Antenna Array Beamforming Based on Deep Learning Neural Network Architectures
2022 3RD URSI ATLANTIC AND ASIA PACIFIC RADIO SCIENCE MEETING (AT-AP-RASC)(2022)
摘要
The implementation of antenna array beamforming using several neural network (NN) architectures is compared in this paper. Gated recurrent unit, feed-forward NN, convolutional NN, and long short-term memory architectures have been used for the beamforming process. This comparative study is carried out using various metrics, such as the root mean square error, and the computational time for each NN. In addition, the mean absolute divergences of the antenna array main lobe and nulls directions from their respective desired directions have also been used to assess the performance of each beamformer. The neural networks are trained using the simulation results of a 16-element microstrip patch antenna array. It is demonstrated that deep learning-based beamformers are capable of computing optimum antenna array weights in real time and in environments that change with time.
更多查看译文
关键词
mean absolute divergences,microstrip patch antenna array,deep learning-based beamformers,optimum antenna array weights,deep learning neural network architectures,gated recurrent unit,convolutional NN,short-term memory architectures,antenna array beamforming,feed-forward NN,long short-term memory architectures
AI 理解论文
溯源树
样例
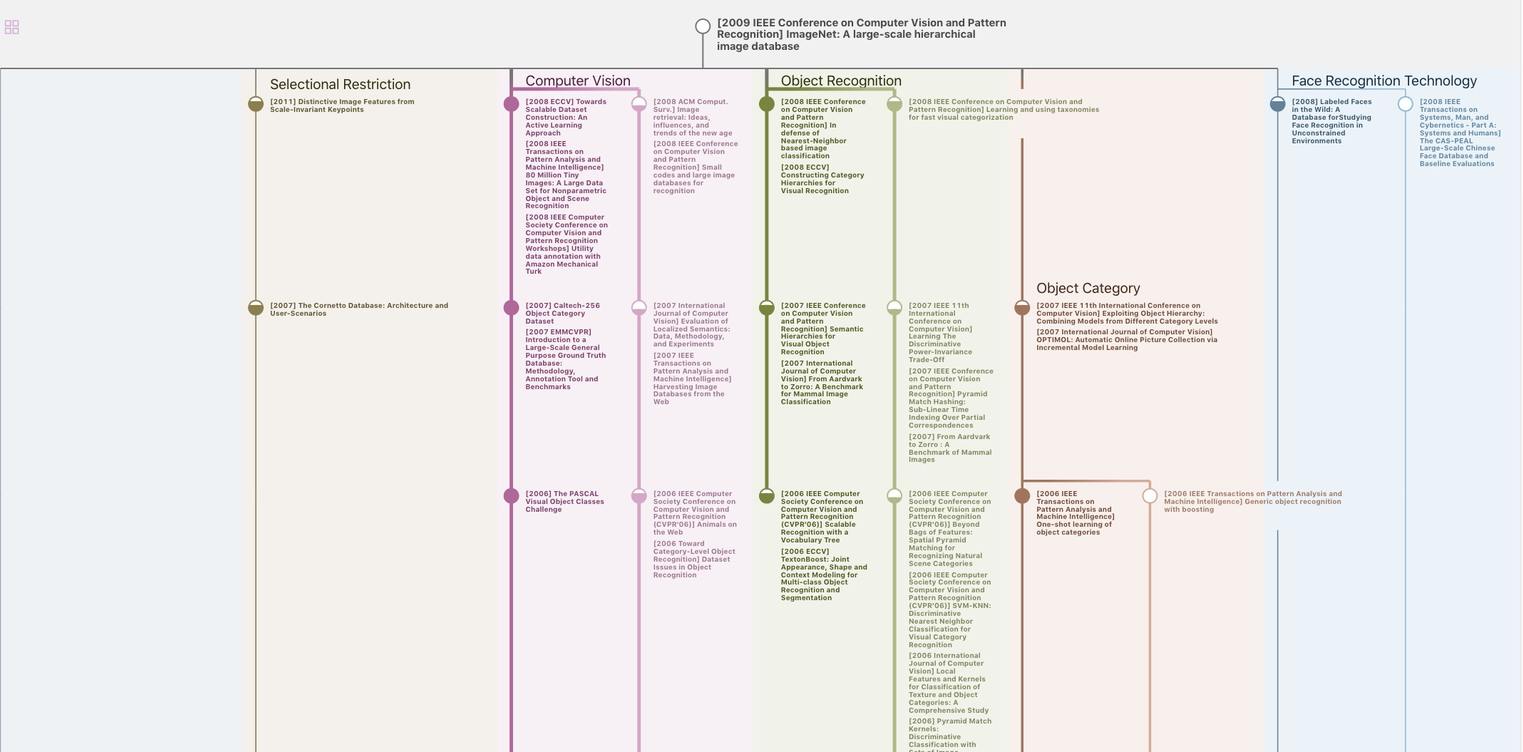
生成溯源树,研究论文发展脉络
Chat Paper
正在生成论文摘要