Clustering and Classification Models For Student's Grit Detection in E-Learning
2022 IEEE World AI IoT Congress (AIIoT)(2022)
摘要
Grit plays a crucial role in determining high individual success more than intellectual talent alone. However, there is no existing literature that ventured into the trait identification in an e-learning environment. This study presents a comprehensive computational-driven strategy for detecting a learner's grit using machine learning. Empirical results show that DBSCAN and Random Forest models produce average accurate prediction consistency of 92.67% against the questionnaire method. Knowledge interpretation using feature importance and association mining quantifies perseverance and sustained interest as the most pressing component of grit. Correlational analysis reveals that grit has a weak connection with course grades (short-term goal) but demonstrates a strong positive association with professional achievement (long-term goal) and maturation. Collectively, our findings substantiate that breakthrough accomplishment is contingent not solely on cognitive ability but on constant interests and resilience.
更多查看译文
关键词
classification,density-based clustering,dynamic time warping,grit scale,non-cognitive traits,prediction
AI 理解论文
溯源树
样例
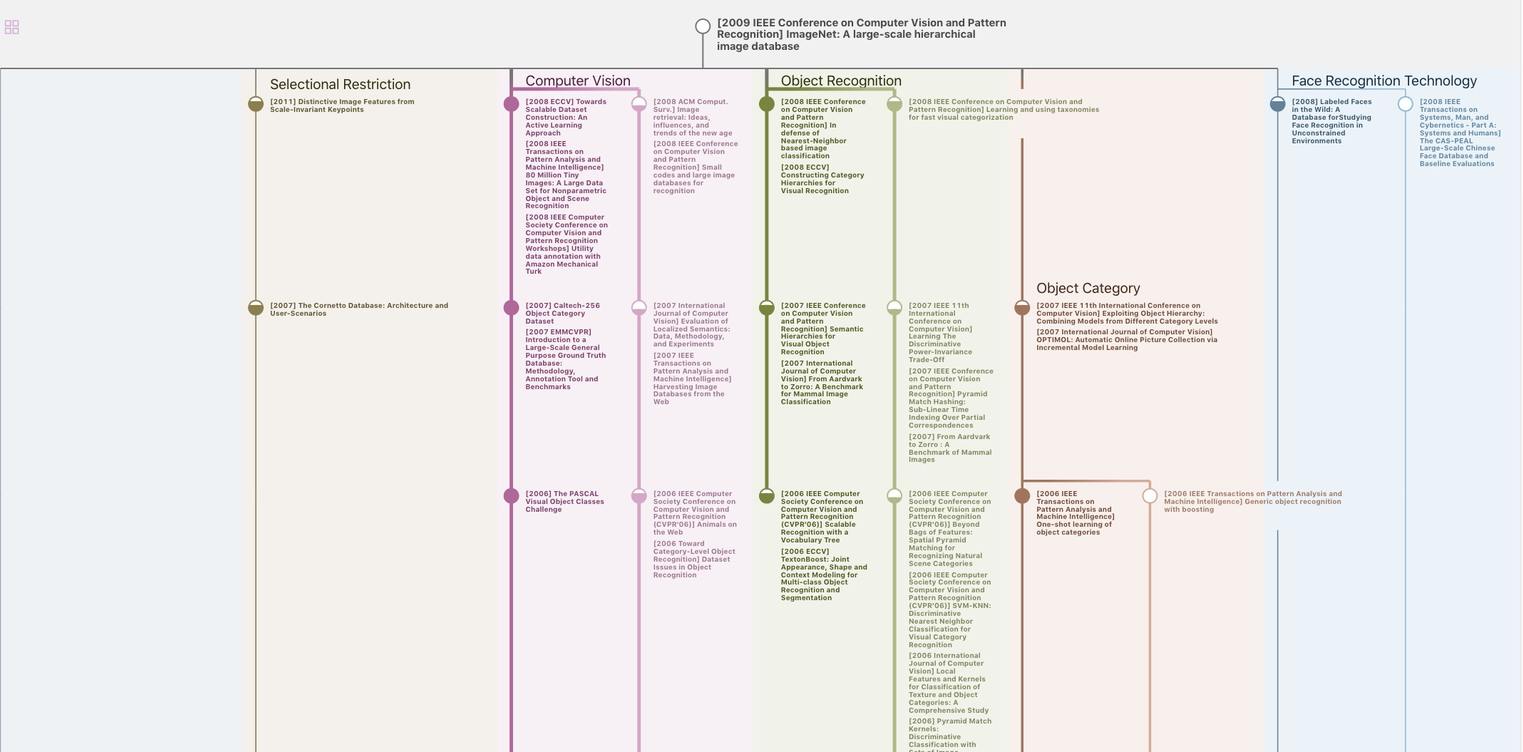
生成溯源树,研究论文发展脉络
Chat Paper
正在生成论文摘要