High-accuracy quantitative analysis of coal by small sample modelling algorithm based laser induced breakdown spectroscopy
JOURNAL OF ANALYTICAL ATOMIC SPECTROMETRY(2022)
摘要
In general, high-accuracy quantitative analysis of material components based on laser induced breakdown spectra requires large sample sizes for accurate modeling. However, in most cases, there are no abundant standard samples. Herein, small sample size (less than 20 standard samples) machine learning based on LIBS is utilized to realize high-accuracy quantitative analysis of coal. The algorithm is implemented through specific data extraction and data resampling. Partial spectra of each sample are extracted to form a batch of training data for establishing a prediction model based on the principal component analysis combined with partial least squares method (PCA-PLS). Repeatedly stochastic data are extracted from the spectra of the samples to form abundant batches of training data sets, and thus the most representative dataset is discovered in the training processes. The model trained by the new algorithm via 10-15 samples has a comparable performance to the model trained by the traditional method via 60-80 samples. With a training sample size of 15, the ARE of carbon prediction is less than 4% in the new prediction model, indicating that the proposed model has better generalization and higher prediction accuracy for small-sample-size machine learning. It will greatly improve the universality of the LIBS technology for fast elemental detection.
更多查看译文
关键词
coal,laser,high-accuracy
AI 理解论文
溯源树
样例
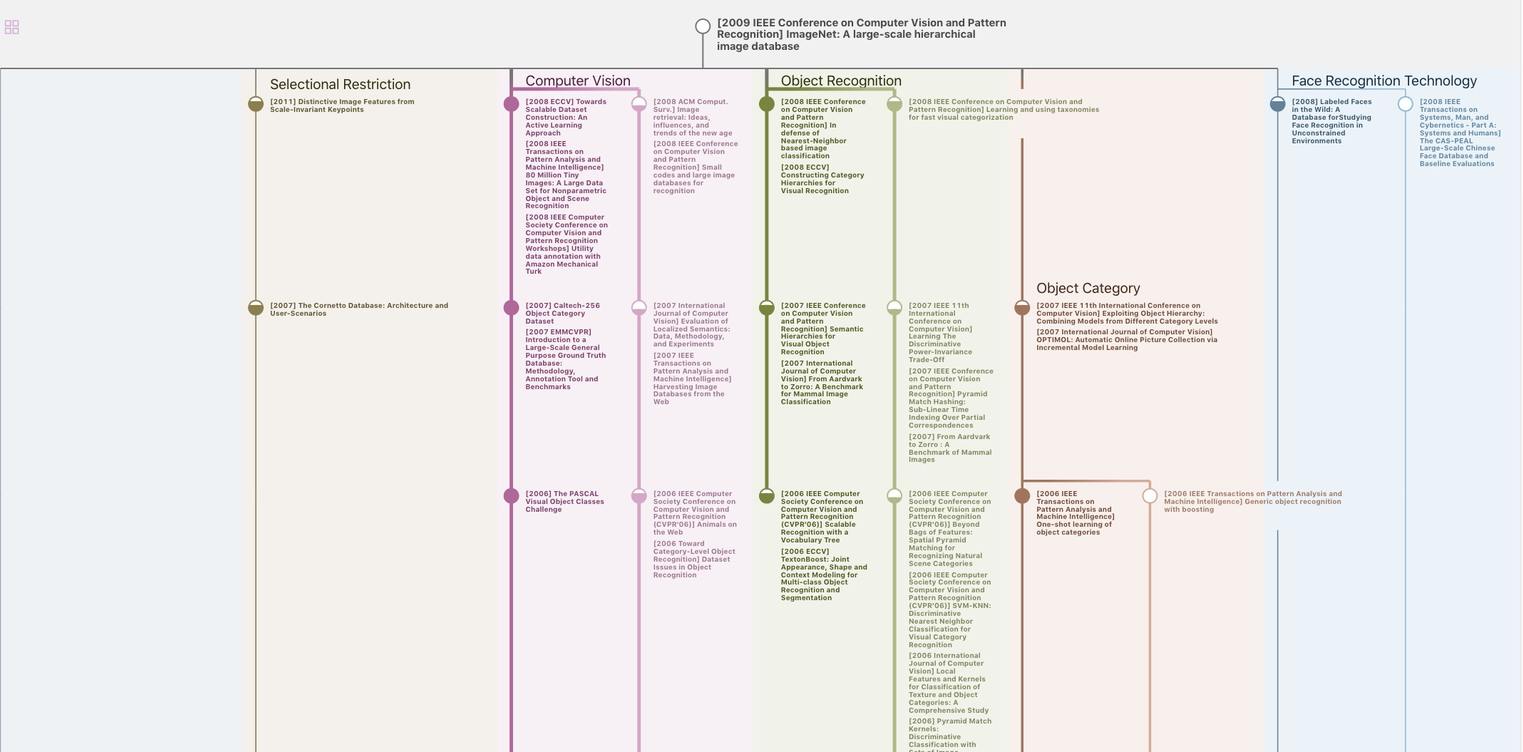
生成溯源树,研究论文发展脉络
Chat Paper
正在生成论文摘要