Elucidating the Chemical Compositions and Source Apportionment of Multi-Size Atmospheric Particulate (PM10, PM2.5 and PM1) in 2019-2020 Winter in Xinxiang, North China
ATMOSPHERE(2022)
摘要
The pollution characteristics of multi-size atmospheric particulates in Xinxiang, which was one of the most polluted cities across China, are still unclear even through air quality in Xinxiang has been improved in recent years. PM10, PM2.5, and PM1 samples were synchronously collected from 21 December 2019 to 17 January 2020 to explore pollution levels and reveal sources of PM in Xinxiang. The average mass concentrations of PM10, PM2.5, and PM1 were as high as 155.53 mu g m(-3), 120.07 mu g m(-3), and 85.64 mu g m(-3) during the observation period, respectively. Almost all of the chemical compositions in PM10, PM2.5 and PM1 increased continuously and obviously with the aggravation of the pollution level. Compared with the clean period, the enhancement of sulfate (23-27%) in PM was obvious higher than nitrate (19-22%) during the pollution period, which demonstrated that sulfate was the main contributor to the high concentration of PM in this study. Similar source distributions for PM10, PM2.5, and PM1 were also found, including traffic source, combustion source, secondary aerosols, industrial source, and fugitive dust, by using the positive matrix factorization (PMF) model. Furthermore, the contributions of the combustion source and secondary aerosol were found to be higher in smaller particles (PM2.5 and PM1), while the contribution of fugitive dust was higher in PM10. Moreover, dust and sand were entrained by air masses from the northwest that increased the contribution of dust in PM at the observation site. The potential source contribution function (PSCF) analysis illustrated that regional emission sources in northern and eastern Xinxiang might be important potential contributors to PM pollution in Xinxiang.
更多查看译文
关键词
multi-size airborne particles, chemical species, source apportionment, potential source contribution function, Xinxiang
AI 理解论文
溯源树
样例
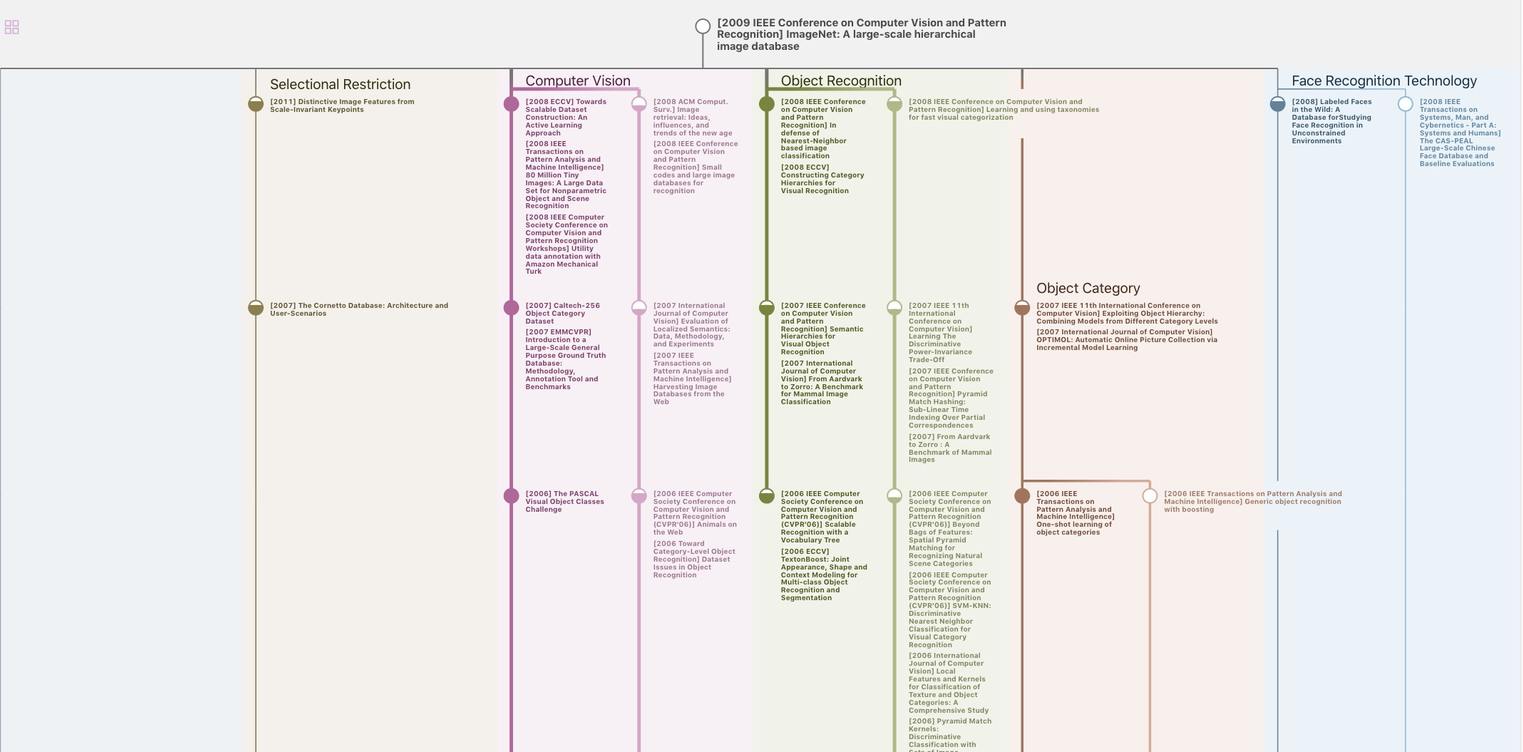
生成溯源树,研究论文发展脉络
Chat Paper
正在生成论文摘要