Adversarial Robustness in Graph-Based Neural Architecture Search for Edge AI Transportation Systems
IEEE TRANSACTIONS ON INTELLIGENT TRANSPORTATION SYSTEMS(2023)
摘要
Edge AI technologies have been used for many Intelligent Transportation Systems, such as road traffic monitor systems. Neural Architecture Search (NAS) is a typcial way to search high-performance models for edge devices with limited computing resources. However, NAS is also vulnerable to adversarial attacks. In this paper, A One-Shot NAS is employed to realize derivative models with different scales. In order to study the relation between adversarial robustness and model scales, a graph-based method is designed to select best sub models generated from One-Shot NAS. Besides, an evaluation method is proposed to assess robustness of deep learning models under various scales of models. Experimental results shows an interesting phenomenon about the correlations between network sizes and model robustness, reducing model parameters will increase model robustness under maximum adversarial attacks, while, increasing model paremters will increase model robustness under minimum adversarial attacks. The phenomenon is analyzed, that is able to help understand the adversarial robustness of models with different scales for edge AI transportation systems.
更多查看译文
关键词
Robustness, Computational modeling, Data models, Mathematical models, Analytical models, Deep learning, Computer architecture, Adversarial robustness, adversarial example, model compression and neural architecture search
AI 理解论文
溯源树
样例
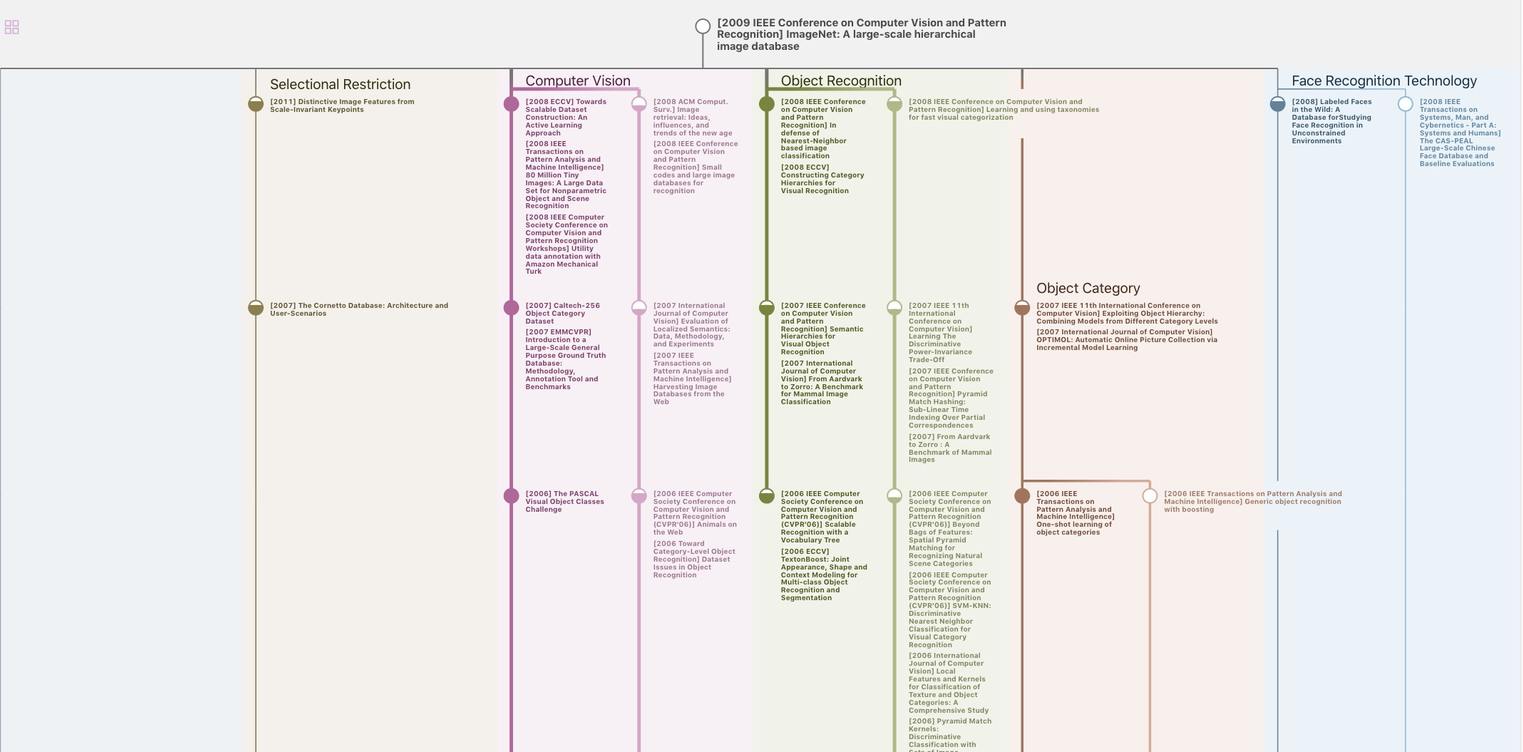
生成溯源树,研究论文发展脉络
Chat Paper
正在生成论文摘要