Learning to accelerate globally optimal solutions to the AC Optimal Power Flow problem
ELECTRIC POWER SYSTEMS RESEARCH(2022)
摘要
We propose machine learning-based (ML) methods to accelerate convergence to global solutions for the AC Optimal Power Flow (AC-OPF) problem. In particular, for the non-convex AC-OPF problem, optimality-based bound tightening (OBBT) has been observed to be a very effective approach for tightening the variable domains, thus leading to tight convex relaxations that are nearly global optimum solutions. However, by construction, OBBT is computationally expensive even on medium-scaled power networks. To address this issue, we propose a novel ML-based policy to replace the exhaustive algorithm of OBBT by choosing a subset of variables whose tightening of bounds can still contribute to the best improvement of the convex relaxation of the AC-OPF problem. To this end, we leverage historical data of load profiles for a test system to learn a map between the system loading and subset selection of variables which will need to participate in the OBBT algorithm, thus enabling us to find near-global optimal solutions at faster run-times. Finally, we present detailed numerical studies on a few medium-sized benchmark instances, on which we observe up to 6.3x speed-up in OBBT run times.
更多查看译文
关键词
Optimal power flow,Global optimization,Convex relaxation,Machine learning,Neural network
AI 理解论文
溯源树
样例
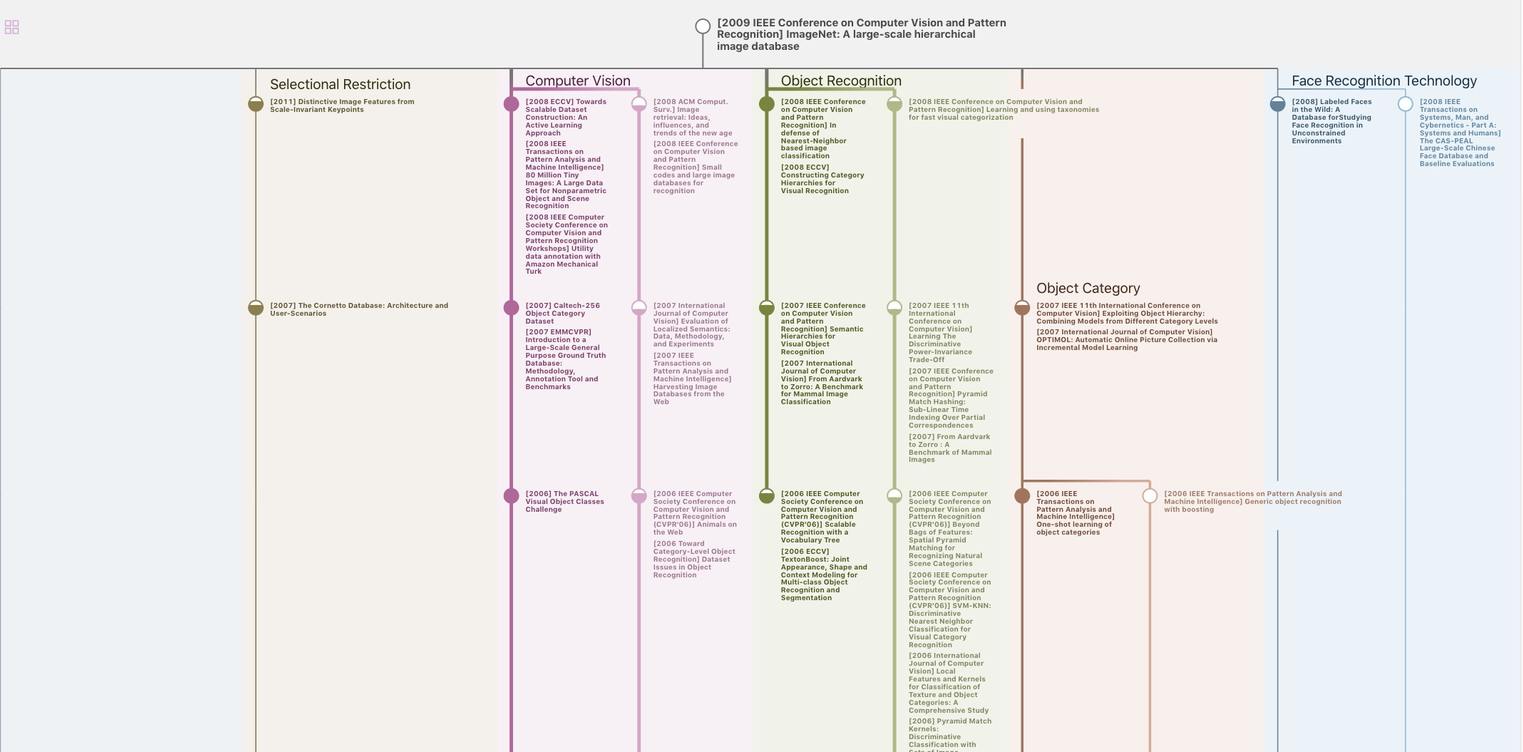
生成溯源树,研究论文发展脉络
Chat Paper
正在生成论文摘要