Assessment of training strategies for convolutional neural network to restore low-dose digital breast tomosynthesis projections
MEDICAL IMAGING 2022: PHYSICS OF MEDICAL IMAGING(2022)
摘要
Convolutional neural networks (CNNs) have been used for image processing tasks such as denoising, deraining, super-resolution and deblurring. It is known that deep models require a large amount of data for the training process. In medical X-ray imaging, data availability is a concern due to patient privacy and radiation exposure. To optimize the training process, here we investigate the effects of training strategies, such as the dataset size, early stop and cross-validation, on the performance of deep neural network. We used the residual network (ResNet) architecture, dedicated to restore low-dose (LD) digital breast tomosynthesis (DBT) raw projections. For this assessment, we generated 500 synthetic breast phantoms through virtual clinical trials software (Open-VCT) and validated them in terms of noise and signal properties. We acquired real raw DBT projections on a commercially available DBT system using a physical anthropomorphic breast phantom and restored the LD projections after training the CNN. Our goal is to restore the LD projections to achieve the same characteristics as the full-dose acquisitions. We found that early stop can be applied in the training process depending on the denoising strength desired for the network. Also, different training realizations are necessary to achieve good results. Furthermore, the training sample size may be smaller compared to other computer vision tasks using deep learning algorithms. Clearly, the amount of data is not the only factor in medical image restoration and other aspects such as network architecture, loss function and data variability must be investigated. The source code developed in this study is available at www.github.com/LAVI-USP/SPIE2022.
更多查看译文
关键词
Digital breast tomosynthesis, convolutional neural network, deep learning, virtual clinical trials, image denoising, image restoration
AI 理解论文
溯源树
样例
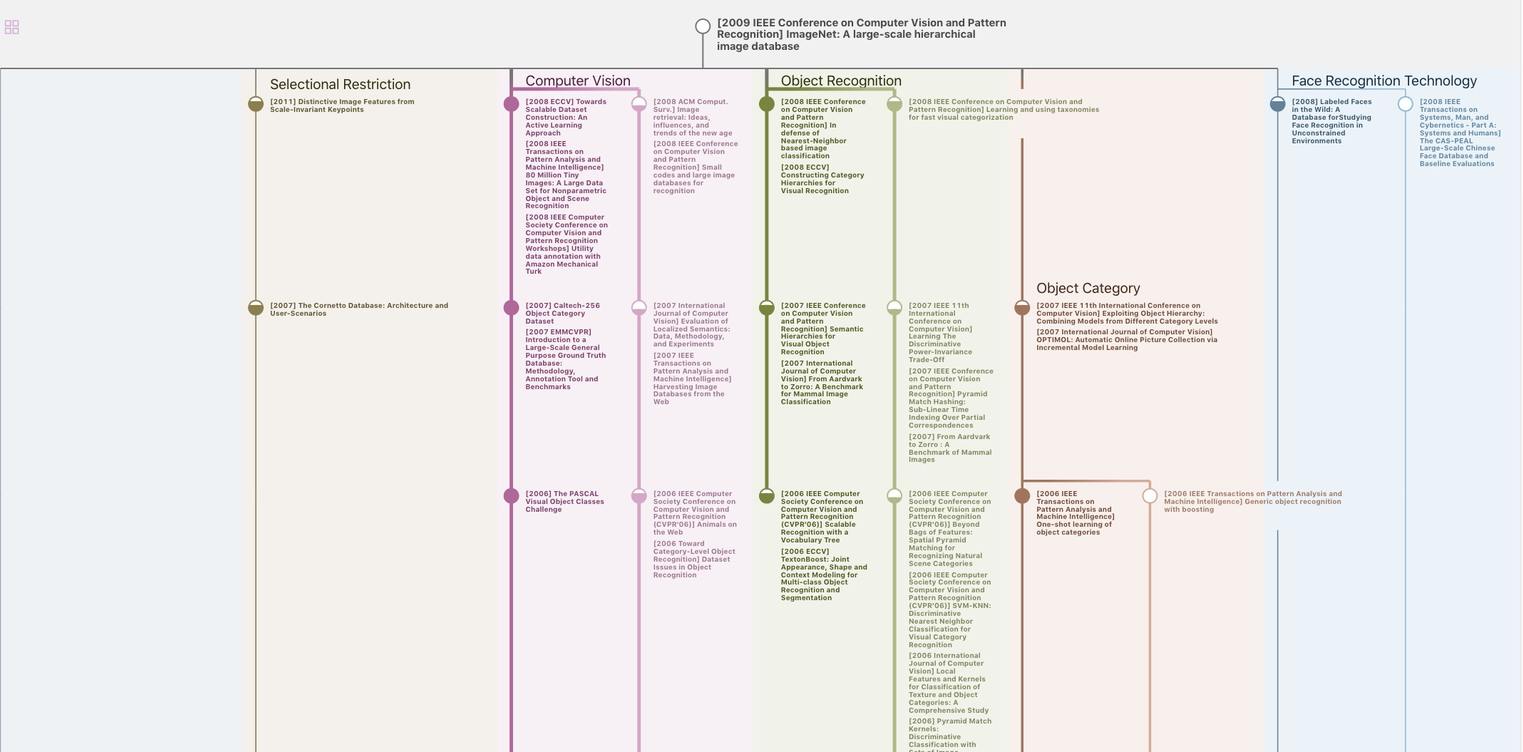
生成溯源树,研究论文发展脉络
Chat Paper
正在生成论文摘要