Minimum Sample Size Estimate for Classifying Invasive Lung Adenocarcinoma
APPLIED SCIENCES-BASEL(2022)
摘要
Statistical Learning Theory (SLT) plays an important role in prediction estimation and machine learning when only limited samples are available. At present, determining how many samples are necessary under given circumstances for prediction accuracy is still an unknown. In this paper, the medical diagnosis on lung cancer is taken as an example to solve the problem. Invasive adenocarcinoma (IA) is a main type of lung cancer, often presented as ground glass nodules (GGNs) in patient's CT images. Accurately discriminating IA from non-IA based on GGNs has important implications for taking the right approach to treatment and cure. Support Vector Machine (SVM) is an SLT application and is used to classify GGNs, wherein the interrelation between the generalization and the lower bound of necessary sampling numbers can be effectively recovered. In this research, to validate the interrelation, 436 GGNs were collected and labeled using surgical pathology. Then, a feature vector was constructed for each GGN sample through the fully connected layer of AlexNet. A 10-dimensional feature subset was then selected with the p-value calculated using Analysis of Variance (ANOVA). Finally, four sets with different sample sizes were used to construct an SVM classifier. Experiments show that a theoretical estimate of minimum sample size is consistent with actual values, and the lower bound on sample size can be solved under various generalization requirements.
更多查看译文
关键词
invasive adenocarcinoma, image classification, ground glass nodules, CT image
AI 理解论文
溯源树
样例
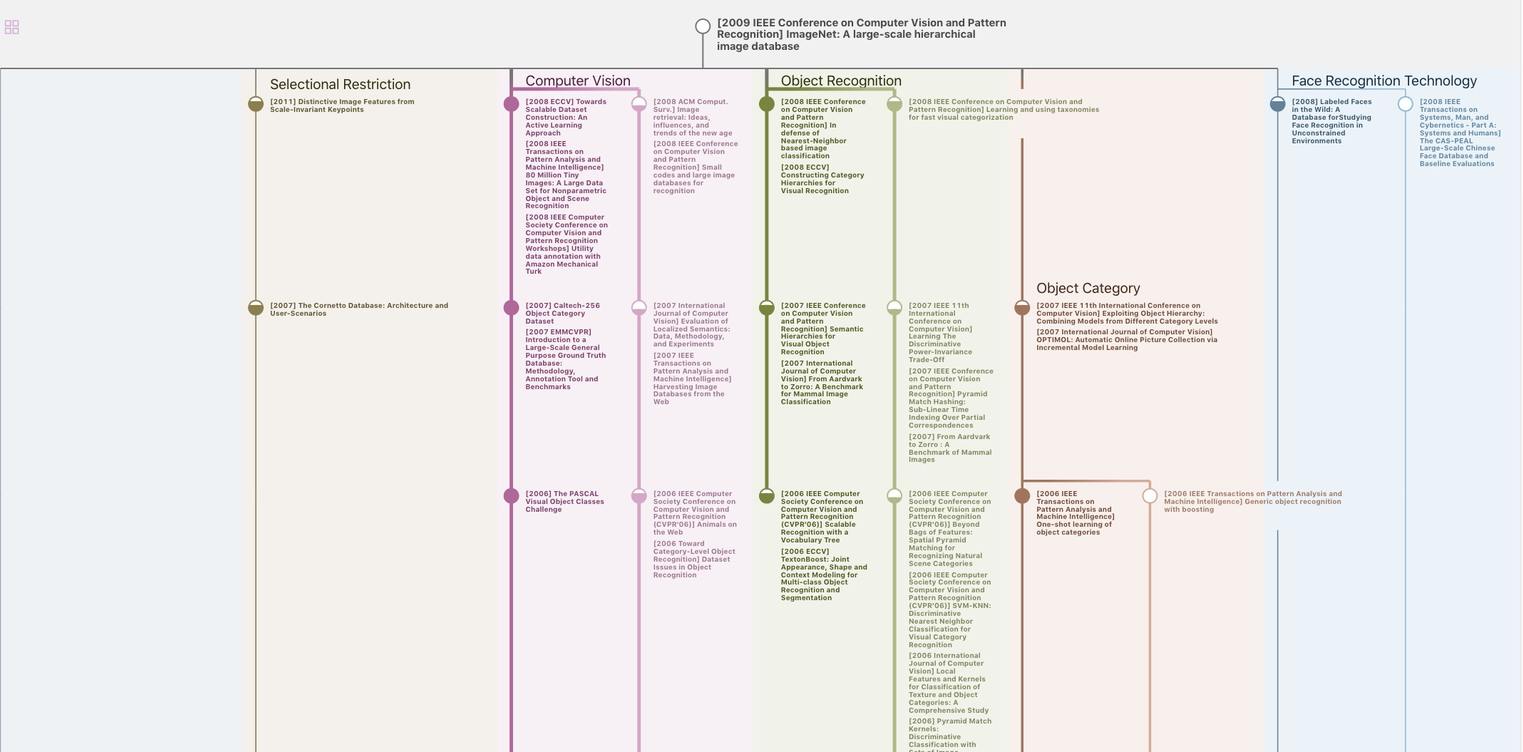
生成溯源树,研究论文发展脉络
Chat Paper
正在生成论文摘要