Traffic Anomaly Detection and Video Summarization Using Spatio-Temporal Rough Fuzzy Granulation With Z-Numbers
IEEE TRANSACTIONS ON INTELLIGENT TRANSPORTATION SYSTEMS(2022)
摘要
Existing traffic video summarization algorithms are capable of detecting one-class (i.e., collision) anomaly and cannot handle uncertainty issues arising between two-class anomalies, such as collision and near-miss. To address the issues, a new video summarization algorithm, namely Z-number s-based spatio-temporal rough fuzzy granulation (Z-STRFG) is developed. In Z-STRFG, various spatio-temporal features are computed over the video frames and used for obtaining the approximate anomaly-prone regions in terms of granules. In these regions, uncertainty (i.e., fuzziness) may arise among three scenarios, namely collision, near-miss, and normal traffic. Therefore, two types of rough fuzzy granules (RFGs) along with their roughness scores are computed to distinguish the aforesaid three scenarios. For each RFG, Z-number is computed based on the membership value of its roughness score to ensure a higher degree of reliability in the detection of anomaly class. Aforesaid characteristics of Z-STRFG improve its speed and accuracy for traffic anomaly detection. The efficacy of Z-STRFG has been demonstrated over 130 real-time traffic videos containing collisions, near-misses, and normal traffics. The superiority of Z-STRFG over some state-of-the-art is also proved through extensive experiments.
更多查看译文
关键词
Traffic anomaly detection, video summarization, spatio-temporal features, rough fuzzy granules, Z-numbers
AI 理解论文
溯源树
样例
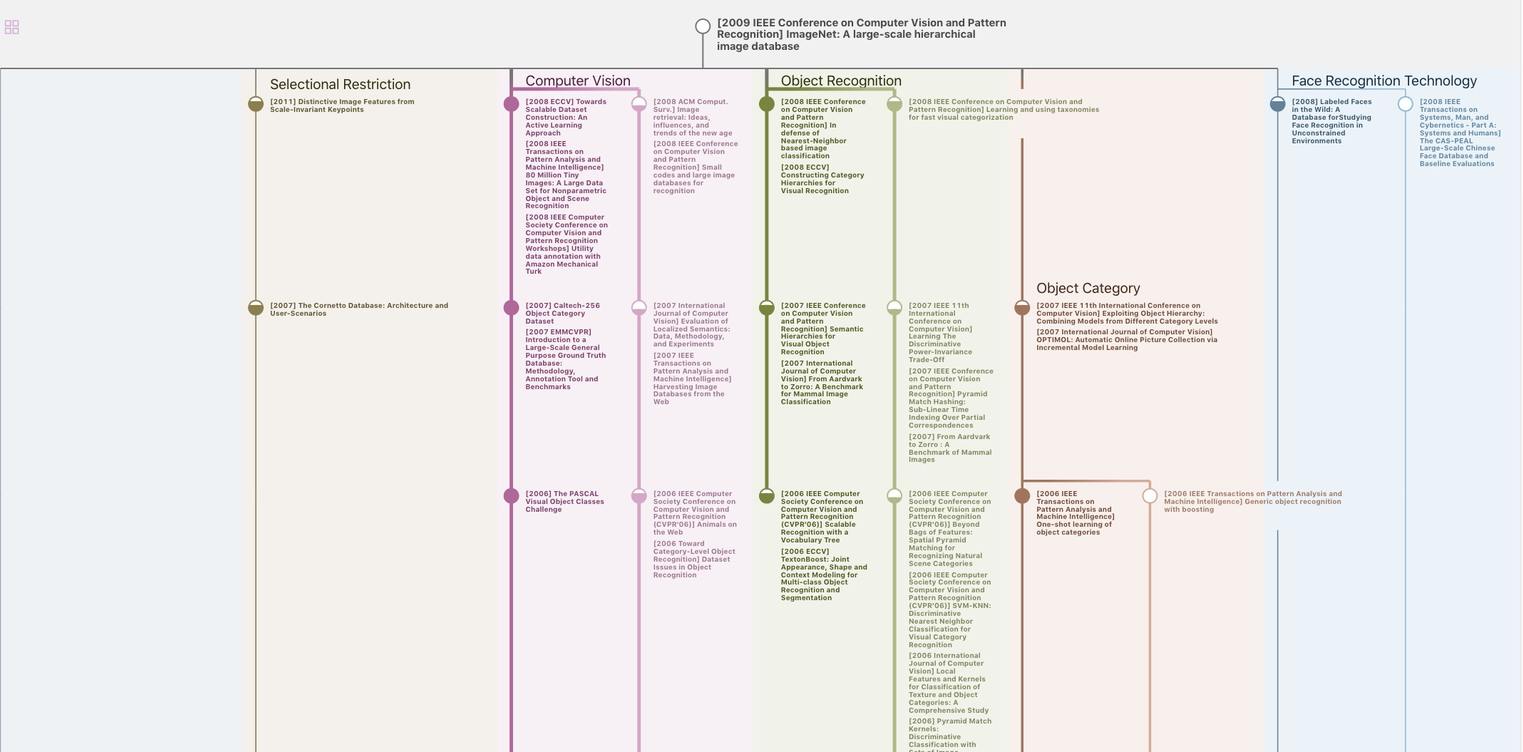
生成溯源树,研究论文发展脉络
Chat Paper
正在生成论文摘要