Singular spectrum analysis and circulant maximum variance frames
ADVANCES IN DATA SCIENCE AND ADAPTIVE ANALYSIS(2022)
摘要
Singular spectrum analysis (SSA) is a powerful method that is frequently used in dynamical systems theory and time series analysis. However, the algorithm itself is only partially understood. In this paper, we tackle the problem of a thorough interpretation of the complete basic SSA algorithm. We point out the relationship between SSA and Fourier analysis (FA), linear filtering (LF), principal component analysis (PCA), and power spectrum density estimation (PSDE). The main contribution is a reformulation and comprehensive interpretation of SSA by means of a novel class of data-adaptive circulant frames. Based on this class of frames, we introduce a formalism for time series decomposition and reconstruction. This mathematical framework generalizes principle component analysis and SSA. We show that SSA, PCA, FA, LF, and PSDE can be found from special cases of the proposed frame structures. This way we are able to provide a formal description of SSA and its relationship to the aforementioned methods. Whilst previous approaches to the interpretation of SSA relied on approximations, we provide exact mathematical descriptions of the relation between the above-mentioned techniques.
更多查看译文
关键词
SSA, PCA, discrete Fourier transform, linear filtering, spectral density estimation, frames, Circulant matrix, Toeplitz matrix, IIankel matrix
AI 理解论文
溯源树
样例
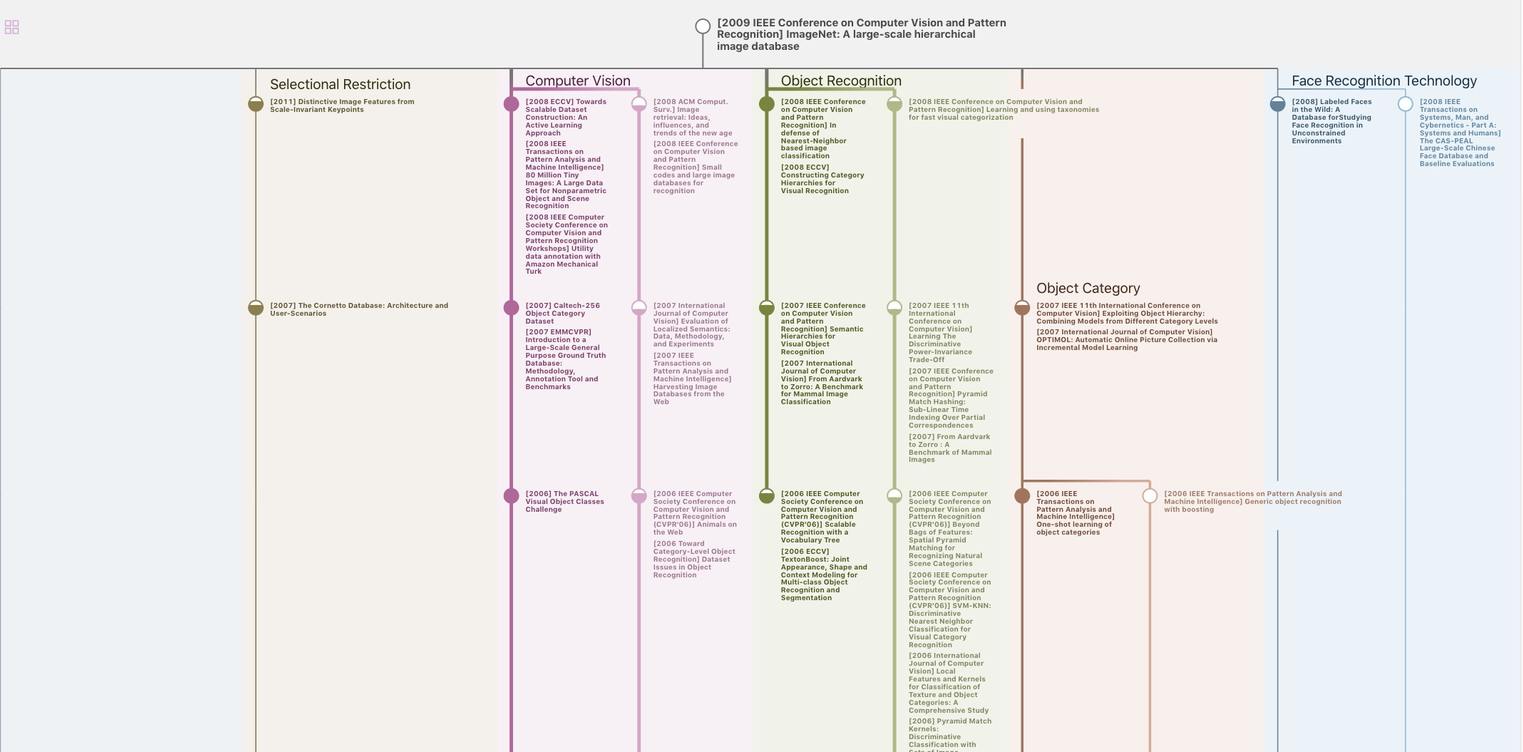
生成溯源树,研究论文发展脉络
Chat Paper
正在生成论文摘要