Cross Validation of TEMPEST-D and RainCube Observations Over Precipitation Systems
IEEE JOURNAL OF SELECTED TOPICS IN APPLIED EARTH OBSERVATIONS AND REMOTE SENSING(2022)
摘要
This article presents cross validation of nearly simultaneous observations between the Temporal Experiment for Storms and Tropical Systems
-
Demonstration
(
TEMPEST-D) and Radar in a CubeSat (RainCube) satellite microwave sensors over precipitation systems. RainCube senses the atmosphere using a
Ka
-band nadir-pointing radar, and TEMPEST-D senses using multifrequency millimeter-wave radiometers. Nine precipitation systems were used in this cross-validation study. Occurring over nearly a two-year period, these storms were scattered over many regions of the world from the Tropics to the midlatitudes. A shift correction algorithm was developed to remove the uncertainty between the two sensors’ observations due to the time difference and the storm motion between the two instrument overpasses. Correlation coefficients were calculated between self-normalized, inverted RainCube cumulative reflectivity and self-normalized TEMPEST-D brightness’ temperatures. As expected, these correlation coefficients are consistently higher for the four TEMPEST-D high-frequency channels than for the single low-frequency channel. The shift correction algorithm improved the average correlation coefficient by 19% for the four high-frequency channels and by 58% for the single low-frequency channel. The average correlation coefficient after shift correction is 0.76 for TEMPEST-Ds four high-frequency channels and 0.54 for the single low-frequency channel. The comparisons demonstrated high consistency between the TEMPEST-D and RainCube observations, even though the two microwave sensors’ fundamental physics is different; one is passive, and the other is active.
更多查看译文
关键词
CubeSat, Storms, Satellite broadcasting, Clouds, Meteorology, Atmospheric measurements, Sensors, CubeSats, radar in a CubeSat (RainCube), smallsats, temporal experiment for storms and tropical systems-demonstration (TEMPEST-D)
AI 理解论文
溯源树
样例
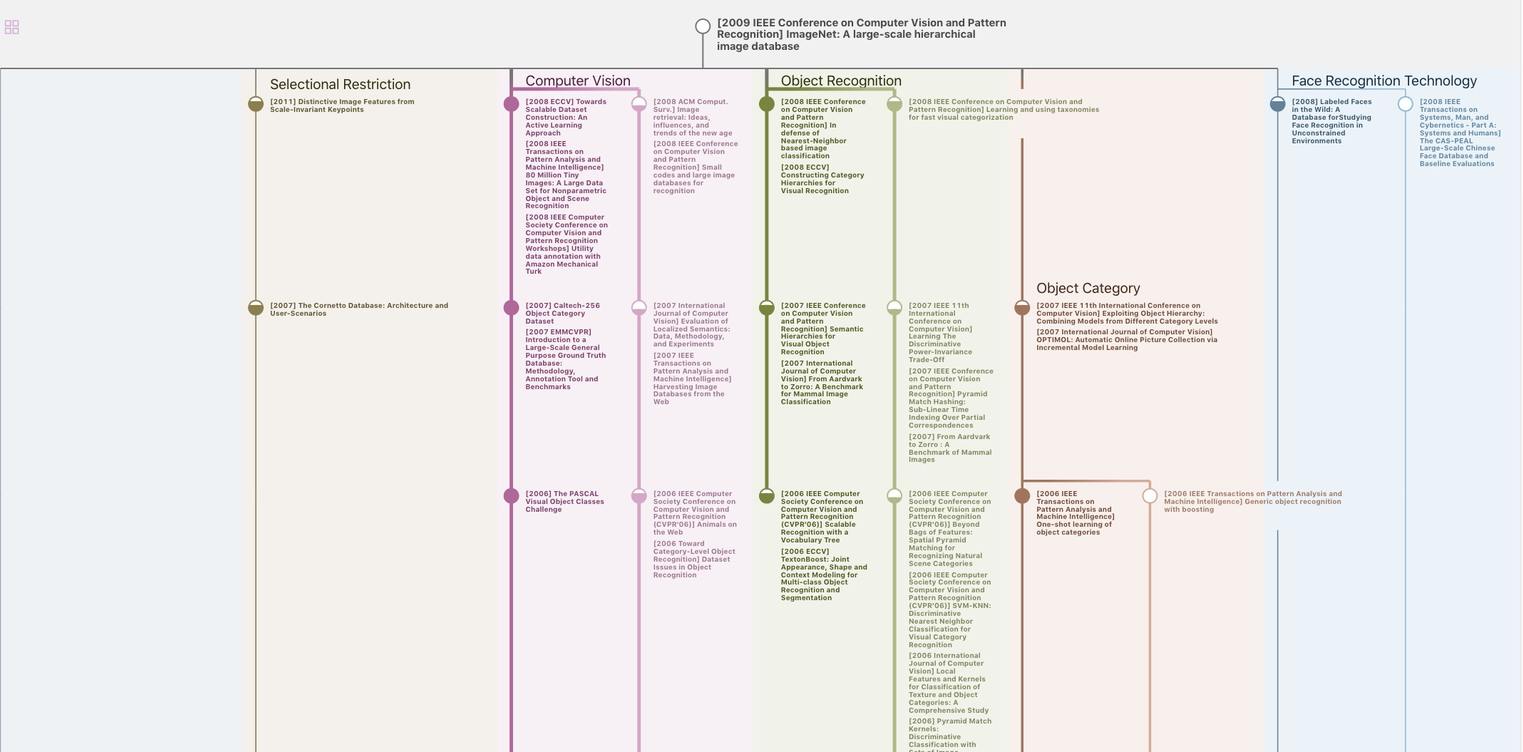
生成溯源树,研究论文发展脉络
Chat Paper
正在生成论文摘要