Overlapping Community Detection via Semi-Binary Matrix Factorization: Identifiability and Algorithms
IEEE TRANSACTIONS ON SIGNAL PROCESSING(2022)
摘要
Community detection is a fundamental problem in knowledge discovery and data mining. In this paper we propose a semi-binary matrix factorization (SBMF) model for community detection, which can be understood as a marriage between K-means clustering and (semi-)nonnegative matrix factorization. This leads to an easy-to-interpret factorization that can naturally handle overlapping communities. Unlike K-means, the proposed approach does not restrict each individual to belong to only a single community, nor does it restrict the sum of "soft membership" values to add up to one. We derive relatively easy-to-check uniqueness conditions suggesting that meaningful communities can be obtained via SBMF. Computing a (least-squares) optimal SBMF is a hard mixed integer nonconvex optimization problem. We bypass this challenge by converting the problem into a coupled matrix-tensor factorization form, which only involves continuous variables and can be tackled using tensor decomposition tools, and can also be used to initialize optimization based methods. We present experiments with real data to demonstrate the effectiveness of the proposed approach for community detection in coauthorship networks and in financial stock market data.
更多查看译文
关键词
K-means clustering, combinatorial optimization, community detection, coupled matrix-tensor factorization, identifiability, matrix factorization, nonnegative matrix factorization, unsupervised learning
AI 理解论文
溯源树
样例
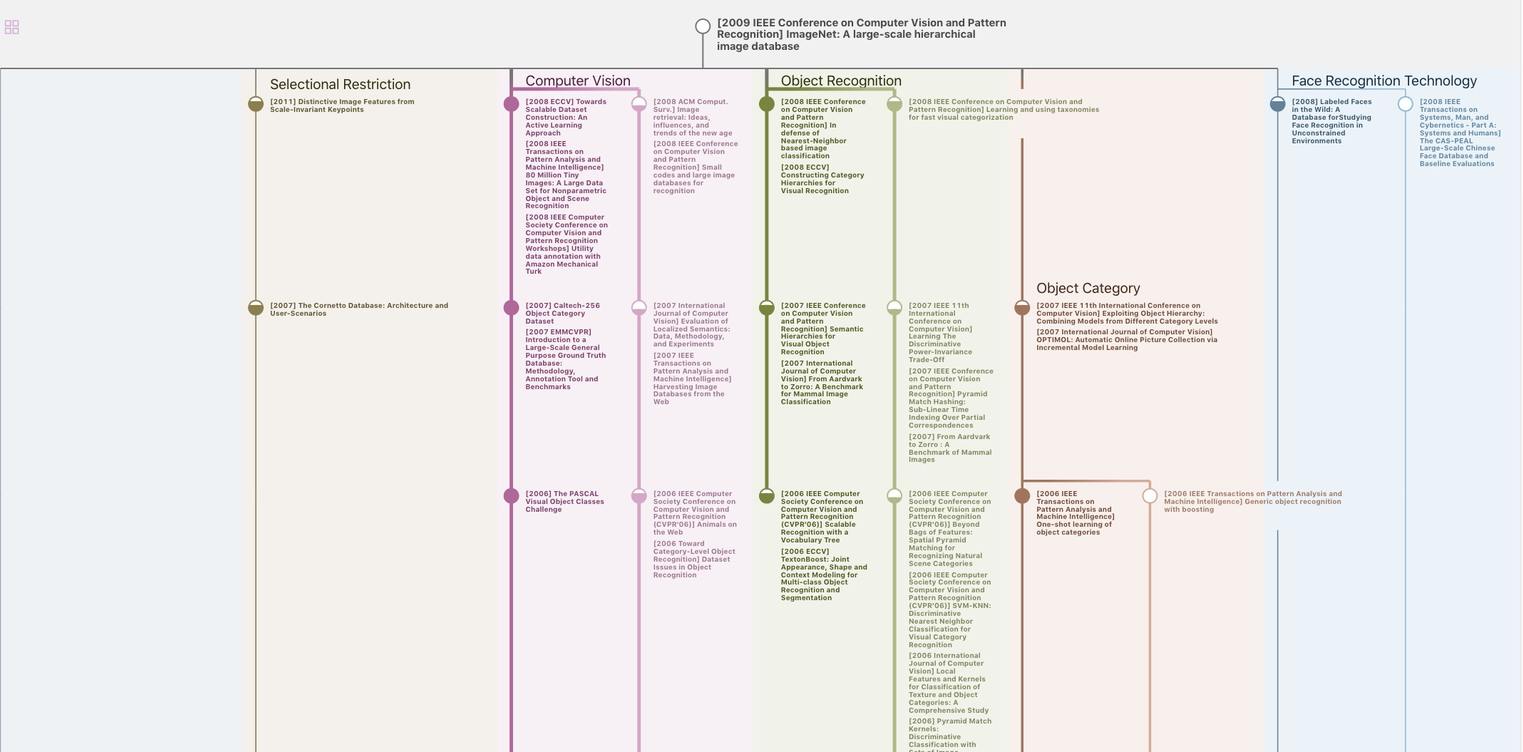
生成溯源树,研究论文发展脉络
Chat Paper
正在生成论文摘要