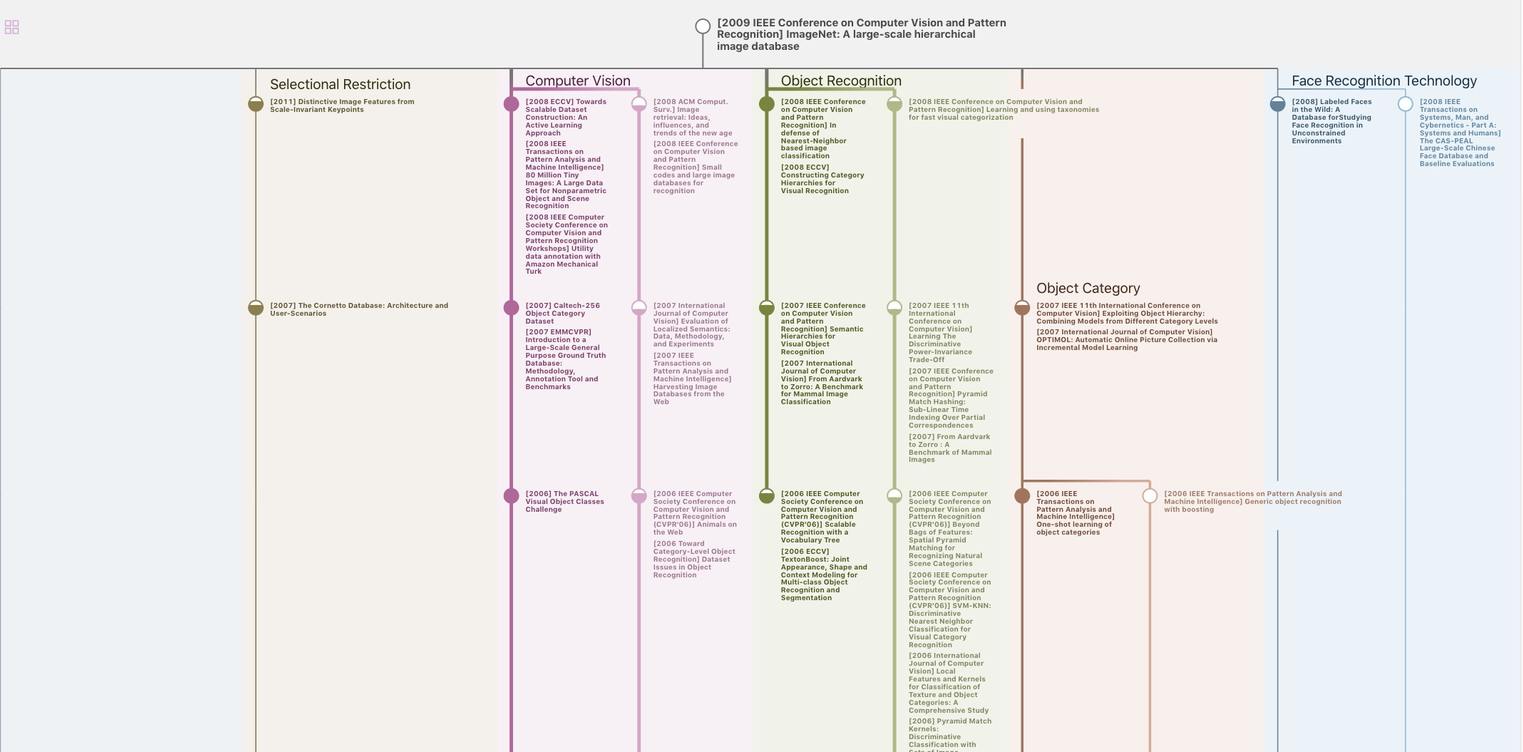
生成溯源树,研究论文发展脉络
Chat Paper
正在生成论文摘要