ENHANCING COLLABORATIVE FILTERING RECOMMENDATION USING REVIEW TEXT CLUSTERING
JORDANIAN JOURNAL OF COMPUTERS AND INFORMATION TECHNOLOGY(2021)
摘要
The enormous rapid growth of the online world and universal computing brought a wide range of choices for Internet users to obtain information of interest. However, the huge amount of new information released every day in "big data" is greater than the human information processing capacity. As a result, it becomes harder and harder for users to obtain the required information quickly and they are also facing the problem of information overload. Collaborative Filtering (CF) systems play an important role in overcoming the information overload phenomenon by providing users with relevant information based on their preferences. CF is one of the best recommendation approaches that automate the process of the "word-of-mouth" paradigm. The most critical tasks in CF are finding similar users with similar preferences and then predicting user ratings to provide a personalized list of ranked items to the users. Previous studies have almost exclusively focused on these tasks separately to enhance the quality of recommendation. Nevertheless, we argue that these two tasks are not completely independent, but are part of an incorporated process. The purpose of this study is to propose a recommendation method that bridge the gap between the tasks of rating prediction and ranking to better grasp the best similar users to the target user by combing the advantage potential information of users review text clustering and user numerical ratings to enhance the CF recommendation methods proposed in the literature. The experimental results on three different datasets from Amazon show a considerable improvement over the baseline CF approaches in terms of recall, precision and F1-measure.
更多查看译文
关键词
Recommender systems, Collaborative filtering, Review text, Clustering, Top-N recommendation
AI 理解论文
溯源树
样例
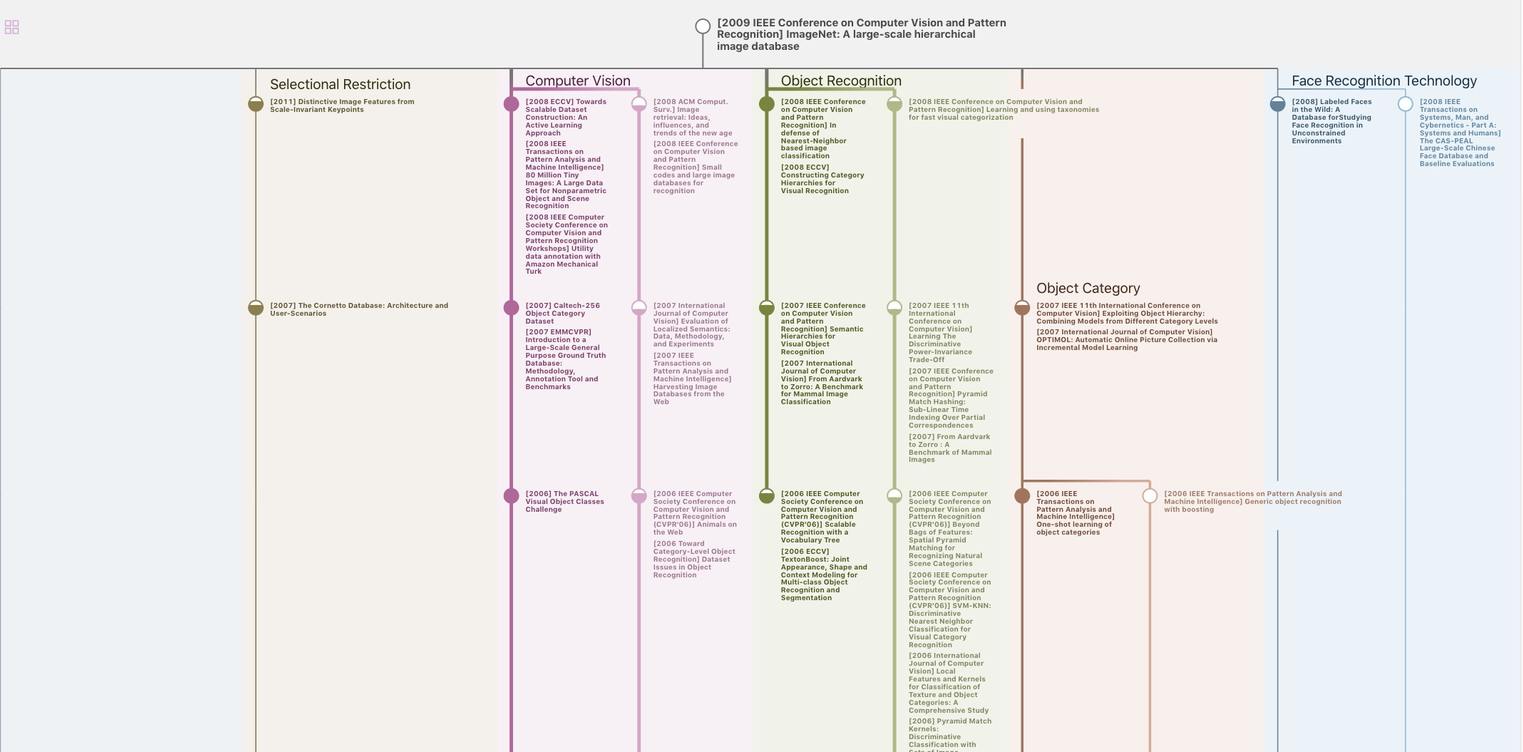
生成溯源树,研究论文发展脉络
Chat Paper
正在生成论文摘要